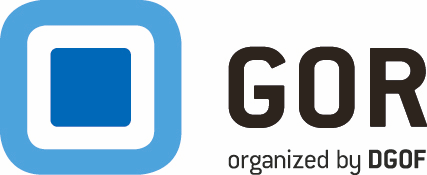
General Online Research Conference 2024 (GOR 24)
Rheinische Fachhochschule Cologne - Campus Vogelsanger Straße
21 - 23 February 2024
Conference Agenda
Overview and details of the sessions of this conference. Please select a date or location to show only sessions at that day or location. Please select a single session for detailed view (with abstracts and downloads if available).
|
Session Overview |
Date: Wednesday, 21/Feb/2024 | |
9:00am - 10:00am | Begin Check-in |
10:00am - 1:00pm | Workshop 1 Location: Seminar 2 (Room 1.02) Session Chair: Lisa de Vries, Bielefeld University, Germany Session Chair: Zaza Zindel, Bielefeld University, Germany |
|
Embracing Diversity: Integrating Queer Perspectives in Online Survey Research Bielefeld University, Germany Duration of the Workshop: Target Groups: Is the workshop geared at an exclusively German or an international audience? Workshop Language: Description of the content of the workshop: Political and social advancements have enhanced the acceptance and visibility of sexual and gender minorities in many Western countries. However, the ongoing challenge of accurately addressing their unique experience in online survey research remains. Researchers and sur-vey providers often struggle to incorporate queer perspectives, leaving many surveys and research designs blind to these minority groups. This workshop offers a comprehensive introduction to the integration of sexual and gender diversity within (online) survey research. It focuses on four key areas: 1) Measurement of Sexual Orientation and Gender Identity: Exploring nuanced approaches for respectful and inclusive data collection on sexual orientation and gender identity. 2) Integrating Queer Perspectives: Learning effective strategies to craft survey questions that resonate with and capture the experiences of sexual and gender minorities. 3) Sampling Methods: Gaining insights into strategies and techniques for effectively reaching and engaging sexual and gender minority populations in online survey re-search. 4) Data Preparation and Analysis: Equipping participants with the skills to sensitively manage and analyze data collected from diverse populations to draw valuable in-sights. This dynamic workshop combines informative presentations, group discussions, and hands-on exercises, ensuring participants leaving with the confidence and skills to successfully integrate sexual and gender diversity into their research projects.
Necessary prior knowledge of participants: Literature that participants need to read for preparation Recommended additional literature Information about the instructors: Lisa de Vries is a research associate in the Quantitative Methods of Empirical Social Research department at Bielefeld University. In her dissertation, she focused on the effects of discrimination on leadership positions and job preferences of sexual and gender minorities. Her research interests incluce discrimination and harassment, LGBTQI* parent families and the measurement of sexual orientation and gender identity (SOGI). In addition, she has several experiences with sampling LGBTQI*-people in probability and non-probability surveys as well as measuring SOGI. Zaza Zindel is a research associate and a doctoral candidate in sociology at Bielefeld University, specializing in survey research. Her dissertation centers around the use of social media as a means of recruiting rare populations for web surveys. Her research interests include survey methodology, the potential of social media for empirical social research, and exploring new technologies to improve statistical representation of marginalized, vulnerable, or rare populations. Will participants need to bring their own devices in order to be able to access the Internet? Will they need to bring anything else to the workshop? |
10:00am - 1:00pm | Workshop 2 Location: Seminar 4 (Room 1.11) Session Chair: Blanka Szeitl, HUN-REN, Hungary |
|
Probability theory in survey methods HUN-REN, Hungary Duration of the Workshop: Target Groups: Is the workshop geared at an exclusively German or an international audience? Workshop Language: Description of the content of the workshop: Goals of the workshop: Necessary prior knowledge of participants: Literature that participants need to read for preparation Recommended additional literature Information about the instructors: Blanka Szeitl is a survey methodologist and PhD candidate in applied mathematics. She is lecturer at Department of Statistics at University of Eötvös Lorand and at Bolyai Institute of Mathematics at University of Szeged. She is the head of Survey Methods Room Budapest research group focusing on innovative sampling procedures and data correction methods. She is researcher at HUN-REN Centre for Social Sciences, where she analyzes data of the European Social Survey (ESS). She is a member of the ESS Sampling and Weighting Expert Panel working on the implementation of the ESS sampling strategy for countries participating in the ESS data collection. She is co-founder of Panelstory Opinion Polls, which is the first mixed-method probability panel in Hungary. Her research interests are survey sampling, innovative methods, probability theory and assessment procedures. She loves to read about the history of probability and statistics. Will participants need to bring their own devices in order to be able to access the Internet? Will they need to bring anything else to the workshop? |
1:00pm - 1:30pm | Break |
1:30pm - 4:30pm | Workshop 3 - ONLINE! Location: Online (Virtual Room) Session Chair: Raffael Meier, onlineumfragen.com, Switzerland |
|
ONLINE WORKSHOP: The Magnificent Seven: Identify and Reduce Common Data Quality Issues in Online Surveys 1onlineumfragen.com GmbH, Switzerland; 2Pädagogische Hochschule Schwyz, Switzerland Duration of the Workshop: Target Groups: Is the workshop geared at an exclusively German or an international audience? Workshop Language: Description of the content of the workshop: In the workshop, we will look together at various possible contaminations, analyse data using concrete examples, discuss how such data can be prevented or cleaned up and what recommendations can be derived for future survey conducting. The workshop will include theoretical and practical inputs, as well as numerous activities with concrete data. Questions and discussions as well as examples from your own experience and data practice are welcome. Phenomena include speeders, outliers, acquiescence, extreme response styles, mid-point responding and stereotypical responses, patterns, misunderstanding of questions due to poor item construction, and falsified and partially falsified interviews by interviewers and staff of survey research organisations, different fieldwork standards, different question formats for the same topic across several surveys, different content-related or methodological contexts, e.g. in cross-national surveys or multi-year surveys, heterogeneous interpretation of the questions due to cultural differences. Necessary prior knowledge of participants: Literature that participants need to read for preparation Recommended additional literature Information about the instructors: Raffael is co-founder and CTO of onlineumfragen.com and works as a researcher for the professorship "Media and Computer Science Didactics" at the Institut für Medien und Schule (IMS) at University of Teacher Education Schwyz. With onlineumfragen.com, as a specialised online survey partner and survey consultant for the DACH region, he supports selected clients and manages the development of the survey platform. With an interdisciplinary background in psychology, educational science, computer science and methodology, he works entrepreneurially and scientifically on data quality in online surveys and privacy & data protection behaviour in children. He held several workshops on the topic of data quality in online surveys at the Lucerne University of Applied Sciences and Arts and for the Swiss market research association Swiss Insights. Will participants need to bring their own devices in order to be able to access the Internet? Will they need to bring anything else to the workshop? |
1:30pm - 4:30pm | Workshop 4 Location: Seminar 4 (Room 1.11) Session Chair: Ji-Ping Lin, Academia Sinica, Taiwan |
|
Why Data Science and Open Science Are Key to Build Smart Big Data: An Example Based on a Decade Research on Hard-to-Reach Population in Taiwan Academia Sinica, Taiwan Duration of the Workshop: Target Groups: Is the workshop geared at an exclusively German or an international audience? Workshop Language: Description of the content of the workshop: Because the corresponding domain knowledge about hard-to-reach population research and Taiwan Indigenous Peoples (TIPs) is not easy to understand for the audience, the instructor will make a very short introduction. The workshop uses a set of open data in TIPD (Taiwan Indigenous Peoples Open Research Data, for details, see https://osf.io/e4rvz/) as an example to demonstrate big data, open data, smart data, data science, and open science. TIPD complies with FARE (Findable, Accessible, Interoperable, Reusable) data principle. Goals of the workshop: Necessary prior knowledge of participants: Literature that participants need to read for preparation Recommended additional literature (2) Lin, Ji-Ping, 2017b, "An Infrastructure and Application of Computational Archival Science to Enrich and Integrate Big Digital Archival Data: Using Taiwan Indigenous Peoples Open Research Data (TIPD) as Example," in Proceedings of 2017 IEEE Big Data Conference, the IEEE Computer Society Press. (3) Lin, Ji-Ping. 2018. "Human Relationship and Kinship Analytics from Big Data Based on Data Science: A Research on Ethnic Marriage and Identity Using Taiwan Indigenous Peoples as Example," pp.268-302, in Stuetzer et al. (ed) Computational Social Science in the Age of Big Data. Concepts, Methodologies, Tools, and Applications. Herbert von Halem Verlag (Germany), Neue Schriften zur Online-Forschung of the German Society for Online Research. (4) Lin, Ji-Ping. 2021. "Computational Archives of Population Dynamics and Migration Networks as a Gateway to Get Deep Insights into Hard-to-Reach Populations: Research on Taiwan Indigenous Peoples," Proceedings of 2021 IEEE International Conference on Big Data, IEEE Computer Society Press. Information about the instructor: Dr. Ji-Ping Lin received his B.Sc. in Geography from National Taiwan University (Taiwan) in 1988, M.Sc. in Statistics from National Central University (Taiwan) in 1990, and Ph.D. in Geography in 1998 from McMaster University (Ontario, Canada). His main research specialty and interests include migration and population studies, labor study, survey study, scientific & statistical computing, big & open data, data science, and open science. He is serving as associate research fellow at Academia Sinica, Taiwan. The instructor worked in Taiwan’s Bureau of Statistics & Census as research scientist, with abundant real-world experiences in processing, integrating, and enriching various sources of large-scale raw data, as well as in survey planning, sampling design, and conducting surveys. Lin has been serving as consultant for a number of Taiwan’s central government agencies. Since 2013, the instructor devotes himself to the research on hard-to-reach population (HRP) and Taiwan Indigenous Peoples (TIPs). Based on the fundamentals of computational social science, data science and open science, he has been building a number of big open data and smart data. Will participants need to bring their own devices in order to be able to access the Internet? Will they need to bring anything else to the workshop? |
1:30pm - 4:30pm | Workshop 5 Location: Seminar 2 (Room 1.02) Session Chair: Ludger Kesting, Tivian, Germany |
|
Flexible Text Categorisation in Practice: Using AI Models to Analyse Open-Ended Survey Responses Tivian, Germany Duration of the Workshop: Target Groups: Is the workshop geared at an exclusively German or an international audience? Workshop Language: Description of the content of the workshop: Goals of the workshop: Necessary prior knowledge of participants: Information about the instructors: Ludger Kesten's educational background is empirical social science and statistics based on my studies of sociology, computer science and ethnology. His focus and interest were always a data driven understanding of connections between people, social groups and cultures now breaking this down to employee and customer experience to help companies to listen to their employees, empower their leaders to drive success. Will participants need to bring their own devices in order to be able to access the Internet? Will they need to bring anything else to the workshop? |
4:30pm - 5:00pm | Break |
5:00pm - 6:30pm | DGOF Members General Meeting Location: Auditorium (Room 0.09/0.10/0.11) |
6:30pm - 7:00pm | Break |
7:00pm - 8:00pm | Early Career Speed Networking Event Location: Franky's Bar - Venloer Str. 403, 50825 Köln |
8:00pm - 11:00pm | GOR 24 Get Together Location: Franky's Bar - Venloer Str. 403, 50825 Köln |
Date: Thursday, 22/Feb/2024 | |
8:00am - 9:00am | Begin Check-in |
9:00am - 10:15am | GOR 24 Opening & Keynote I Location: Auditorium (Room 0.09/0.10/0.11) |
|
Digital monopolies: How Big Tech stole the internet - and how we can reclaim it AMP Digital Ventures, Germany Data measurements prove crystal clear: The huge amount of domains on the internet is meaningless, it is only a handful of tech companies which are attracting the majority of digital traffic. The rest of the internet resembles a huge graveyard. Digital monopolies are currently bringing ever larger parts of our lives under their control. The platforms are increasingly dominating the formation of political opinion and at the same time are deleting our free market economy. We should ask ourselves: is this still legal? Why should we put up with it any longer? Media scientist Martin Andree shows how far the hostile takeover of our society by the tech giants has already progressed - and how we can reclaim the internet. Moreover, the keynote will address the specific role of science and market research, which should take a clear position towards these problems. The current destabilization of Western democracies shows how much people depend on competent scientific media research to provide guidelines and orientation for society in order to save our democracy, especially in times of disinformation and fake news. It’s time to take over responsibility and make a change for the better – as long as it is still possible.
|
10:15am - 10:45am | Break |
10:45am | Track A: Survey Research: Advancements in Online and Mobile Web Surveys sponsored by GESIS – Leibniz-Institut für Sozialwissenschaften |
10:45am | Track B: Data Science: From Big Data to Smart Data |
10:45am | Track C: Politics, Public Opinion, and Communication |
10:45am | Track D: Digital Methods in Applied Research |
10:45am | Track T: GOR Thesis Award 2024 |
10:45am - 11:45am | A1: Survey Methods Interventions 1 Location: Seminar 1 (Room 1.01) Session Chair: Almuth Lietz, Deutsches Zentrum für Integrations- und Migrationsforschung (DeZIM), Germany |
|
Providing Appreciative Feedback to Optimizing Respondents – Is Positive Feedback in Web Surveys Effective in Preventing Non-differentiation and Speeding? Technical University of Darmstadt, Germany Relevance & Research Question Interactive feedback to non-differentiating or speeding respondents has proven effective in reducing satisficing behavior in Web surveys (Couper et al. 2017; Kunz & Fuchs 2019). In this study we tested the effectiveness of appreciative dynamic feedback to respondents who already provide well-differentiated answers and who already take sufficient time in a grid question. This feedback was expected to elevate overall response quality by motivating optimizing respondents to keep response quality high. Methods & Data About N=1,900 respondents from an online access panel in Germany participated in a general population survey on “Democracy and Politics in Germany”. In this study, two 12-item grid questions were selected for randomized field-experiments. Respondents were assigned to either a control group with no feedback, or to experimental group 1 receiving feedback when providing non-differentiated (experiment 1) or fast answers (experiment 2) or to experimental group 2 receiving appreciative feedback when providing well differentiated answers (experiment 1) or when taking sufficient time to answer (experiment 2). Interventions were implemented as dynamic feedback appearing as embedded text bubbles on the question page up to four times and disappearing automatically. Results Results suggest that appreciative feedback to optimizing respondents has only limited positive effects on response quality. By contrast, we see indications of deteriorating effects when praising optimizing respondents for their efforts. We speculate that appreciative feedback to optimizing respondents is perceived as an indication that they process the question more careful than necessary. Comparing various types of attention checks in web-based questionnaires: Experimental evidence from the German Internet Panel and the Swedish Citizen Panel 1GESIS - Leibniz Institute for the Social Sciences, Germany; 2SOM Institute, University of Gothenburg, Sweden Relevance & Research Question Evaluating methods to prevent and detect inattentive respondents in web surveys 1Institute for Employment Research (IAB), Germany; 2LMU Munich; 3University of Mannheim; 4NYU Relevance & Research Question Inattentive respondents pose a substantial threat to data quality in web surveys. In this study, we evaluate methods for preventing and detecting inattentive responding and investigate its impacts on substantive research. We use data from two large-scale non-probability surveys fielded in the US. Our analysis consists of four parts: First, we experimentally test the effect of asking respondents to commit to providing high-quality responses at the beginning of the survey on various data quality measures (attention checks, item nonresponse, break-offs, straightlining, speeding). Second, we conducted and additional experiment to compare the proportion of flagged respondents for two versions of an attention check item (instructing them to select a specific response vs. leaving the item blank). Third, we propose a timestamp-based cluster analysis approach that identifies clusters of respondents who exhibit different speeding behaviors and in particular likely inattentive respondents. Fourth, we investigate the impact of inattentive respondents on univariate, regression, and experimental analyses. First, our findings show that the commitment pledge had no effect on the data quality measures. As indicated by the timestamp data, many respondents likely did not even read the commitment pledge text. Second, instructing respondents to leave the item blank instead of providing a specific response significantly increased the rate of flagged respondents (by 16.8 percentage points). Third, the timestamp-based clustering approach efficiently identified clusters of likely inattentive respondents and outperformed a related method, while providing additional insights on speeding behavior throughout the questionnaire. Fourth, we show that inattentive respondents can have substantial impacts on substantive analyses. Added Value The results of our study may guide researchers who want to prevent or detect inattentive responding in their data. Our findings show that attention checks should be used with caution. We show that paradata-based detection techniques provide a viable alternative while putting no additional burden on respondents. |
10:45am - 11:45am | B1: Large, Larger, LLMs Location: Seminar 3 (Room 1.03/1.04) Session Chair: Daniela Wetzelhütter, University of Applied Sciences Upper Austria, Austria |
|
Free Text Classification with Neural Networks: Training, Process Integration and Results for ISCO-08 Job Titles 1Inspirient GmbH, Germany; 2Verian Group, Germany Relevance & Research Question: Manual classification of job titles into classification systems like ISCO-08 is a time-consuming and labor-intensive task that often suffers from inconsistencies due to differences in expertise. The naïve approach of using lookup tables, while effective for a limited number of samples, falls short when handling larger and heterogeneous datasets, having different spelling styles for the same job. To address these challenges, our research seeks to answer the question: Can we build a classifier that outperforms the naïve approach and significantly improves the process of job title classification into ISCO-08? Methods & Data: After a rigorous exploration of various machine learning approaches, we identified feedforward neural networks as the most effective for our task. Our training dataset comprises a diverse collection of approximately 100,000 distinct job titles (German), each meticulously labeled with the corresponding ISCO-08 code by human experts. Results: In our classifier, around 60% of classifications demonstrate confidence levels of 90% or higher, achieving an impressive accuracy of approximately 94% for those classifications. This allows for swift review of about 60% of the data. The overall accuracy of the classifier is approximately 73%, when no low confidence classifications are discarded. Despite this, manual review remains crucial for ensuring correctness, but the classifier significantly speeds up the process. Furthermore, the classifier provides three ISCO-08 recommendations with confidence scores for each, giving human experts a solid reference point for manual classification, even in cases of lower confidence. Added Value: Our data science approach offers several valuable benefits. Firstly, it significantly reduces the time investment required for this process, enabling researchers to process larger datasets efficiently. Secondly, it enhances the consistency of job title classification into ISCO-08, mitigating the issues associated with completely manual classification. Lastly, our classifier's accuracy is expected to improve even further as it continues to learn from more examples and corrections over time, making it a valuable and ever-evolving tool for labor market research. First tests show that the same approach works very well with other national and international economic and social classifications (e.g. ISCED, KldB2010). Where do LLMs fit in NLP pipelines? Q Agentur für Forschung GmbH, Germany Relevance & Research Question Large language models (LLMs) can perform classic tasks in natural language processing (NLP), such as text classification, sentiment analysis and named entity recognition. It is tempting to replace whole pipelines with an LLM. But the flexibility and ease of use of LLMs comes at a price: their throughput is low, they require a provider like OpenAI or one’s own GPU cluster and have high operating cost. This study aims to evaluate the practicality of LLMs in NLP pipelines by asking, "What is the optimal placement of LLMs in these pipelines when considering speed, affordability, adaptability, and project management?”. Methods & Data This study utilizes a mixed-method approach of benchmarks, economic analysis and two case studies. Model performance and speed are assessed on benchmarks. Economic considerations stem from prices for machine learning workloads on cloud platforms. The first case study is on social media monitoring of a patient community. It is centered on an LLM that performs multiple tasks using in-context instructions. The second case is large-scale monitoring of cosmetics trends using a modular pipeline of small models. Results Small neural networks outperform LLMs by over 100-fold in throughput and cost-efficiency. Yet, without parameter training, LLMs attain high accuracy benchmark scores through in-context examples, making them preferable for small scale projects lacking labeled training data. They also allow flexibility of labeling schemes without retraining, which helps at the proof-of-concept stage. Further, they can be used to aid or automate the collection of labeled examples. Added Value LLMs have only recently become available for many organizations and drew new practitioners to the field. A first instinct may be to treat LLMs as a universal solution for any language problem. The aim of this study is to provide social scientists and market researchers with references that help them navigate the tradeoffs of using LLMs versus classic NLP techniques. It combines theory with benchmark results and practical experience. Sentiment Analysis in the Wild 1Bilendi & respondi, France; 2Université Paris Nanterre, France Relevance & Research Question Methods & Data We used a dataset of 167K pairs of the form <comment, rating> in English and French where participants were routinely asked to provide 0-10 ratings to evaluate how much they appreciated a certain product AND to provide a comment explaining their thoughts in connection with the rating. Then we did assess the different SA models on how good they can guess participants’ rating based on their comment? Since SA models typically predict SA on a five point/stars scale, the question is whether those stars somehow match the ratings given by the participant. We compared the google NLP suite vs NLP Town, an open source multi-lingual neural network model based on BERT. Basically, a multilingual BERT finetuned for sentiment analysis on 629k amazon reviews. The perforamance vary between 50% and 70%. In this context, qual/quant data is useful to fine-tune open-source models such as NLP Town. But the risk of generalization failure is very real. It proved very useful to supplement our qual/quant data by bringing in some extra open data (Wikipedia extracts as paradigmatic descriptive verbatims) However, few shot learnings and last gen LLMs such as ChatGPT 4 do change the game by providing an ‘effortless’ (but still not perfect) solution. Added Value Fondamental research… - assessing reliability of AI in NLP - comparing the reliability of different AI models - identifying challenges and limitations |
10:45am - 11:45am | C1: Media Consumption Location: Seminar 4 (Room 1.11) Session Chair: Felix Cassel, University of Gothenburg, Sweden |
|
Anxiety and Psychological distance as a drive of mainstream and online media consumption during war The Max Stern Yezreel Valley College, Israel Relevance & Research Question This study examines media consumption patterns among Israeli users, during the 2023 Israeli-Hamas war. Drawing from the extensive body of literature on media use during wartime, this study investigates how civilians utilize different channels and platforms to fulfill their needs and perspectives amid this violent conflict. Specifically, consumption patterns will be analyzed as a function of users’ level of anxiety, and their psychological distance from the war. We hypothesized that (1) The extent of individual anxiety will predict differences in mainstream versus online media usage, and that (2) Psychological distance from the war will mediate the relationship between anxiety and media usage patterns. Methods & Data A structured questionnaire was delivered among a nationally representative sample of Jewish -Israelis aged 18 and above (n=500) during the third week of the war, October 2023. Maximum standard error was set at 4.5%. Sample size calculations conducted using G*Power were based on a medium-sized effect size to achieve 90% power in detecting significant differences. Results To test our first hypothesis (H1), a multiple regression analysis assessed the impact of anxiety on the usage of mainstream versus online media. The results indicated that anxiety significantly predicted an increase in mainstream media usage (B = .039, p < .05) but had no significant impact on alternative media usage (B = -.097, p > .05). suggesting that higher levels of anxiety were associated with a preference for mainstream media. The second hypothesis (H2) involved a mediation analysis using Hayes' PROCESS macro. The analysis showed full mediation; the direct effect of anxiety on media usage became nonsignificant when accounting for psychological distance (B = .012, p > .05). However, the indirect effect of anxiety on media usage through psychological distance was significant (B = .053, 95% CI [.023, .129]), indicating that psychological distance completely mediates the relationship between anxiety and media usage patterns during wartime. Added Value This study contributes to the current literature on media consumption during wartime, by focusing on war-related anxiety as a drive, and by adopting ‘psychological distance’ to this field, analyzing it as another relevant variable.
Engagement Dynamics and Dual Screen Use During the 2022 FIFA World Cup Max Stern Academic College of Emek Yezreel Relevance & Research Question We found significant positive correlations between engagement, transportation, enjoyment, and media event perception with match-related and unrelated dual-screen usage. Specifically, the Pearson correlation coefficients were r = .56 for engagement with match-related dual-screen usage (p < .001) and r = .37 for engagement with match-unrelated dual-screen usage (p < .001), highlighting the strong association between these psychological factors and dual-screen behaviors. Added Value This study shows how psychological factors influence dual-screen usage during major sports events like the FIFA World Cup. It provides critical insights for media producers, advertisers, and digital strategists in developing engagement strategies and content for dual-screen platforms. It enriches the discourse on media consumption patterns in the context of global sports events, significantly enhancing our understanding of contemporary media engagement in a multi-screen world. |
10:45am - 11:45am | D1: Best Practice Cases Location: Auditorium (Room 0.09/0.10/0.11) Session Chair: Yannick Rieder, Janssen EMEA, Germany |
|
Brave new world! How artificial intelligence is changing employee research at DHL. 1r)evolution GmbH, Germany; 2DHL Group Relevance & Research Question We try to estimate the possible financial, personnel and time savings by using AI and we want to show how AI can improve the outcome of a research system. Abstract In an annual online survey, DHL Group collects structured and open feedback from around 550,000 employees. This Employee Opinion Survey (EOS) is conducted worldwide in 55 languages and for 60,000 organizational units. Due to its size, the operational implementation of the survey requires the use of large financial and human resources. It is not surprising that various stakeholders expressed a desire to optimize the survey: HR departments wanted more automation and a more effective follow-up process. Team heads wished more specific recommendations for action and top management wanted an optimized use of resources. EOS project team then asked itself how AI could help to make the project simpler, more insightful, and more effective. All process steps were evaluated, and five AI pilots/projects were rolled out. 1. AI to automate survey setup. The challenge is that the reporting structure cannot be derived directly from the formal line organization. And therefore, it’s a major manual effort for the HR departments to assign 550,000 employees to their teams. To optimize the process, we used machine learning models to automatically assign employees to the reporting structure. 2. AI to improve online questionnaire. In online surveys, the answers to open questions often remain short, as there is no interviewer to explore. To fill this gap, we used a GPT model that reacts individually to the respondent's open answer and asks additional in-depth questions. 3. AI to speed up the open comment processing. We used AI to translate, anonymize and categorize the 142,000 open comments in a fully automated process. 4. AI to make results dashboard more user-friendly and effective. We implemented a chatbot in our reporting tool that uses the current OpenAI GPT model. The chatbot starts with an individual management summary and answers specific questions of the users. 5. AI to predict which follow-up measures are particularly effective. We evaluated the initiatives documented in the action planning tool from previous years with next year’s EOS results using an AI-supported decision tree analysis. Using these five AI projects as examples, we show the opportunities, limits and risks of using AI, especially for large research systems. |
10:45am - 11:45am | T1: GOR Thesis Award 2024 Competition: Bachelor/Master Location: Seminar 2 (Room 1.02) Session Chair: Olaf Wenzel, Wenzel Marktforschung, Germany |
|
Fair Sampling for Global Ranking Recovery University of Mannheim, Germany Relevance & Research Question Measuring human perception of attributes such as text readability (Crossley et al., 2023) or perceived ideology of politicians (Hopkins and Noel, 2022) is oftentimes difficult because rating scales are hard to interpret. Pairwise comparisons between candidates—for instance: which of these two politician is more conservative?—pose a viable alternative. Given such pairwise comparisons, the task is to recover a global ranking of all candidates. This is non-trivial because of its probabilistic nature, i.e., a "weaker" candidate might win a comparison by chance. Furthermore, resources are often limited and not all pairs can be compared until a satisfactory estimate of individual strength is reached. Therefore, pairs of individuals must be selected according to a specified sampling strategy. In recent years, a subfield of machine learning has developed around the quantification of fairness. While not without criticism, researchers propose fairness metrics and integrate fairness targets into machine learning algorithms. Lately, algorithmic fairness research has expanded from classification tasks to ranking scenarios as well. Yet, the fairness of ranking recovery from pairwise comparisons remains largely unexplored. This is particularly relevant since measured human perceptions are likely biased. For instance in hiring, pairwise comparisons between candidates for a position might not lead to the identification of the ideal candidate in the presence of such biases. To the best of my knowledge, no previous research is concerned with the combined influence of sampling strategies and ranking recovery methods on the accuracy and fairness of recovered rankings. I thus propose the following research questions:
Methods & Data In this thesis, I present a framework that manipulates the sampling of individuals for comparison in the presence of bias. I simulate individuals with latent "skill scores" on a certain task. I then separate the individuals into two groups and subtract a bias from the scores of the "unprivileged" group. I implement three distinct sampling strategies for selecting individuals from both groups for comparison: (1) random sampling (2) oversampling the unprivileged group and (3) sampling by previous success. Using the Bradley-Terry model (Bradley and Terry, 1952), I then simulate pairwise comparisons between the sampled individuals. On the simulated pairwise comparison data, I apply various ranking recovery methods including basic heuristics (David, 1987) and a state-of-the art method that involves graph neural networks: GNNRank (He et al., 2022). Further, I recover rankings with Fairness-Aware PageRank (Tsioutsiouliklis et al., 2021)—an algorithm developed for a different task, that is, however, group-aware and aims at eliminating bias. In order to evaluate the interaction between sampling strategies and ranking recovery methods, I propose a novel group-conditioned accuracy measure tailored towards ranking recovery. Using this measure, I am able to evaluate both the overall accuracy of the recovered ranking, but also its fairness as operationalized through group representation (exposure) and group-conditioned accuracy. I provide a Python package under MIT license to facilitate replication of my findings as well as for further investigation of fairness in ranking recovery. Results Regarding the effect of sampling strategies, I find that both oversampling and rank-based sampling harm the accuracy of the recovered ranking. This is surprising as we would expect oversampling to improve the ranking accuracy of the unprivileged group that is oversampled. However, since this group's ranking accuracy also depends on correct comparisons against the individuals of the other group, the oversampled group's accuracy suffers as well. Oversampling thus is not a good remedy against biased comparisons. In scenarios where there is no bias present against the unprivileged group, the optimal choice of ranking recovery method depends on the sampling that was used before pairwise comparison. Under random sampling, more advanced methods add little to no benefit in accuracy compared to heuristics based methods (i.e., David's Score). When oversampling or rank-based sampling is applied, however, GNNRank outperforms the other methods. In the presence of bias against the unprivileged group, Fairness-Aware PageRank outperforms all other ranking recovery methods. Not only does it mitigate group representation bias from the recovered ranking, it also improves the ranking's accuracy when measured against the unbiased, latent "skill scores". This highlights the importance of group-aware ranking recovery over marginal benefits observed between the other ranking recovery methods. Added Value This thesis bridges the gap between previous research on fairness in machine learning and ranking recovery from pairwise comparisons. It is the first to introduce a framework for systematic investigation of fairness in ranking recovery and focusses on real-world sampling strategies and existing ranking recovery methods. Further, I propose a novel group-conditioned accuracy measure tailored towards ranking recovery. The results highlight the importance of fairness-aware ranking recovery methods and I supply recommendations on which ranking recovery method to use under which circumstances. Understanding the Mobile Consumer along the Customer Journey: A Behavioural Data Analysis based on Smartphone Sensing Technology 1Technische Hochschule Köln, Germany; 2Murmuras GmbH, Germany Relevance & Research Question: Digitalisation is shaping a new consumption era characterised by high connectivity, mobility and a broad range of easily accessible information on products, prices and alternatives. The modern consumers are broadly connected via social media and more mobile than ever with their smart devices. This empowers consumers to make sophisticated buying decisions based on a comprehensive amount of easily accessible online information, while having a broad range of options to choose from. Moreover, they compare prices, ask for opinions online and are willing to choose alternative products or services if they fit better in their lifestyle and meet their needs. As a result, it becomes more difficult than ever to understand modern consumers along their complex and dynamic path to purchase. However, since the modern consumers are constantly online through their smartphones, they produce a notable amount of data about their mobile and online behaviour such as movement, social media activities, online purchases or google searches. This behavioural data is immensely valuable for companies because it allows them to get a deep understanding about the mobile consumption behaviour of their customers. Yet, there is no solution on how to use this data to follow the consumers on their mobile devices. Therefore, this thesis investigates the extent to which mobile data collected with sensing technologies is useful to describe mobile consumer behaviour. The goal was to propose a first approach on how mobile data can be analysed to understand mobile consumers along their customer journey. For this purpose, an explorative analysis was conducted based on the following research question: What analyses can be performed using data generated with smartphone sensing technology to understand mobile consumer behaviour along the customer journey? Methods & Data: As a first step, a literature review on current customer journey analytics theories, models and practices was conducted as foundation for the explorative data analysis. Because there could not be found any reasonable research that focuses on analysing customer journeys from mobile consumers, a mobile customer journey model was developed by adapting current models that are used among practitioners in customer journey analytics. For the data analysis, the author collaborated with Murmuras, which developed a smartphone sensing technology for collecting sensing data via an application on participants’ mobile phones. The collection process adheres to GDPR compliance standards, with data exclusively stored on servers located in Germany. Importantly, no personal information is tracked; instead, only consumption-relevant data is recorded. The company runs an ongoing incentivised smartphone sensing panel with a constant participant basis of approximately 1.500 smartphone users in Germany. Because of this, the thesis could be provided with long-term data from 01.10.2021 to 31.08.2022. This mainly included app usage data as well as mobile browser data (e.g. google search terms, website visits, etc.) and specific in-app content such as advertisement in the Facebook and Instagram app and in-app shopping content from the Amazon shopping app. The data was provided and analysed via the platform Metabase, which mainly uses SQL-programming for analysing data. As the author has previous experience working with the data and analytics platform during a student internship, this knowledge could be used to transfer the mobile customer journey model into analytics concepts. Based on that, an explorative data analysis was conducted to explore the full potential of sensing data in the context of customer journey analytics. Results: The results show that mobile sensing data can be used in three main research areas among customer journey analytics: examining the touchpoint performance of a brand across mobile apps, describing different target groups by their smartphone usage behaviour and deriving real customer journeys on users’ devices. For these areas interactive dashboards using different types of sensing data were developed. The first dashboard focuses on analysing the touchpoint performance across various sensing datasets, including general app usage, in-app advertising, browser data, and Amazon shopping data. Key Performance Indicators (KPIs) were calculated to assess both general and app-related touchpoint performance. The integrated mobile customer journey provides an overview of all brand touchpoints over time, with detailed analyses of ads, browser interactions, and shopping behaviour. The second dashboard dives into target group analysis, aiming to understand mobile behaviour and preferences by providing insights into demographics, smartphone usage habits, contact channels, and mobile shopping behaviours on Amazon. The last part of the analysis employs the dashboards to conduct a deep analysis of an individual brand customer. This involved identifying relevant touchpoints, observing intercorrelations between touchpoints, analysing phone and mobile shopping habits, and mapping the customer journey stages. The insights gained from this analysis contribute to a comprehensive customer journey map and offer opportunities for the brand based on a deeper understanding of the consumers’ mobile life. Added Value: Although the vast amount of sensing data and the complexity of its analysis in the context of customer journey analytics remains challenging, it could be shown that sensing data presents a big opportunity for companies and researchers in this research area. It is not only possible to follow the relevant customers on their complex path to purchase, but also act on it by having the knowledge on how and where exactly to interact with their customers in the mobile world. As this has been a blind spot for companies and researchers before, they now have the ability to decode the whole customer journey of target groups by combining existing data with the insights derived from mobile sensing data. As sensing technology and sensing capabilities as well as smart devices are constantly improving, it is expected that an even more complete picture of mobile customer journeys can be analysed, which will add further value to customer journey analytics in future. Effects of active and passive use on subjective well-being of users of professional networks TH Köln, Germany Relevance & Research Question: Over the past decade online networking platforms have become integral parts of everyday life for most people, reshaping the way individuals communicate and network both privately and professionally. The growing popularity of these sites has sparked both enthusiasm and apprehension, resulting in a heated debate on the negative consequences of social network site (SNS) use on users’ well-being in both popular culture and academia. Almost simultaneously with the rise of private network sites such as Facebook, professional network sites (PNSs) including LinkedIn have gained popularity. Despite the great interest in usage patterns (active and passive use) and the negative effects of SNS use on users’ well-being, relatively little research has been performed on PNSs. Especially the association between PNS use and well-being has received very little academic attention so far. In view of the increasing popularity of PNSs for both private users and organizations this is surprising. Examining the impact on well-being is important as PNSs become more popular, leading to an increasing number of users who may be affected by the potentially harmful consequences such as decreased satisfaction with life, increased depressive symptoms or loneliness some authors have previously attributed to SNS use. The aim of this study was to transfer previous findings on SNS use to the context of PNSs, exploring the multifaceted relationship between usage patterns and users’ well-being leading to the following research questions: RQ1 What is the relationship between PNS usage type and users’ subjective well-being? RQ2 What factors play a role in determining the influence of PNS usage type on the subjective well-being of the users? RQ2.1 How does bridging social capital influence the relationship between active use and users’ subjective well-being? RQ2.2 How do social comparison and envy influence the relationship between passive use and users’ subjective well-being? Methods & Data: A quantitative online survey was conducted which yielded an adjusted total sample of 526 LinkedIn users (173 male, 350 female, 2 diverse, 1 undisclosed) aged 19 to 65 (M = 28.69, SD = 8.66). A convenience sample was recruited using WhatsApp, LinkedIn and university mailing lists. Additionally, three survey sharing platforms (i.e. SurveyCircle, SurveySwap and PollPool) were used. According to the active-passive model of SNS use (Verduyn et al., 2017), which was employed as the theoretical framework for this thesis and transferred to the context of PNSs for this purpose, the effects of active and passive use on users’ subjective well-being are explained by three mediating variables: social capital for active and social comparison as well as envy for passive use. Followingly, participants were asked to fill out measures regarding their usage pattern on LinkedIn, their subjective well-being, their tendency to engage in social comparison behavior, their experiences with envy as well as their levels of social capital. Three mediation analyses were run using the PROCESS add on (Hayes, 2013) for IBM SPSS 28.0.1.0. To test the relationship between active LinkedIn use and subjective well-being, which was predicted to be mediated by bridging social capital, a simple mediation model was tested (model 1). Next, a serial mediation analysis was run to test the relationship between upward social comparison and envy as mediators in the relationship between passive LinkedIn use and subjective well-being (model 2). The same procedure was repeated, replacing upward comparison by downward social comparison (model 3). Results: Results of the mediation analyses revealed an indirect positive relation between active use of LinkedIn and well-being. Conversely, a negative indirect relation was found between passive use of LinkedIn and subjective well-being. Bridging social capital fully mediated the relationship between active LinkedIn use and well-being (significant positive indirect effect ab = .0624, 95%-CI [.0303; .0999] and insignificant direct effect c’ = .0967, p = .1237, 95%-CI [-.0191; .1585]). As predicted, social comparison and envy acted as serial mediators in the relation between passive LinkedIn use and subjective well-being (model 2: a1d21b2 = -.0347, 95%-CI [-.0583; -.0120]; model 3: a1d21b2 = -.0101, 95% CI [-.0217; -.0009]). Though, results of the two mediation models examining passive LinkedIn use indicated possible omissions of other mediating variables as the direct effect between passive LinkedIn use and subjective well-being (model 2: c’ = .1692, p < .001, 95%-CI [.0904; .2481]; model 3: c’ = .1433, p < .001, 95%-CI [.0651; .2215]) remained significant after the mediator variables were added to the model. Added Value: The results of this thesis further expand upon previous research by examining users of PNSs. This study extends prior findings of other studies in two ways. First, it advances literature on online networking site use and well-being as it explores PNS use. Previous research mainly examined the relation between SNS use and well-being with special attention to Facebook. Moreover, prior studies have mainly focused on examining either passive or active use, while this study examined both usage patterns at once. While results of this study are preliminary and should not be generalized, findings suggest that SNSs and PNSs share similarities, that lead to similar effect patterns when examining the relationship between usage patterns and well-being. Testing the active-passive model of SNS use (Verduyn et al., 2017) in the context of PNSs, revealed appropriate applicability. Results of this thesis also have practical relevance for both users and creators of platforms like LinkedIn. Active use behavior should be promoted and encouraged as it has been associated with positive affects on users’ well-being. When being educated on the different effects of usage patterns, users can proactively change their behaviors, positively affecting their well-being. |
11:45am - 12:00pm | Break |
12:00pm - 1:15pm | A2: Mixing Survey Modes Location: Seminar 1 (Room 1.01) Session Chair: Jessica Daikeler, GESIS, Germany |
|
Navigating the Digital Shift: Integrating Web in IAB (Panel) Surveys Institut für Arbeitsmarkt- und Berufsforschung, Germany Relevance & Research Question In the realm of social and labor market research, a noteworthy transformation has unfolded over the past few years, marking a departure from conventional survey methods. Traditionally, surveys were predominantly conducted through telephone interviews or face-to-face interactions. These methods, while effective, were time-consuming and resource-intensive. However, with the rapid advancement of technology, there has been a significant paradigm shift towards utilizing online modes for data collection. The emergence of the web mode has revolutionized the landscape of surveys, offering a more efficient and cost-effective means of gathering information. Online surveys provide researchers with a broader reach, enabling them to engage with diverse populations across geographical boundaries. Moreover, the convenience and accessibility of web-based surveys have contributed to increased respondent participation. As we navigate the digital age, the web mode has become increasingly integral in shaping the methodologies of social and labor market research. Its versatility, speed, and ability to cater to a global audience underscore its growing importance in ensuring the accuracy and comprehensiveness of data collection in these vital fields. Methods & Data In this paper, we focus on the largest panel surveys conducted by the Institute for Employment Research. These include the Panel Labor Market and Social Security (PASS), the IAB Establishment Panel (IAB-EP), the Linked Personnel Panel (LPP), consisting of both employer and employee surveys, and the IAB Job Vacancy Survey. Historically, all these surveys employed traditional data collection methods. However, in recent years, they all have undergone a transition by incorporating or testing the inclusion of the web mode. The incorporation of the web mode in key Institute for Employment Research panel surveys is crucial in the digital age. This transition enhances efficiency, reduces costs, and broadens participant diversity, ensuring studies remain methodologically robust and adaptable to the evolving digital landscape. Effect of Incentives in a mixed-mode Survey of Movers University of Bonn, Germany Relevance & Research Question The use of incentives to reduce unit nonresponse in surveys is an established and effective practice. Prepaid incentives have been shown to increase participation rates, especially for postal surveys. As surveys keep moving online and response rates keep dropping, the use of incentives and its differential effect on survey modes need to be further investigated. In our experiment, we investigate both the effects of survey mode and incentives for participation rates in a postal/web mixed-mode survey. In particular, we aim to answer the questions: i) In which sociodemographic groups do incentives work (particularly well)? ii) Is the effect of incentives affected by survey mode? iii) How does data quality differ between incentivized and non-incentivized participants? Methods & Data Our data is based on a random sample of all residents who moved from two neighborhoods of Cologne, Germany, between 2018 and 2022. Addresses were provided by the city's Office for Urban Development and Statistics. We were also provided with the age and gender of all selected residents as reported on their official registration. For the experiment, we randomly selected 3000 persons. Of those, 2000 received postal invitations to a web survey, while 1000 received a paper questionnaire with the option to participate online. In both groups, 500 participants were randomly selected to receive a prepaid incentive of 5 euros cash with the postal invitation. Results Our design yielded a good response rate of around 35% overall (47% with incentives and 26% without). Over 80% participated in the online mode. As we have information on the age and gender of the whole sample, including non-responders, detailed analyses on the effectiveness of incentives and their possible effect on data quality (measured by the share of “non-substantive” answers, response styles, and the amount of information provided in open-ended questions), will be presented. Added Value With this paper, we contribute to the literature on the effect of incentives, particularly on the comparison of survey modes. As our data is based on official registration and we have reliable information on non-responders, our results on the effects of incentives are of high quality. Mode Matters Most, Or Does It? Investigating Mode Effects in Factorial Survey Experiments 1Institute for Employment Research, Germany; 2University of Bamberg, Germany; 3Ludwig-Maximilians University Munich, Germany Relevance & Research Question Factorial survey experiments (FSEs), such as vignettes, have increased in popularity as they have proven to be of great advantage when collecting opinions on sensitive topics. Generally, FSEs are conducted via self-administered interviews in order to allow the participant to understand and assess the given scenario entirely. However, many establishment panels, such as the BeCovid establishment panel in Germany, rely on interviewer-administered data collection (e.g. telephone interviews), but could also benefit from using FSEs when interested in collecting opinions on more sensitive topics. Thus, the question emerges whether FSEs conducted via telephone result in similar results compared to web-based interviews. Furthermore, it would be of great interest to know whether these modes differ in their answer behavior for FSEs, such as straightlining, extreme responding or item non-response. Methods & Data To shed light on this issue, a mode experiment was conducted in the BeCovid panel in which a random subset of telephone respondents was assigned to complete a vignette module online (versus continuing with the telephone mode). Respondents were given a set of 4 vignettes varying in six dimensions followed by two follow-up questions regarding the person’s success in the application process. Additional to various descriptive analyses we run multilevel regressions (random intercept model) to take the multiple levels into account. Results The analysis shows no overall difference in the results of the random intercept model when controlling for the mode. However, there are significant differences between the modes regarding specific dimensions of the vignette, which could be described as sensitive. Furthermore, CATI shows an increase of straightlining as well as extreme responding, but no influence on the probability of acquiescence bias or central tendency bias. Lastly, respondents interviewed via telephone lead to more item non-response. Added Value This study shows that conducting FSEs through telephone interviews is feasible, but is associated with certain limitations. Depending on the subject matter, these interviews might fail to accurately capture genuine opinions, instead reflecting socially accepted responses. Additionally, they may result in diminished data quality due to satisficing and inattention.
|
12:00pm - 1:15pm | B2: AI Tools for Survey Research 1 Location: Seminar 3 (Room 1.03/1.04) Session Chair: Timo Lenzner, GESIS - Leibniz Institute for the Social Sciences, Germany |
|
In Search of the Truth. Are synthetic, AI generated data the future of market research? Human8 Europe, Belgium Relevance & Research Question Generative AI is the most talked-about topic among insight professionals, with 93% of researchers seeing it as an opportunity for the industry. With the rise of generative AI also came the rise of synthetic data. This data is artificially generated through machine learning techniques, rather than being observed and collected from real-world sources. Are we at the start of an era? What if synthetic data is taking over – being faster, cheaper, better (also in terms of privacy)? To understand the potential of generative AI systems, Human8 has been on a journey to conduct what we like to call ‘research on research’. Our primary focus has been on understanding how generative AI impacts qualitative research. Methods & Data By developing a personal AI research assistant using ChatGPT as an algorithm, we have been able to experiment with AI on also confidential research data and put generative AI to the test. After we had conducted research with an online community of n=86 human participants, we created a synthetic pendant. With the help of AI and internet data, we developed an online community with 86 synthetic participants that were statistically and structurally identical to the original training data. We asked them the same research questions we had asked the human participants before. We created an artificial dataset with the same characteristics as the real-world dataset but without including any real-world data. And, we compared the outcome. Results The results show very clearly how the conditions of ChatGPT influence study results. We will share the findings of our experiments and the learning for using synthetic data moving forward. Added Value Our presentation will help better understand the differences between data collected from human beings and synthetic, AI generated data. We will explain the reasons-why and provide some guidance on the cases in which the use of synthetic, AI generated data can be beneficial and, where the use of AI involves a risk. ChatGPT as a data analyst: focus on the benefits and risks 1University of Applied Sciences Upper Austria, Austria; 2Johannes Kepler University Linz, Austria Relevance & Research Question: Simple descriptive results can (now) be generated with ChatGPT in a relatively resource-efficient way - e.g. by generating a syntax code at the push of a button and then supporting the user in interpreting the output. The skills required to formulate the prompts and validate the descriptive results generated are still rather "manageable" compared to more complex analysis procedures (e.g. classification analysis). Errors that can occur start with the use of inappropriate analysis methods and range from the use of incorrect syntax to incorrect interpretation of results. This leads to the question: what are the benefits - and what are the risks of generating (and possibly using) incorrect results - of the new possibilities offered by AI-based data analysis? Methods & Data: Based on this, the article will focus on the following aspects (using replication datasets with available, tested syntax codes) in the course of replication of already published studies - Errors in the generated syntax code (e.g. omitting important steps, suggesting inappropriate statistical tests) - Number of trials required (until an acceptable result is obtained or it is determined that no 'acceptable' result can be obtained) - Usefulness of the results (e.g., clarity of interpretation, compactness). Results: The findings can be summarised as follows. The use of tools such as ChatGPT (1) is convincing for generating a decision basis for the choice of analysis method. (2) can support simple descriptive data analysis, result description and interpretation in a resource-efficient way. (3) is only advisable for generating a syntax for carrying out a complex procedure (e.g. MDS) with the appropriate expertise (possible for checking and adapting various specifics) - as it is very error-prone. Added Value: The presentation focuses on the application of AI-supported data analysis in 'everyday research', which can be amateurish in nature, and emphasises the need to have the necessary skills to ensure the required quality of results. The aim of the resulting research is to develop a specific strategy for efficient 'scientific use'. Chatbot Design as an Alternative to a Mobile First Design in Web Surveys: Data Quality and Respondent Experience Technical University of Darmstadt, Germany Relevance & Research Question The increasing use of smartphones in Web surveys requires an optimized questionnaire design for smartphones (Dillman et al., 2104). Since instant messaging is an integral part of every-day communication, this study investigates whether an instant messaging interface (chatbot design) can be used to improve the respondents’ experience with Web surveys and whether data quality is comparable to Web surveys using a mobile-first design. Methods & Data In 2020, a survey on “Implications of COVID-19 on the Student’s life” has been administered to a sample of 280 university students in Germany. Participants were randomly assigned to either a chatbot design or a mobile-first design. About half of the respondents in each design was invited to use their Smartphone to answer the survey while the other half was instructed to use a large screen device. Results Added Value According to this study, the use of a chatbot design improves the respondents’ experience with Web surveys even though the new chatbot design is still rated more difficult compared to the more traditional mobile-first design. Also, a chatbot design has the potential to minimize the burden (response time) of smartphone respondents. Results concerning data quality exhibit a so far inconsistent picture of the chatbot design that asks for a comprehensive assessment. |
12:00pm - 1:15pm | C2: Online research, attitudes, preferences, behavior Location: Seminar 4 (Room 1.11) Session Chair: Dana Weimann Saks, The Max Stern Yezreel Valley College, Israel |
|
Correlating Abortion Attitude Measures Across Surveys: A Novel Approach to Leveraging Historical Survey Data University of Michigan, United States of America Relevance & Research Question The wealth of survey data amassed over the last century represents an invaluable tool for understanding human beliefs, attitudes, and behaviors and how these have evolved. But although thousands of datasets are available to researchers, scholars are often unable to use more than a handful for any given project. One challenge is that many questions, even those asking about similar topics, employ different wordings and response options. Hence, it is often difficult to tell whether differences between responses to questions are indicative of items that track subtly different topics, methodological choices, or changes over time. Instead, scholars examining trends often limit analyses to the subset questions asked identically at multiple time points. The current study proposes a novel solution to identifying common questions across data collections. Methods & Data Using microdata from over 2000 distinct probability US surveys of abortion attitudes, we produce a vector of means for each abortion measure at the intersections of age, gender, race, religion, and location. These can then be correlated across surveys (with appropriate weighting) to determine how similar the measures are and to identify measures that appear to capture similar underlying constructs (through clustering and other dimension reduction). We then parameterize how estimates of that similarity shift depending on the data collection methods, survey firms, and the temporal distance between surveys. We show that this technique allows us to (1) identify the different types of historical questions that exist to measure views on abortion, (2) discern the similarity of those different types of questions, and (3) estimate how attitudes toward different types of questions have trended over time both overall and within population subgroups. We also find that stability of measures is relatively consistent for relations between items asked within 100 days of one-another, whereas it drops notably with longer time differences between measures. The study opens up novel methods for analysis of historical survey data. Does survey response quality vary by respondents’ political attitudes? Evidence from the GGGS 2021 University of Bonn, Germany Relevance & Research Question German General Social Survey (ALLBUS/GGGS 2021), https://doi.org/10.4232/1.14002. The GGGS is a biennial survey based on a random sample of the German population. In 2021, due to the Covid-19 pandemic, it was fielded as a mail / web survey for the first time. The questionnaire was distributed in three randomized split versions. In the first step, indicators for response quality are constructed separately for each split version. These include non-substantial “response styles”, such as extreme responding and non-differentiation, as well as the proportion of item non-response. Subsequently, we conduct regression analyses with political attitudes (e.g. political interest, positions towards cultural and economic issues, intention to vote in upcoming election) as explanatory factors of response quality while controlling for socio-demographic variables, survey mode and number of contact attempts. The analyses show that differences in response quality in the GGGS 2021 are systematically related to age, education and political interest as well as other political attitudes. Building the city: a novel study on architectural style preferences in Sweden University of Gothenburg, Sweden Relevance & Research Question Frequency Matters? Assessing the Impact of Online Interruptions on Work Pace 1Max Stern Academic College of Emek Yezreel; 2Shenkar College of Engineering, Design and Art Relevance & Research Question |
12:00pm - 1:15pm | D2: Innovation in Practice: LLMs and more ... Location: Auditorium (Room 0.09/0.10/0.11) Session Chair: Stefan Oglesby, data IQ AG, Switzerland |
|
Beyond Reports: Maximizing Customer Segmentation Impact with AI-Driven Persona Conversations Factworks, Germany Relevance & Research Question Segmentation is a challenging, core strategic research task, enabling businesses to understand the many needs of diverse customer groups. Yet, its effectiveness lies in its adoption within an organization. Despite AI's pervasive influence, its potential benefit in segmentation studies remains underutilized. This prompts us to explore: To what extent can AI help us socialize segmentation research, enabling stakeholder interaction with data and driving organizational adoption to influence business outcomes? Our research introduces an innovative approach leveraging AI-driven persona chatbots, tapping into Language Model-based systems like ChatGPT. Our aim is to create an interactive chatbot that can be shared across organizational departments, facilitating in-depth familiarization with customer segments. To do that, we train a GPT model on a comprehensive dataset combining quantitative and qualitative research findings from a study on segmenting online travel booking site users. This will enable us to evaluate its potential as a tool to humanize research findings and share accurate information about identified segments. Our research plans to assess the chatbot's capacity to uphold factual accuracy based on its training data while also exploring its ability to generate creative yet aligned responses consistent with the characteristics of the segmented customer groups it represents. Initial assessments showcase promising signs of the chatbot's capacity to navigate between factual accuracy and creative engagement, aligning well with the segmented customer profiles it represents. However, its effectiveness heavily depends on well-engineered prompt design. Added Value In embracing this innovative approach, our goal is to create a tool that aids organizations in unlocking the full potential of segmentation. By encouraging greater immersion and fostering deeper empathy with consumer segments, our persona chatbot aims to make research findings more accessible to wider, less research-savvy audiences and enables them to get to know segments in a more playful and engaging way. How good are conversational agents for online qualitative research? 1Université Paris Nanterre, France; 2Bilendi Relevance & Research Question Conversational agents such as chatGPT open up new ways for online qualitative research. On the analysis side, they may be used to extract key ideas and to provide participants’ quotes illustrating those. On the field management side, they may be used for moderation, to help dig deeper into what participants think. However, beyond the obvious advantages in terms of feasibility, numbers, speed and costs, the question how AI supplemented research design fares compared to purely human driven research appears as a pressing and hard to address question. Our goal in this research is more precisely to assess the quality of AI supplemented qualitative research for analysis and moderation, by comparison with human standards. Methods & Data We shall compare results obtained with and without a chatGPT powered AI assistant on a recently launched qualitative research platform www.bilendi.de/static/bilendi-discuss enabling the use of such an assistant. Regarding analysis, we qualitatively compare the results of a pure human analysis with those of the ChatGPT powered analysis. Regarding moderation, we quantitatively compare the response rate of participants to human moderators vs the chatGPT powered moderator. The data will consist in two data sets, a first study run in Finland in September 2023 gathering 22 participants, and a second study run in France and Germany and the UK over November-December 2023 gathering 30 participants per country. A pilot was run for this second demo in France in November 2023 with 225 participants. In the Finnish study (analysis only), ideas provided by the ChatGPT powered assistant were found to be 70% consistent with those of the human analysis, 20% consistent but ‘not usable as such’ and 10% inconsistent. In the pilot study for the second demo (moderation only), response rate to human moderators was 85% and response rate to the ChatGPT powered assistant was 74.63%. Added Value Recent research by Chopra and Haaland (‘Conducting Qualitative Interviews with AI’, Cesifo Working Paper, 2023) provides encouraging evidence in terms of participants engagement and generated insights. The present research develops on those results by coming with systematic comparisons between human and machine performance. Smartphone app-based mobility research intervista AG, Switzerland Thanks to GPS tracking with a smartphone app, a person's mobility behavior can be tracked in great detail. The information obtained on stages, routes, transport use and mobility purposes offers real added value in many areas of research. In this presentation, Beat Fischer explains the methodology, provides insights into the data science behind it and shows case studies with data from the Swiss Footprints Panel. |
12:00pm - 1:15pm | T2: GOR Thesis Award 2024 Competition: PhD Location: Seminar 2 (Room 1.02) Session Chair: Olaf Wenzel, Wenzel Marktforschung, Germany |
|
Challenging the Gold Standard: A Methodological Study of the Quality and Errors of Web Tracking Data 1University of Oxford, United Kingdom; 2The London School of Economics, United Kingdom; 3Universitat Pompeu Fabra, Spain Relevance & Research Question The advent of the Internet has ushered the social sciences in a new era of data abundancy. In this era, when individuals engage with online platforms and digital technologies, they leave behind digital traces. These digital traces can be collected for scientific research through innovative data collection methods. One of these methods, web trackers, has gained popularity in recent years. This approach hinges on the utilization of web tracking technologies, known as meters, encompassing a diverse array of solutions that participants can install onto their devices. These meters enable the tracking of various traces left by participants during their online interactions, such as visited URLs. Historically, web tracking has been upheld as the de facto gold standard for measuring online behaviours. This thesis studies whether this prevailing notion holds true. Specifically, it explores the following questions: is web tracking data affected by errors? If so, what is the prevalence of these errors? To what extend do these errors introduce bias to web tracking measures? What is the overall validity and reliability of web tracking measures? And can we do something to limit the impact of web tracking data on the measurement quality of its measures? Methods & Data To explore these questions, this thesis uses data from the TRI-POL project. The TRI-POL is three-wave survey, conducted between 2021 and 2022, matched at the individual level with web tracking data. Data were collected through the Netquest opt-in metered panels in Spain, Portugal, and Italy, which consist of individuals who have meter(s) already installed in their devices and who can be contacted to conduct surveys. Cross quotas for age and gender, and for educational level, and region were used in to ensure a sample matching on these variables to the general country online populations. The thesis is composed of three interconnected papers. The first paper, “When survey science met web tracking: Presenting an error framework for metered data”, develops and present a Total Error framework for digital traces collected with Meters (TEM). The TEM framework (1) describes the data generation and the analysis process for metered data and (2) documents the sources of bias and variance that may arise in each step of this process. Using a case study, the paper also shows how the TEM can be applied in real life to identify, quantify, and reduce metered data errors. The second paper, “Uncovering digital trace data biases: tracking undercoverage in web tracking data,” adopts an empirical approach to address tracking undercoverage. This is a key error identified in the TEM: the failure to capture data from all the devices and browsers that individuals utilize to go online. The paper uses a new approach to combine self-reported data on participant’s device usage, and paradata about the devices tracked, to identify undercoverage. Moreover, the paper estimates the bias introduced by different undercoverage scenarios, through the use of a Monte Carlo simulations. The third and last paper, “Validity and Reliability of Digital Trace Data in Media Exposure Measures: A Multiverse of Measurements Analysis,” explores the validity and reliability of web tracking data when used to measure media exposure. To do so, the paper uses a novel multiverse of measurements analysis approach, to estimate the predictive validity and true-score reliability of more than 7,000 potentially designable web tracking measures of media exposure. The reliability of the multiverse of measurements is estimated using Quasi-Markov Simplex Models, and the predictive validity of the measures is inferred as the association between media exposure and political knowledge (gains). Furthermore, the paper estimates the effect of each design choice on the reliability and validity of web tracking measures using Random Forests. Results The TEM in the first paper suggest that web tracking data can indeed be affected by a plethora of error sources and, therefore, statistics computed with this data might be biased. Hence, caution should be taken when using metered data for inferential statistic. By clearly showing how web tracking data is collected and analysed, and identifying the errors of web tracking data, the framework allows to develop approaches to quantify those errors, and strategies to minimise them. Furthermore, the thesis shows - in the second paper- that tracking undercoverage is highly prevalent in commercial panels. Specifically, it reveals that across the countries examined, 74% of the panellists studied had at least one device they used for online activities untracked. Additionally, the simulations prove that web tracking estimates, both univariate and multivariate, are often substantially biased due to tracking undercoverage. As an example, across the different scenarios tested, undercoverage can inflate the proportion of participants identified as news avoiders by 5-21 percentage points, an overestimation of 29-123%. This represent the first empirical evidence demonstrating that web tracking data is biased. Moreover, it exposes deficiencies in the practices and procedures followed by both online fieldwork companies and researchers. Focusing on the measurement properties of web tracking measures, the third paper shows that the median reliability of the entire universe of measurements explored is high but imperfect (≈ 0.86). Hence, in general, the explored measures of media exposure capture around 86% of the variance of their true score. Conversely, the predictive validity of the measures is low, given that overall the association between being exposed to media and gaining political knowledge is null. Although most self-reported measures of media exposure have been criticized precisely because of their lack of predictive power, results suggest that this is not limited to self-reports. Hence, with the current evidence, web tracking measures of media exposure cannot be considered an improvement to self-reports. Additionally, results from the Random Forests suggest that the design decisions made by researchers when designing web tracking measurements can have a substantial impact on their measurement properties. Added Value Collectively, this thesis challenges the prevailing belief in web tracking data as the gold standard to measure online behaviours. It shows that web tracking data is affected by errors, which can substantially bias the statistics produced, as well as harm the reliability and validity of the resulting measures. In addition, the thesis demonstrates that high-quality measures can only be achieved through conscious design decisions, both when collecting the data (e.g., making sure all devices are tracked), and when defining how to construct the measurements. Methodologically, the thesis illustrates how a combination of traditional survey and computational methods can be used to assess the quality of digital trace data. The Language of Emotions: Smartphone-Based Sentiment Analysis 1University of St. Gallen, Switzerland; 2LMU Munich Relevance & Research Question: In an era transformed by artificial intelligence (AI) and the surge of voice assistants, chatbots, and other text or speech-based systems generating massive volumes of language data, automated emotion recognition and sentiment analysis have become integral across disciplines ranging from online marketing to user experience research. However, a main challenge has constrained previous research in this field: differentiating subjective emotional experience ("How do I feel in this moment?") from observable emotional expressions ("How do I express my feelings through language?"). While recognizing subjective emotions is of great scientific and practical relevance, the empirical difficulty of obtaining data on subjective emotional experiences and concurrent real-time language samples has limited the research. As a consequences, prior studies and deployed algorithms mainly relied on datasets composed of text or speech data either rated by participants for their emotional content or provided by actors, thereby focusing on emotion expression. Here, the advent of conventional smartphones has provided a novel research tool, enabling the collection of self-reports on subjective emotional experience via apps and the gathering of everyday speech data through the smartphone's keyboard and built-in microphone. The present work leverages the ubiquity of smartphones, utilizing those capabilities to gather authentic text and speech samples, along with self-reported emotional states, bridging the gap between subjective emotional experiences and their linguistic expressions. Thereby, the present dissertation addresses the research question if subjective emotional experience can be associated with and predicted from features in spoken and written natural language. Moreover, it identifies specific language characteristics, such as the use of certain word categories or voice parameters, associated with one’s subjective emotional experience. Finally, this work examines the influence of the context of language production on emotional language. Methods & Data: The present dissertation unfolds across two pivotal studies, employing everyday smartphones to collect rich datasets of both spoken and written language as well as self-reports on momentary emotional experience. Study 1 analyzes subjective momentary emotion experience in more than 23,000 speech samples from over 1,000 participants in Germany (Study 1.1) and the US (Study 1.2). In Study 1.1, participants uttered predetermined sentences with varying emotional valences (positive/neutral/negative) into their smartphones' microphones and self-reported on their momentary emotional states through an app. From the voice logs, vocal parameters (e.g., loudness, pitch, frequency) were algorithmically extracted. On the contrary, in Study 1.2, participants were given the freedom to express their current thoughts and feelings during the speech recordings alongside the emotion self-reports. Here, not only acoustic parameters, but also state-of-the-art word embeddings based on a Large Language Model (LLM) were extracted from participants’ speech. Then, machine learning algorithms were employed to predict self-reported emotional experience from the extracted voice parameters and word embeddings. Also, interpretable machine learning methods were employed to identify the most important vocal features for emotion predictions. Study 2 leverages a dataset of over 10 million typed words from 486 participants to investigate traces of subjective emotion experience in text data. Here, the smartphone’s keyboard was utilized to log data on typing dynamics (e.g., typing speed), word use based on sentiment dictionaries and indirect emotion markers (e.g., use of first person singular), and emoji and emoticon use. Moreover, the logged data were enriched with contextual information on the app where the respective text had been produced as well as the input prompt text (e.g., “Was gibt’s Neues?” on Twitter). This allowed to distinguish between private communication, for example sending a message on WhatsApp, and public communication, like posting on Facebook. As in study 1, self-reported momentary emotional states and overall stable trait emotionality were assessed through an app. Then, descriptive correlations between self-reported emotion measures and language characteristics as well as machine learning models were investigated for different communication contexts and time aggregations (e.g., daily emotional experience vs. momentary emotions). Results: Results from study 1 indicate that while scripted speech offers limited emotional cues, spontaneous speech significantly enhances the prediction accuracy for emotions. Further, speech content showed a superior predictive performance compared to vocal acoustics in the employed machine learning models. Also, for both prompted and spontaneous speech, the emotional valence of the spoken content had no effect on the algorithmic recognition of emotions from vocal features. Finally, interpretable machine learning methods revealed vocal features related to loudness and spectral fluctuation to be most relevant for emotion predictions from vocal parameters. Study 2 reveals that sentiment dictionaries capture subjective emotion experience for large time windows, such as for overall trait emotionality or weekly emotion experience, but are limited for shorter periods, like momentary emotions. Besides those time effects, findings indicate that the context of language production has a significant impact on distinct emotion-related language variations. Most prominently, the use of first-person singular words (e.g., "I," "me") correlated significantly stronger with negative trait emotionality in public communication than in private communication while the use of first person plural (e.g., "we") had a higher correlation with positive trait emotionality for private communication than public communication. Added Value: In conclusion, the present dissertation sheds light on the complex interplay between language and subjective emotion experience. The two studies that underpin this dissertation are among the first pieces of research to collect and scientifically investigate everyday spoken and written language using conventional smartphones over an extended period, illustrating the promises of personal devices as a new data collection tool. Moreover, the present work emphasizes the significance of the context of language production in emotion detection, demonstrating the potential for nuanced context-aware sentiment recognition systems to understand consumer sentiment and enhance user experience. Finally, by highlighting the challenges of current emotion-recognition methodologies, this dissertation contributes to the academic discourse as well as the development of privacy-conscious sentiment detection technologies. Imputation of missing data from split questionnaire designs in social surveys German Institute for Economic Research (DIW Berlin), Germany Relevance & Research Question In face of declining response rates and escalating costs in social survey research, more and more survey projects are switching from traditional face-to-face interviews to much less expensive self-administered online surveys. However, online surveys have comparatively narrow limits in questionnaire length due to a higher susceptibility for breakoffs. Thus, moving online may force survey designers to cut down on the number of questions asked in a survey, potentially resulting in the cancellation of important research projects due to limited resources. In this context, survey projects increasingly adopt innovative new data collection designs promising to reduce questionnaire length without dropping questions entirely from the survey, such as split questionnaire designs. This is achieved by presenting each respondent only randomly assigned subsets of the questionnaire with the goal of imputing the planned missing data originating from this procedure thereafter. This dissertation addresses the imputation of social survey data from split questionnaire designs and the methodological decisions associated with implementing such surveys to facilitate imputation, asking how split questionnaires may be designed and how the resulting data may be imputed such that estimates could be achieved with a satisfying accuracy in practice based on the imputed data.
Methods & Data Through a series of Monte Carlo simulations, drawing on real social survey data from the German Internet Panel and the European Social Survey, this research assesses the accuracy of estimates across various scenarios, encompassing the implementation of both the split questionnaire design and the subsequent imputation. It delves into the impacts of different split questionnaire module construction strategies, varying imputation techniques, the interplay between planned missingness and conventional item nonresponse, and the implications of general-purpose versus analysis-specific imputation on the accuracy of estimates for a multivariate model. In each simulation run, a split questionnaire design is simulated by allocating items to modules, randomly assigning a number of modules to each survey participant, and deleting all data from the modules not assigned. Thereafter, the data are multiply imputed and estimates calculated based on the imputed data. These estimates are then compared to benchmarks calculated from the complete data to assess their accuracy.
Results Main findings from this research involve:
Added Value The insights gleaned from these simulations thus offer valuable guidance and recommendations for future implementations of split questionnaire designs in online surveys: Split questionnaire survey designers should take care to present questions from preferably all survey topics to each respondent and make sure the split questionnaire design does not result in too large amounts of missing data, also taking into account their expectations about additional unplanned nonresponse. Furthermore, researchers applying imputation to these data may need to reduce complexity in the imputation models to some extent, as for example through dimensionality reduction. Finally, if the data are imputed for general purposes, it should be communicated clearly for which kinds of analyses the imputed data could be used and for which analyses an analysis-specific imputation may be needed. Essays on Inference for Non-probability Samples and Survey Data Integration Utrecht University, The Netherlands Relevance & Research Question Probability sample surveys, which are the gold standard for population inference, are facing difficulties due to declining response rates and related increasing costs. Fielding large size probability samples can be cost prohibitive for many survey researchers and study sponsors. Thus, moving towards less expensive, but potentially biased, non-probability sample surveys or alternative data sources (big or digital trace data) is becoming a more common practice. While non-probabilistic data sources offer many advantages (convenience, timeliness, exploring new aspects of phenomena), they also come with limitations. Drawing inference from non-probability samples is challenging because of the absence of a known sampling frame and random selection process. Moreover, digital trace data are often unstructured and require additional analysis to extract the information of interest. Additionally, there is no unique framework for evaluating their quality, and the lack of a benchmark measure can be a problem when studying new phenomena. Furthermore, it is important to evaluate the construct being measured, as it may be different from the one measured by traditional data sources. Thus, from a statistical perspective, there are many challenges and research questions that need to be addressed, such as the possibility of doing inference with non-probabilistic data, the quality of these data, and whether these data sources can replace or supplement traditional probability sample surveys. The focus of this work is on answering three research questions: 1) What is the evolution of the field of survey data integration and what new trends are emerging?, 2) Can probability and non-probability sample surveys be combined in order to improve analytical inference and reduce survey costs?, and 3) How can traditional and digital trace data be combined to augment the information in traditional sources and better describe complex phenomena? Methods & Data The three research questions are addressed by three different studies. The first study presents an original science mapping application using text mining and bibliometric tools. In addition to characterizing the field in terms of collaboration between authors and research trends, it also identifies research gaps and formulates a research agenda for future investigations. From this research, it appears evident that data integration is a broad and diverse field in terms of methodologies and data sources. Thus, the second and third studies explore whether using non-probabilistic data can improve inference or can allow to study new aspects of a complex phenomenon. The second study focuses on the structured and more traditional volunteer web surveys. In order to address the second research question, the paper presents a novel Bayesian approach to integrate a small probability sample with a larger online non-probability sample (possibly affected by selection bias) to improve inferences about logistic regression coefficients and reduce survey costs. The approach can be applied in different contexts. We provide examples from socioeconomic contexts (volunteering, voting behavior, trust) as well as health contexts (smoking, health insurance coverage). The third study relates the analysis of traditional data in combination with unstructured textual data from social media (Twitter, now X). It shows how digital trace data can be used to augment traditional data, thus feeding smart statistics. On this purpose we propose an original general framework to combine traditional and digital trace based indicators. We show an application related to business statistics but it can be applied to all cases where traditional and new data sources are available. Results In the second study, through the simulation and the real-life data analysis we show that the Mean Squared Errors (MSEs) of regression coefficients are generally lower when implementing data integration with respect to the case of no data integration. Also, using assumed probability and non-probability sample costs, we show that potential cost savings are evident. This work is accompanied by an online application (Shiny App) with replication code and an interactive cost-analysis tool. By entering probability and non-probability (per-unit) sample costs, researchers are able to compare different scenarios of costs. These results can be used as a reference for survey researchers interested in collecting and integrating a small probability sample with a larger non-probability one. The third study results in the development of a general framework to combine traditional and digital trace data. This framework is modular and it is composed of three layers, each describing the steps necessary for the technical construction of a smart indicator. The modularity of the framework is a key feature, as it allows for flexibility in its application. In fact, researchers can use the framework to explore different methodological variants within the same architecture, and potentially carry out improvements to specific modules or test for sensitivity of the results obtained at the different levels. Added Value Research in the field of survey data integration and inference for non-probability samples is expanding and becoming increasingly dynamic. Combining different data sources, especially traditional and innovative ones, is a powerful way to gain a comprehensive understanding of a topic, exploring new perspectives, and can result in new and valuable insights. This work significantly contributes to the current debate in the literature by presenting original methodological findings and adopting a broad perspective in terms of analytical tools (text mining, Bayesian inference and composite indicators) and data sources (volunteer web surveys and textual data from social media). Addressing the three research questions, it: a) enhances understanding of existing literature, identifying current trends and research gaps for future investigations, b) proposes an original Bayesian framework to combine probability and non-probability online surveys in a manner that improves analytic inference while also reducing survey costs, and c) establishes a modular framework that allows for building composite smart indicators in order to augment the information available in traditional sources through digital trace data. The added value of this work lies in its presentation of diverse perspectives and case studies on data integration, showcasing how it can provide enhanced statistical analysis. |
1:15pm - 2:30pm | Lunch Break Location: Cafeteria (Room 0.15) |
2:30pm - 3:30pm | P 1.1: Postersession Location: Auditorium (Room 0.09/0.10/0.11) |
|
Fear in the Digital Age – How Nomophobia together with FoMO and extensive smartphone use lowers social and psychological wellbeing Rheinische Fachhochschule gGmbH (RFH), Germany Relevance & Research Question While FoMO (Fear of Missing Out) is already well known as an important factor that leads to extensive smartphone use (ESU) and lowers wellbeing (WB), research starts to look at the new phenomenon Nomophobia (the fear of being separated from one’s smartphone and not being connected and reachable, e.g. Yildirim & Correia, 2015). However, it still remains unclear, how Nomophobia lowers wellbeing – social as well as psychological wellbeing – over and above the already known factors FoMO and ESU. Methods & Data This study (ad-hoc-sample: N=132) combines all factors in one design and investigates to what extend Nomophobia (measured by: NMP-Q-D Coenen & Görlich, 2022) is an additional factor that causes negative effects on wellbeing (measured by: FAHW, Wydra, 2020) over and above FoMO (measured by FoMO, Spitzer, 2015) as well as ESU (measured by: SAS-SV, Randler et al., 2016). Several regression analyses calculated the effect sizes for the main effects as well as the interaction effects for the different factors – controlled for age and gender. Results Interestingly, different effects can be found regarding psychological wellbeing compared to social wellbeing. Whereas ESU (beta=-.31, p<.01) but not nomophobia lowered the psychological wellbeing quite a lot, instead Nomophobia (beta=-.18, p<.10) but not ESU lowered the social wellbeing significantly. FoMO was similarly a negative factor for psychological (beta=-.22, p<.05) as well as social wellbeing (beta=-.21, p<.05). Interaction effects between all of these factors were tested but could not be found. All in all, quite a part of the variance can be explained only by these three factors: 16% of the variance of psychological wellbeing and 12% of the variance of social wellbeing. Added Value This study extends the knowledge about the factors that causes negative effects on the wellbeing of people in the digital age. Smartphones are so prominent and important nowadays that the fear of losing them can cause additional harm. The results show, that they serve as an important connection tool for social relationships, losing them creates stress and their exorbitant use lowers the wellbeing of people. Is less really more? The Impact of Survey Frequency on Participation and Response Behaviour in an Online Panel Survey German Centre for Higher Education Research and Science Studies (DZHW), Germany Relevance & Research Question Online surveys offer the possibility of interviewing participants of a panel more frequently at reasonable costs. A higher contact frequency might thereby lead to a lower rate of unsuccessful contact attempts through increased bonding with the respondents and address maintenance. If life history data is collected, a higher survey frequency also offers the advantage of shorter reporting periods and decreased time lag for the retrospective collection of these data (Haunberger 2010). This should reduce recall errors and the cognitive burden for respondents. Nevertheless, more frequent interviews also increase the response burden or survey fatigue and could thus lead to a reduced willingness to participate (Haunberger 2010; Schnauber and Daschmann 2016; Stocké and Langfeldt 2003; Nederhof 1986). Until now there is insufficient empirical evidence for survey makers to decide on an optimal design when implementing online panel surveys (see most recently Zabel 1998 for very short wave intervals). Furthermore, existing evidence on survey frequency is limited to CATI and face to face interviews, constraining the validity of possible conclusions about online surveys. We are therefore analysing how the response rate changes when the survey frequency in an online survey is increased. Methods & Data To examine the effect of the survey frequency we implemented an experiment in a panel of secondary school graduates that surveys respondents every two years. To vary the survey frequency, an additional wave was conducted one year after the second wave for a random sample of participants. Both, control and treatment group, were interviewed again two years after the second wave. We compare response rates between these two groups in the latest wave. Results We find a minimally higher response rate with a biennial survey – but without statistical significance. Thus, with a higher expected data quality, no (significant) losses in terms of response seem to be expected if the survey frequency is increased from biennial to annual. Added Value Our results serve as a guideline for survey makers on how to implement online panel surveys aiming for the sweet spot between optimized contact strategies, response burden, and high quality online panel data. |
2:30pm - 3:30pm | P 1.2: Postersession Location: Auditorium (Room 0.09/0.10/0.11) |
|
Digitalisation: Catalyzing the Transition to a Circular Economy in Ukraine Centre for Advanced Internet Studies, Germany Relevance & Research Question Digitalization can contribute to the shift towards a sustainable circular economy (CE). Digitalization not only refines business processes but also emphasizes waste curtailment, prolonging product life, and slashing transaction costs. However, fully leveraging this integration presents challenges, with clear gaps hindering the fluid adoption of digital-backed circular business models. Despite its significance, there's a dearth of comprehensive literature on digitalization's potential and challenges. This research aims to explore the main benefits and obstacles of applying digitalization in CE business models in Ukraine, focusing on identifying opportunities and challenges and finding ways to overcome these hurdles. Methods & Data The study involved interviews with business representatives, researchers, NGOs, and students (a total of 36 participants) during the thematic training course according to the Erasmus+ program in an online format in Ukraine. One of the activities during the training course was finding answers to four questions relevant to the research aim in small groups for 40 minutes for 7-8 participants. Results I identified challenges related to the integration of circular principles into existing business models, data ownership, data sharing, data integration, collaboration, and competence requirements. The post-war rebuilding and modernizing of industries towards sustainability, visualization, and innovation in product design, enhancement in resource efficiency, and optimization of logistics process collaboration with stakeholders and implementation of digital technologies were noted as main opportunities in adopting business models based on CE in the Ukrainian perspective. Added Value This research uncovered less recognized or previously unexplored prospects linked to digitalization in the context of transitioning to a CE. One of the new opportunities is virtualization in business models can influence on reduce costs, conserve resources, and provide reliable data. The research underscores the significant role of digitalization in enabling the transition towards a circular economy in Ukraine's business sector. While there are considerable opportunities for innovation and modernization, the challenges of integration, collaboration, data management, and skill gaps cannot be overlooked. Addressing these challenges through targeted educational programs, strategic partnerships, and supportive policies will be pivotal in harnessing the full potential of digitalization in advancing circular economy models. Device use in a face-to-face recruited neighborhood survey. University of Groningen, Netherlands, The Relevance & Research Question Due to the overall presence of smartphones and the ease of use of these devices, understanding the impact of device choice on survey data quality is becoming increasingly important. This study delves into the intricacies of a community survey conducted through both a paper flyer in the mail box and face-to-face recruitment by students. The primary objective is to explore the correlation between demographic characteristics and the selection of devices for survey completion. Additionally, the study investigates variations in data quality, measured through completion time and response patterns such as straightlining, acquiescence bias, and midpoint responding. Methods & Data The target population consisted of all 5475 residents of a neighborhood in Groningen, living in 4035 households. In December 2023, a total of 3500 flyers were distributed to every address that was recognized as a home address with a separate mail box. Subsequently, students visited homes, encouraging residents to participate in the survey. Students referred to the flyer delivered in the mail box, but presented residents with a new flyer in case the flyer got lost. Participants were given the option to engage via a QR code (i.e., completion on a smartphone) or a concise URL (i.e., completion on a pc), with a sweet incentive of a cake as compensation for their contribution. Results Within two weeks, 605 residents completed the questionnaire, resulting in a response rate of 17%. Notably, the QR code emerged as the preferred method for survey completion, with 85% opting for it, while the URL accounted for 15%. Interestingly, both students and individuals aged over 65 demonstrated a higher likelihood of using the URL. However, no significant associations were uncovered between completion time and the type of device chosen for survey participation. Added Value This study boasts a unique inclusion of all addresses within a single neighborhood in the recruitment sample, transforming it into a comprehensive population survey. In addition, in this study door-to-door recruitment and use of flyers that respondents use to decide their type of device distinguishes this study from earlier work. |
2:30pm - 3:30pm | P 1.3: Postersession Location: Auditorium (Room 0.09/0.10/0.11) |
|
Long Term Attrition and Sample Composition Over Time: 11 Years of the German Internet Panel University of Mannheim, Germany Relevance & Research Question For longitudinal research and panel practitioners, it is important to understand how much attrition to expect over time and which groups of respondents are especially at risk. These insights aid in guiding researchers in determining how many respondents to recruit, when to refresh the sample and which respondents should be especially targeted with strategies for improving recruitment rates or reducing attrition. SampcompR: A new R-Package for Sample Comparisons and Bias Analyses GESIS - Leibnitz Institute for Social Sciences, Germany Relevance & Research Question The steady trend in declining response rates and the rise of non-probability surveys makes it increasingly important to conduct nonresponse and selection bias analyses for social science surveys or conduct robustness checks to evaluate if the results are robust across population subgroups. Although this is important for any research project, it can be very time-consuming. The new R-Package SampcompR was created to provide easy-to-apply functions for those analyses and make it easier for any researcher to compare their survey against benchmark data for bias estimation on a univariate, bivariate, and multivariate level. Methods & Data To illustrate the functions of the package, we compare three web surveys conducted in Africa in March 2023 using Meta advertisements as a recruitment method (Ghana n = 527, Kenya n = 2,843, and South Africa n =313) to benchmarks from the cross-national Demographics and Health Survey (DHS). The benchmarks will be socio-demographics and health-related variables such as HIV knowledge. In univariate comparison, bias is measured as the relative bias for every variable and, on an aggregated level, the average absolute relative bias (AARB). In bivariate estimation, we compare Pearson’s r values against each other, and in multivariate comparison, different regression models are compared against each other. Results Our poster will show examples of output from the package, including visualizations and tables for each comparison level. While the focus will be on figures, tables can also be useful for documentation and more detailed inspection. As to the specific content of our example, we will see that the social media surveys show a high amount of bias on a univariate level. In contrast, the bias is less pronounced on a bivariate or multivariate level. We will also report country differences in sample accuracy. Added Value Our R-Package will provide an easy-to-use toolkit to perform bias analyses and survey comparisons and, therefore, will be a valuable tool in the social research workflow. Using the same or similar procedures and visualizations for the various comparisons will increase comparability and standardization. The visualization is based on the commonly used R-package ggplot2, making it easily customizable.
|
2:30pm - 3:30pm | P 1.4: Postersession Location: Auditorium (Room 0.09/0.10/0.11) |
|
Ask a Llama - Creating variance in synthetic survey data GESIS-Leibniz-Institut für Sozialwissenschaften in Mannheim, Germany Relevance & Research Question: Recently there has been a growth of research on whether Large Language Models (LLM) can be a source for high quality synthetic survey data. However, research has shown that synthetic survey data produced by LLMs underestimates the variational and correlational patterns that exist in human data. Additionally, the process of creating synthetic survey data with LLMs inherently has a lot of researcher’s degrees of freedom which can impact the distribution of the synthetic survey data. In this study we assess the problem of underestimated (co-)variance by systematically varying three factors and observe their impact on synthetic survey data: (1) The number and type of covariates a LLM sees before answering a question, (2) the model used to create the synthetic survey data and (3) the way we extract responses from the model. Methods & Data: We use five socio-demographic background questions and seven substantive questions from the 2018 German General Social Survey as covariates to have the LLM predict one substantive outcome, the satisfaction of the respondent with the government. To predict responses to the target question we use LLama2 in its chat and non-chat variant, as well as two versions finetuned on German text data to control for differences between LLMs. Results: First results show that the (co-)variance in synthetic survey data changes depending on (1) the type and quantity of covariates the model sees, (2) the model used to generate the responses and (3) whether we simulate from the model implied probability distribution or only look at the most likely response option. Especially (3), simulating from the model implied probability distribution, improves the estimation of standard deviations. Covariances estimates, however, remain underestimated. Added Value: We add value in three ways: (1) We provide information on which factors impact variance in synthetic survey data. (2) By creating German synthetic survey data, we can compare findings with results from research that has mostly focused on survey data from the US. (3) We show that using open-source LLMs enables researchers to obtain more information from the models than relying on closed-source APIs. To Share or Not to Share? Analyzing Survey Responses on Smartphone Sensor Data Sharing through Text Mining. Statistics Netherlands, Netherlands, The Relevance & Research Question In 2019, Statistics Netherlands (CBS) conducted the consent survey, inviting respondents to share various types of smartphone data, including location, personal photos and videos, and purchase receipts. The survey particularly focused on understanding the reasons behind the reluctance to share this data. This study explores the following research question: What classifications of motivations and sentiments can be identified for unwillingness to share data with CBS, using a data-driven text mining approach? Methods & Data This research applies multiple text mining techniques to detect underlying sentiments and motivations for not sharing sensor measurements with CBS. The manually classified responses from the survey serve as valuable training and test data for our text mining algorithms. Our findings provide a comprehensive comparison and validation of manual and automated classification methods, offering insights into the effectiveness of text mining. Added Value The study underscores the potential of text mining as an additional tool for analyzing open-text responses in survey research. By using this technique, we detect sentiments and motivations, enhancing the understanding of respondents’ perspectives on data sharing. This approach not only contributes to applying text mining in understanding attitudes towards data privacy and consent, but expands the methodology of survey research for analyzing open-ended questions and text data in general. |
2:30pm - 3:30pm | P 1.5: Postersession Location: Auditorium (Room 0.09/0.10/0.11) |
|
The AI Reviewer: Exploring the Potential of Large Language Models in Scientific Research Evaluation Bielefeld University, Germany Relevance & Research Question The advent of large language models (LLMs) has introduced the potential to automate routine tasks across various professions, including the academic field. This case study explores the feasibility of employing LLMs to reduce the workload of researchers by performing simple scientific review tasks. Specifically, it addresses the question: Can LLMs complete simple reviewer tasks to the same degree as real researchers? Methods & Data We utilized original text data from abstracts submitted to the GOR 2024 conference, along with multiple reviewer assessments (i.e., numeric scores) for each abstract. In addition, we used ChatGPT 4 to generate several AI reviewer scores for each abstract. The ChatGPT model was specifically instructed to mimic the GOR conference review criteria applied by the scientific reviewers, focusing on the quality of research, relevance to the scientific field, and alignment with the conference’s focus. This approach allows us to compare multiple AI assessments with multiple peer-review assessments for each abstract. Results Our results indicate that ChatGPT can quickly and comprehensively evaluate conference abstracts, with ratings slightly higher, i.e. on average more positive, than those of academic reviewers, while retaining a similar variance. Added Value This case study contributes to the ongoing discourse on the integration of AI in academic workflows by demonstrating that LLMs, like ChatGPT, can potentially reduce the burden on researchers and organizers when handling a large set of scientific contributions. Can socially desirable responding be reduced with unipolar response scales? University of Iceland, Iceland Relevance & Research Question It is well-known that the presentation and length of response scales can affect responses to questionnaire items. However, less is known about how different response scales affect responses and what the possible underlying mechanisms are. The purpose of this study was to compare bipolar and unipolar scales using a measure of personality (HEXACO-60) with regard to changes is response distributions, social desirability and acquiescence.
Methods & Data Four versions of the HEXACO-60 personality questionnaire were administered online via MTurk to 1,000 participants, randomly assigned to one of four groups, each containing one of the four versions. The first group received the HEXACO with its original response options (a five-point bipolar response scale), the second group received the HEXACO with a five-point unipolar agreement response scale, and the third group also received a unipolar agreement response scale but with three response options (the original response scale without the disagree response options). The fourth group was asked to rate the social desirability scale value (SDSV) of each of the 60 HEXACO items on a seven-point response scale (from very undesirable to very desirable). An index of item desirability was created from the SDSV and a measure of acquiescence was created by selecting items with incompatible content from the HEXACO to produce item pairs where agreement to both items would indicate acquiescence.
Results The three versions of the HEXACO-60 were analyzed with regard to distributions, social desirability of item content and acquiescence. The results show differences in the distribution of responses between the three response scales. Compared to the bipolar scale, the unipolar scales increased agreement with items rated as undesirable, which would indicate less socially desirable responding on unipolar scales. However, the use of unipolar scales increased overall agreement to items, which could indicate either increased acquiescence or different interpretations of the question in relation to the response options. The results and possible interpretations will be discussed. Added Value The study provides added understanding of the effects of changing response scales from bipolar to unipolar and aids in understanding of the mechanisms underlying responses.
|
3:30pm - 3:45pm | Break |
3:45pm - 4:45pm | A3.1: Solutions for Survey Nonresponse Location: Seminar 1 (Room 1.01) Session Chair: Oriol J. Bosch, University of Oxford, United Kingdom |
|
Does detailed information on IT-literacy help to explain nonresponse and design nonresponse adjustment weights in a probability-based online panel? 1GESIS, Germany; 2University of Bern Relevance & Research Question The generalizability of inference from online panels is still challenged by the digital divide. Newer research concludes that not only individuals who do not have Internet access are under-represented in online panels but also those who do not feel IT-literate enough to participate which is potentially leading to nonresponse bias. Weighting methods can be used to reduce bias from nonresponse if they include characteristics that are both correlated to nonresponse and the variable(s) of interest. In our study we assess the potential of asking nonrespondents about their IT-literacy in a nonresponse follow-up questionnaire on improving nonresponse weighting and reducing bias. Our research questions are: 1.) Does including information on IT-literacy collected in the recruitment survey improve nonresponse models for online panel participation compared to standard nonresponse models including socio-demographics only? 2.) Does including IT-literacy improve nonresponse adjustment? Methods & Data Data are collected in the 2018 recruitment of a refreshment sample of the probability-based German Internet Panel (GIP). Recruitment was conducted by sending invitation letters for the online panel by postal mail. Sampled individuals who were not willing or able to participate in the recruitment online were asked to fill in a paper-and-pencil questionnaire asking about their IT-literacy. The questionnaire was experimentally fielded in the first invitation or reminder mailings. The control group did not receive a paper questionnaire. Results We find IT-literacy to explain nonresponse to the GIP over and above the standard socio-demographic variables frequently used in nonresponse modeling. Nonresponse weights including measures of IT-literacy are able to reduce bias for variables of interest that are related to IT-literacy. Added Value Online surveys bear the risk of severe bias for any variables of interest that are connected to IT-literacy. Fielding a paper-and-pencil nonresponse follow-up survey asking about IT-literacy can help to improve nonresponse weights and reduce nonresponse bias. Youth Nonresponse in the Understanding Society Survey: Investigating the Impact of Life Events Utrecht University, The Netherlands Relevance & Research Question Survey response rate are declining worldwide, particularly among young individuals. This trend is evident in both cross-sectional and longitudinal surveys, such as Understanding Society, where young people exhibit a higher likelihood of either missing waves or dropping out entirely. This paper aims to explore why young individuals exhibit lower participation rates in Understanding Society. Specifically, we investigate the hypothesis that young people experience more life events such as a change in job, relationship status and a move of house, and it is the occurrence of such life events that are associated with a higher likelihood to not participate in the survey. Methods & Data The data source is Understanding Society, a mixed-mode probability-based general population panel study in the UK. We analyze individuals aged 18-44 at Understanding Society's Wave 1, and we follow them until Wave 12. We consider four age groups: 18-24 (youth), 25-31 (early adulthood), 32-38 (late adulthood) and 39-45 middle age (reference group for comparison). In order to study the effect of life events on attrition, we applied the Discrete-Time Multinomial Hazard Model. In this model the time is entered as a covariate and the outcome variable is the survey participation indicator (interview, noncontact, refusals or other). The outcome is modeled as a function of lagged covariates, including demographics, labor market participation, qualifications, household structure and characteristics, marital status and mobility, as well as binary indicators for life event-related status changes. Consistent with existing literature, our findings reveal that younger respondents, as well as those with an immigration background, lower education, and unemployment status, are less likely to participate. We also demonstrate that changes in job status and relocation contribute particularly to attrition, with age remaining a significant factor. As many household surveys are moving online to save costs, the findings of this study will offer valuable insights for survey organizations. This paper enriches our understanding of youth nonresponse and presents practical strategies for retaining them. This project is funded by the Understanding Society Research Data Fellowship. Exploring incentive preferences in survey participation: How do socio-demographic factors and personal variables influence the choice of incentive? Deutsches Zentrum für Integrations- und Migrationsforschung (DeZIM), Germany Relevance & Research Question Methods & Data Results Added Value |
3:45pm - 4:45pm | A3.2: Survey Instruments Location: Seminar 3 (Room 1.03/1.04) Session Chair: Cornelia Neuert, GESIS Leibniz Institute for the Social Sciences, Germany |
|
Unmapped potentials: Measuring and considering the self-defined residential area of individuals 1Humboldt-Universität zu Berlin; 2DeZIM-Institut, Humboldt-Universität zu Berlin; 3Humboldt-Universität zu Berlin Relevance & Research Question Many research questions would benefit from information about individuals’ self-defined residential area, i.e., where they spend their daily lives. However, the location information collected (or available to researchers given pseudonymization requirements) are typically larger aggregates, such as the postcode area. Even if respondents’ addresses were available, we still would not know where they actually run their errands, go to the doctor, or spend family time on the playground. However, this is the area substantially affecting their lives, and often the area they care about most. We collected data on the self-defined residential areas for research on anti-Muslim racism and examine the determinants of opposition to the opening of new Muslim-read establishments in those areas. Methods & Data As part of a representative online survey in Germany implemented by Kantar (n = 17.500), respondents drew their residential area as a polygon on a map using the open map tools offered by OpenStreetMap, Leaflet, and Leaflet Draw. Besides a wide range of socio-demographic and attitudinal measures, we also asked about several aspects of the self-defined residential area, e.g., the number of mosques, Turkish/Arabic-read restaurants and supermarkets. Moreover, we asked if respondents or their neighbors would oppose new establishments being built/opened. We then merged the actual number of establishments in that area fetched via the Google Places API. Results [preliminary, new results to be expected by Nov 30] Our results show that about 40 percent of respondents drew a plausible residential area (validated by postcode, shape, and size). First analyses indicate that respondents particularly oppose the opening of new Muslim-read establishments if there are none or very few in their residential area (e.g., not a single mosque). Added Value Although still preliminary, it appears that collecting information about respondents’ residential areas works reasonably well in online surveys with tools already available. Once the area information is collected, it is easy to add point-referenced geographical data, e.g., from Google Places or OpenStreetMap, and crosswalks can be used to add data corresponding to other geographical units – all of which may offer valuable additional perspectives. Partnership biographies in self-administered surveys: The effect of screening-in information on survey outcomes GESIS – Leibniz-Institute for the social sciences, Germany Relevance & Research Question Many cross-sectional and panel studies survey retrospective biographical information, covering areas such as educational and occupational careers, fertility biographies, or former intimate relationship experiences. In interview-administered surveys, the use of event history calendars (EHC) has been established by collecting biographical data retrospectively and is evident to reduce recall effects and, thus, improve data quality. However, to handle raising survey costs, recent survey programs are increasingly conducted in self-administered modes, e.g., as web or mail surveys, or combine both in a mixed-mode approach. Additionally, the share of participants using their smartphone to respond to surveys is high and rising in self-administered modes. The lack of an interviewer, as well as displaying questionnaires on small screens impede the use of approved EHCs in self-administered surveys and at the same time increase the need for user-friendly survey tools and less complex questions. Based on data from a survey experiment, we test whether visual feedback in complex survey modules improves the data quality of biographical information, i.e., relationship biographies. We set out to investigate: (1) Is displaying of information from previous questions on partnership(s) related to survey outcomes regarding non-response and interview duration? (2) Does the number of partnerships and the partnership duration vary with the display of information from previous questions? (3) Is the display of information from previous questions related to respondent burden? We run anova models on a sample of 3,446 respondents from a web survey conducted in December 2022. Within this survey, we vary the display of the question list on relationships using information from previous questions on partnerships as visual feedback. As visual feedback, we used the names of respondents’ (ex-)partners, their relationship status, and respective dates. The control group runs through the question lists without visual feedback. Results Preliminary results do not show differences between the experimental and control group regarding non-response and interview duration. However, our results hint at differences in the number of relationships reported and respondents' perceived burden. Added Value Our study adds knowledge on how complex survey modules can be conducted without the presence of an interviewer. Considering Respondents’ Preferences: The Effects of Self-Selecting the Content in Web Survey Questionnaires Universität Wien, Austria Relevance & Research Question Previous research has shown that the willingness to participate in surveys increases with the individual salience of the survey. In practice, this is usually taken into account to the extent that the survey topic and its relevance are presented in invitation letters hoping that it motivates a large and representative group to participate. In this study, we investigate how the willingness to participate in a survey and respondents’ survey satisfaction change when they can choose from different topics. Methods & Data Our exploratory data analysis compares response rate, panel recruitment, and survey experience of 2,735 respondents. Descriptive statistics and Pearson's Chi-square test were used for the analysis. The sample is stratified by region and has been recruited offline from the Austrian Central Population Register. Respondents are randomly assigned to one of two questionnaire designs. In one design, the number and order are predetermined. Respondents assigned to the other design have the flexibility to decide how many topic blocks (modules) they would like to answer and in what order. Amongst others, the analysis examines the number, thematic preferences, and order of the selected modules. We also evaluate respondents' verbal feedback regarding the module selection choice. Results Announcing that respondents can choose among different survey topics does not attract more or different respondents as opposed to an invitation letter to a survey, in which this option is not mentioned. There is also no difference regarding panel recruitment. Yet, the share of respondents being very satisfied with the survey is higher among those, who answer more modules than required for the incentive. Answers to an open-ended feedback question mirrors this satisfaction. While modules on politics are less often selected than others, most respondents choose all six modules. Added Value This study examines the effect of a dynamic online survey design, in which respondents can flexibly select - at least partially - the content of their survey. Although in practice it may not always be possible to adjust the entire survey’s content to any respondent’s preferences, the study highlights advantages and disadvantages of letting respondents choose parts of the survey. |
3:45pm - 4:45pm | B3: The Power of Social Media Data Location: Seminar 2 (Room 1.02) Session Chair: Ádám Stefkovics, HUN-REN Centre for Social Sciences, Hungary |
|
Bridging Survey and Twitter Data: Understanding the Sources of Differences 1University of Michigan, United States of America; 2Georgetown University, United States of America; 3SSRS, United States of America Relevance & Research Question For years, researchers have attempted to use social media data to generate inferences typically produced using surveys. But Twitter data and other social media traces do not consistently reflect contemporary survey findings. Two explanations have been proposed for why this might be the case: one posits that the set of people producing data on social media sites differs from those recruited to surveys; the other asserts that data generating processes are sufficiently different that it does not make sense to compare their social media and survey outputs directly. Methods & Data This study links a probability US sample of survey respondents with those same individuals’ Twitter data as well as with decahose Twitter data. We compare four datasets to understand links between samples and data generating processes. These include survey responses on three topics for (1) a probability sample of the US public (N=9544); (2) the same survey responses for the subset of individuals who use Twitter, consent to access, and tweet about the topics of interest (N=246); (3) tweets for this set of linked individuals who tweeted about the topic of interest; and (4) tweets from US individuals sampled from the Twitter decahose (N=7,363 after removing bots and non-individual accounts). Open-ended survey questions and social media posts are topic modeled using a guided topic modeling approach within topic areas to identify vaccination behaviors/attitudes, economic evaluations, and parenting challenges during the COVID pandemic. We find that the subset of individuals who used Twitter and consented to linkage differed slightly in demographic composition, but mentioned similar distribution subtopics in response to open-ended survey questions about all three areas. In contrast, individuals with survey and Twitter data provided similar data across these two modes for one of our three topics (economics) and different data across the other topics (vaccinations and parenting). Tweets from consented users and the decahose sample, in contrast, provided similar distributions of topics for vaccinations and parenting, but not economics. This suggests that motivation to post and posting frequency may be more important for data acquired than who is represented. Physical Proximity and Digital Connections: The Impact of Geographic Location on Twitter User Interaction 1Bielefeld University; 2ETH Zürich Relevance & Research Question In the context of an online social network where geographical distance is often assumed to be inconsequential, this study examines how physical proximity relates to Twitter user interaction. In line with previous findings, the central hypothesis is that individuals who live in closer physical proximity are more likely to engage with one another, despite the virtual nature of Twitter. Moreover, the extent of this impact is expected to be contingent on the specific topic under discussion. Methods & Data Employing a multi-layered approach, the study integrates techniques from natural language processing, network analysis, and spatial analysis. A dataset of over 500 million geolocated German tweets (including retweets) forms the basis of the analysis. First, a BERT-like language model is trained on the tweets to categorise them into thematically similar groups, enabling a granular exploration of topic-specific interactions. Subsequently, retweet and reply networks are constructed for each thematic group as well as for the entire tweet corpus. Community detection algorithms are then used to identify clusters of users who frequently retweet and reply to each other. Spatial analysis is then applied to examine the correlation between users' physical proximity and their clustering as identified by community detection. Results Preliminary results indicate a corpus-wide positive correlation between the spatial proximity of users and their clustering based on retweet and reply communities. However, the strength and significance of the correlation varies across the different topics discussed within the Twitter dataset. Notably, the geographical aspect of discussions can be found not only among local topics, but also in topics with a more universal appeal. Added Value This study offers a methodologically complex investigation of the interplay between geography and online social networks. By revealing the nuanced relationship between spatial proximity and Twitter user interaction based on topics, the study extends our understanding of online social dynamics. The findings contribute to the broader discourse on social media by highlighting the importance of local context and regional differences as a determinant of online interaction patterns. Gender (self-)portrayal and stereotypes on TikTok 1Bielefeld University, Germany; 2Bielefeld Graduate School in History and Sociology, Bielefeld; 3Institute for Interdisciplinary Research on Conflict and Violence, Bielefeld Relevance & Research Question Women and men are portrayed differently in advertising and on social media, as research on gender (self-)portrayal has shown. Most studies in this area analyzed small samples of static images to examine gender stereotypes conveyed through images on social media. We study gender (self-)portrayal on TikTok, in particular which dynamic expressions are more often used by individuals passing as women or men. For this, we present a novel method to analyze large amounts of video data with computational methods. Methods & Data Our data encompasses approximately 36,000 unique videos extracted from the top 1000 trending TikTok videos in Germany over a consecutive 40-day period in 2021, supplemented by 973,000 metadata entries. Each video is processed using YOLOv8 pose detection, which dissects the videos into frames and annotates 17 key points per frame. We group the data into commonly used dynamic expressions (i.e., sequences of body movement). We employ HDBSCAN and DTW to deal with differences of sequence and video length and to handle ‘valid’ missing data, e.g., from certain body parts not being visible in the footage. Results Sequences are grouped into prototypes of dynamic expressions. Using manually annotated information, we can distinguish certain types of movement that are more commonly used by one gender. Utilizing metadata and expressions in the videos, we are able to explain a part of the variance of how a video performs, i.e. how many likes it gets or how long it stays within the top 1000 trends. A qualitative assessment of the prototypes of the most gender-biased expressions allows for integration with sociological theory on gender stereotypical body posing and provides insight into why some poses might perform better regarding likes and views. Added Value We extend the framework for analyzing gender stereotypical posing from static social media images to dynamic social media videos, which is an important endeavor to adapt to the trend of video-based social media content (Snapchat, TikTok, Instagram reels) becoming the de facto default type of content, especially for younger generations. Regarding methods, we offer a tractable way to analyze body posing on social media. |
3:45pm - 4:45pm | C3: Artificial Intelligence Location: Seminar 4 (Room 1.11) Session Chair: Julia Susanne Weiß, GESIS, Germany |
|
AI: Friend or Foe? Concerns and Willingness to Embrace AI technologies in Israel 1Academic College of Emek Yezreel, Israel; 2Bar-Ilan University, Israel Relevance & Research Question Research on AI has a long history, spanning seven decades (Jiang et al., 2022), but only recently have scholars began exploring AI's impact on everyday activities (Ertal, 2018). Over the last two years, one could witness a surge in the use of large language models like ChatGPT, Bard, and Dall-e2. This study investigates people's concerns about AI replacing their roles and their willingness to embrace these technologies, focusing on traditional predictors of fear and adoption: income, education, and age. Methods & Data A representative survey of the adult (18+) Jewish population in Israel was conducted (n=502) via an internet panel (iPanel) in the beginning of 2023. It was comprised of demographic and perspectives on AI technologies questions. Results Results indicate a significant negative correlation between income, education, and age with fears of AI replacing jobs (rs = -.179, p < .001; rs = -.108, p < .01; rs = -.096, p < .05). Additionally, a borderline significant positive correlation between willingness to adopt AI models and education (rs = .071, p = .055) and a significant negative correlation with age (rs = -.088, p < .05) were found. No correlation was observed between income and the willingness to adopt these technologies (rs = .019, p > .05). Added Value Notably, this research reveals a unique finding; Contrary to previous studies showing negative correlations between fear of technology and income or education, the fear of adopting new technologies is inversely related to age. As people grow older, their fear of adopting technology diminishes, likely because these tools offer a user-friendly interface resembling existing chat bots, requiring no new technological literacy. Another possible explanation is that the respondents feel secure in their workplace positions regardless to the new technologies. Moreover, the lack of a correlation between income and willingness to adopt may stem from the low (sometimes free) cost associated with these technologies. In an era of rapid AI development and integration into daily life, studies like this one hold significance in understanding public sentiments surrounding these tools and their implications for personal and professional life. Human Accuracy in Identifying AI-Generated Content 1HTW Berlin, Germany; 2horizoom GmbH, Germany; 3pangea labs GmbH, Germany Relevance & Research Question: The research addresses a significant question in the era of advanced digital technology: "Are humans ready to detect AI-generated content?" This question is pivotal as it explores human perception and understanding in the face of rapidly evolving AI capabilities in times of deep fakes on all media platforms. Methods & Data: The empirical approach is using a digital interview with n>1000 Germans exposed to a variety of AI-generated and human-created content. In three categories (pictures, audio, videos) the participants were asked to identify the source of each piece of content, whether it was produced by AI or by a human..The content itself was created using AI Tools and stock content sources. The questionnaire is using implicit measurement and pairwise comparisons using the Analytic Hierarchy Process (AHP) methodology. Results: The findings reveal intriguing insights into the human ability to discern AI-generated content. A significant proportion of participants are heavily challenged in correctly identifying the nature of the content, with varying degrees of accuracy across different types of media. These results highlight the sophistication of current AI technology in mimicking human creativity and the challenges faced by individuals in distinguishing between the two. Added Value: This study adds substantial value to the discourse on AI and human interaction. It provides empirical evidence on the current state of human perception regarding AI-generated content in Germany, offering a foundation for further research in this area. The findings have implications for fields ranging from digital media and communication to AI ethics and policy-making, emphasizing the need for increased awareness and understanding of AI capabilities among the general public. Industry study: Experiences, expectations, hopes and challenges of working with AI in qualitative research. KERNWERT, Germany Relevance & Research Question |
3:45pm - 4:45pm | D3: Virtual Respondents and Audiences - Is This the Future of Survey Research? (organised by marktforschung.de) Location: Auditorium (Room 0.09/0.10/0.11) Session Chair: Holger Geissler, marktforschung.de, Germany Panelists: Dirk Held, Co-Founder & Managing Director of DECODE Marketing and Co-Founder of Aimpower Louise Leitsch, Director Research of Appinio Frank Buckler, Founder & CEO of Success Drivers & Supra Tools Florian Kögl, Founder & CEO of ReDem |
4:45pm - 5:00pm | Break |
5:00pm - 6:00pm | A4.1: Innovation in Interviewing & Coding Location: Seminar 1 (Room 1.01) Session Chair: Jessica Donzowa, Max Planck Institute für demographische Forschung, Germany |
|
Exploring effects of life-like virtual interviewers on respondents’ answers in a smartphone survey 1German Center for Higher Education Research and Science Studies (DZHW); 2Leibniz University Hannover; 3University of Michigan; 4GESIS - Leibniz Institute for the Social Sciences Relevance & Research Question API vs. human coder: Comparing the performance of speech-to-text transcription using voice answers from a smartphone survey 1German Center for Higher Education Research and Science Studies (DZHW); 2Leibniz University Hannover; 3GESIS - Leibniz Institute for the Social Sciences Relevance & Research Question Can life-like virtual interviewers increase the response quality of open-ended questions? 1GESIS Leibniz Institute for the Social Sciences, Germany; 2DZHW; Leibniz University Hannover Relevance & Research Question Open-ended questions in web surveys suffer from lower data quality compared to in-person interviews, resulting in the risk of not obtaining sufficient information to answer the research question. Emerging innovations in technology and artificial intelligence (AI) make it possible to enhance the survey experience for respondents and to get closer to face-to-face interactions in web surveys. Building on these innovations, we explore the use of life-like virtual interviewers as a design aspect in web surveys that might motivate respondents and thereby improve the quality of the responses. We investigate the question of whether a virtual interviewer can help to increase the response quality of open-ended questions. Methods & Data In a between-subjects design, we randomly assign respondents to four virtual interviewers and a control group without an interviewer. The interviewers vary with regard to gender and visual appearance (smart casual vs. business casual). We compare respondents’ answers to two open-ended questions embedded in a smartphone web survey with participants of an online access panel in Germany (n=2,000). Results The web survey will run in November 2023. After data collection, we analyze responses to the open-ended questions based on various response quality indicators (i.e., probe nonresponse, number of words, number of topics, response times). Added ValueThe study provides information on the value of implementing virtual interviewers in web surveys to improve respondents experience and data quality, particularly for open-ended questions. |
5:00pm - 6:00pm | A4.2: Data Quality Assessments 1 Location: Seminar 3 (Room 1.03/1.04) Session Chair: Patricia Hadler, GESIS - Leibniz Institute for the Social Sciences, Germany |
|
Data Quality in a Long and Complex Online-Only Survey: The UK Generations and Gender Survey (GGS) University of Southampton Relevance & Research Question Long surveys present high burden for respondents. For a long time the rule of thumb for length of self-completion surveys was not to exceed 15-20 minutes. More surveys are moving towards self-completion designs due to increasing survey costs and also due to high rates of device ownership and internet access in the UK and other countries. For some social surveys 20 minutes is not enough to continue collecting high quality data required. Some studies experimented with longer questionnaires and obtained reassuring results, for example, European Social Survey (ESS). However, more evidence is needed in this under-researched area. We collected the first wave of the UK Generations and Gender Survey (GGS) where only online mode of data collection was available to respondents. The median time spent on the questionnaire is around 40 minutes which is much higher than the advice given to survey practitioners in the past. It is important to assess different aspects of data quality of the long questionnaire. The main research question is: is long questionnaire associated with poorer data quality?
We analyse the GGS collected in the UK. The GGS is a part of a global data collection infrastructure focused on population and family dynamics. The GGS collects demographic, economic, and social attitude data on young and mid-life adults (18-59) as they enter into adulthood, form partnerships, and raise children. We assess different data quality indicators: break-off rate, item nonresponse, different response style behaviours, consent to participation in the second wave of the survey among other indicators. We first conduct descriptive analysis and then use different logistic regression models to investigate data quality in the UK GGS.
The results are reassuring and suggest that even though the GGS questionnaire is long and complex and interviewers are not there to guide the respondents through the process, the data quality is not poor. Screening the Screens: Comparing Sample Profiles and Data Quality between PC and Mobile Respondents 1National Centre for Social Research, United Kingdom; 2European Social Survey Headquarters - City, University of London Relevance & Research Question: In an era where smartphones have become ubiquitous, the use of mobile devices for survey completion has become prevalent, underscoring the need to understand their impact on data quality. Long gone are instructions for respondents to use alternative devices. While earlier research suggested that mobile device responses resulted in lower data quality, recent studies based on mobile-first survey designs challenge this view, indicating that smartphone usage does not inherently degrade data quality. Methods & Data: This study contributes to this evolving body of research by examining smartphone survey completion in CRONOS-2, the ESS probability-based panel fielded across 12 European countries from November 2021 to March 2023. Results: We investigate response patterns over time and analyse demographic differences between smartphone and PC respondents, discussing how these insights might be used for targeted respondent recruitment. In addition, we explore survey completion times and data quality indicators, including item non-response and satisficing behaviors, drawing comparisons between PC and smartphone respondents. In this study, we also examine potential differences in break-off rates between smartphone and PC respondents. This approach is informed by previous research, which suggests that smartphones might lead to higher break-offs. Should this be the case, our goal is to identify any problematic items that could lead to such outcomes. Added Value: The findings from our research are intended to assist survey researchers and practitioners in the design and execution of high-quality online surveys in a mobile-centric world. Exploring Device Differences: Analyzing Sample Composition and Data Quality in a Large-Scale Survey GESIS – Leibniz Institute for the Social Sciences, Germany Relevance & Research Question More and more large-scale surveys offer web mode as part of a mixed-mode design or as the only mode. Respondents can access the web survey from various devices including desktop computer, tablet, and smartphones. The proportion of respondents using smartphones to complete web surveys is growing. Some studies indicated that the breakoff rate is higher for respondents completing on a smartphone than on other devices. Especially in the context of large-scale social surveys with long completion times, this could lead to more breakoffs at an early stage of the survey. There is also evidence that other data quality indicators vary across devices. Those variations may increase for questions asked later in a survey, as respondents experience a greater burden toward the end of a survey. However, different devices may also address different individuals, which would result in a more balanced sample. We investigate the impact of the growing use of smartphones in large-scale surveys. Methods & Data We use data from the German General Social Survey (ALLBUS) 2021 and 2023. ALLBUS is a register-based cross-sectional survey of persons aged 18 and older in Germany with a completion time of around 50 minutes. Both surveys were conducted in a self-administered mixed-mode design (mail and web). We compare the sample composition and data quality between different devices. The data quality includes breakoffs, the final question before breakoff, item nonresponse, completion time, and providing an answer to an open question. Results The proportion of smartphones used to participate in the survey increases from 5.7% in ALLBUS 2021 to 17.3% in ALLBUS 2023. Preliminary results show that smartphone users restart the survey more often than desktop users. Moreover, breakoff occurs earlier and at a greater rate for smartphone users compared to desktop users. There are significant socio-demographic differences between smartphone and desktop users. Added Value Examining differences in devices improves our understanding of how respondents behave and the potential impact on data quality, especially in the later stages of large-scale surveys.
|
5:00pm - 6:00pm | B4: Willingness to participate in passive data collection studies Location: Seminar 2 (Room 1.02) Session Chair: Johannes Volk, Destatis - Federal Statistical Office Germany, Germany |
|
The influence of conditional and unconditional incentives on the willingness to participate in web tracking studies GESIS Leibniz Institute for the Social Sciences, Germany Relevance & Research Question Linking web tracking and survey data opens new research areas. We record participants browsing behavior via a browser plugin. Tracking allows for measuring behavior that individuals tend to recall inaccurately and reduces the survey burden. However, previous studies found that participants were reluctant to participate in tracking studies. To increase participation rates, monetary incentives are widely used. These can be granted unconditionally, conditional on participation or as a combination of both. It is, however, unclear (1) how large conditional incentives should be and whether unconditional incentives can additionally increase participation rates. Additionally, we are interested in, (2) whether these effects are the same for a convenience sample and a probability-based sample. Methods & Data To answer our research questions, we conduct a 2x3 factorial experiment with approximately 2600 panelists of a new panel. Panelists are recruited via Meta-ads and via a German general population survey (ALLBUS). The first factor is whether panelists receive a prepaid incentive of 5 Euro or not. The second factor is the amount of the postpaid incentive (10,25 or 40 Euro), conditional on 30 out of 60 active days in the tracking period. We investigate (1a) consent for participation in a web tracking study, (1b) actual installation of the browser plugin. We will present logistic regression models. Additionally, (2) we will investigate the differences between Meta and ALLBUS recruited participants. Results Using a smaller dataset of our first field period including only participants recruited via Meta-ads, we find that the unconditional incentive has a positive relation with consent but not with installation. For the amount of the conditional incentive, we cannot see an effect yet. We will analyze a larger dataset for the results that will be presented at the conference. Results for our second research question will be available by the time of the conference. Added Value Little is known on how incentives and other factors impact participation in tracking studies as most research only investigates hypothetical consent. Our study adds to the knowledge on the incentive amount needed to recruit participants via Meta and via a general population survey into a web tracking study. Intentions vs. Reality. Validating Willingness to Participate Measures in Vignette Experiments Using Real-World Participation Data 1HUN-REN Centre for Social Sciences, Hungary; 2IQSS, Harvard University; 3Századvég Foundation; 4Eötvös Loránd University Relevance & Research Question: Vignette and conjoint experiments are extensively utilised in the field of social sciences. These methodologies assess preferences in hypothetical scenarios, exploring decision-making in complex choices with varied attributes, aiming to align survey responses more closely with real-world decisions. However, survey experiments are only valid externally to the extent that stated intentions align with real-world behaviour. This study uses a unique dataset that allows us to compare the outcomes of a vignette experiment (which assessed willingness to participate in a social media data donation study) to actual participation in a real data donation study involving the same survey respondents. Methods & Data: A vignette experiment embedded in an online survey of a non-probability-based panel was conducted in Hungary in May 2022 (n=1000). Respondents expressed their willingness to participate in hypothetical data donation studies. In a mixed factorial design, five treatment dimensions were varied in the study descriptions (platform, range of data, upload/download time, monetary-, and non-monetary incentive). In February 2023, the same participants were invited to a real data donation study which had almost the same characteristics as the ones described in the vignettes. Results: The correlation between the self-reported willingness and actual participation was only 0.29. Moreover, the drivers of willingness and actual participation were different. For instance, education was one of the strongest predictors of willingness, yet was not significantly associated with actual participation. We also found differences regarding the effect of privacy beliefs or the Big-Five personality traits. Added Value: This study contributed to the literature by validating the results of a vignette experiment using within-person comparisons from behavioural data. The results suggest that vignette experiments may strongly suffer from hypothetical or other biases, at least in scenarios when the personal risk and burden are high, and underscore the importance of improving external validity of such experiments. Who is willing to participate in an app- or web-based travel diary study? 1Utrecht University; 2Statistics Netherlands Relevance & Research Question Using apps as a survey mode offers promising features. The use of passive measurements on smartphones can be beneficial for response burden, by replacing traditional survey questions, and for data quality as it can reduce recall bias. However, not everyone is able or willing to participate in an app-based study, causing coverage issues and nonresponse. We investigate whether a mixed-mode design can be effective for our goals by analyzing who chooses to participate in an app study and who prefers a web questionnaire. Methods & Data We report on a study by Statistics Netherlands (winter 2022-2023) for which we invited 2544 individuals from a cross-sectional sample of the Dutch population. We asked individuals to use a smartphone app to collect their travel data, or participate in a web questionnaire. We combine a concurrent mixed-mode design with a “push-to-app” design by offering the web questionnaire at different moments: directly in the invitation letter or in one of the reminders. Invitees are asked to participate in one mode. We assess whether participation is related to individual characteristics with registry data. Results More people register in the app (11.5%) than in the questionnaire (7.0%). Total registration rates are higher when the web questionnaire is offered directly (19.8%) than in the first (18.5%) or second reminder (15.8%). The app registration rate does not increase much when the web questionnaire is offered later, suggesting that certain people have a mode-preference for the app. Most striking is the age effect. The app attracts younger participants while older participants are overrepresented in the web questionnaire. Combining the two yields a more balanced sample. Added Value We show that with a mixed-mode design, we can attract more respondents than with an app-only design in a probability based sample. With the use of population registries we are able to improve our understanding of who participates in app- and web-studies. Additionally, our analysis can contribute to the design of future diary studies combining a smartphone app and a web questionnaire. |
5:00pm - 6:00pm | C4: Political Communication and Social Media Location: Seminar 4 (Room 1.11) Session Chair: Josef Hartmann, Verian (formerly Kantar Public), Germany |
|
Mapping news sharing on Twitter: A bottom-up approach based on network embeddings 1Weizenbaum-Institut e.V., Germany; 2Max-Planck-Institut für Mathematik in den Naturwissenschaften, Germany; 3Sciences Po, médialab, Paris, France Relevance & Research Question We combine multiple data sources via state-of-the-art network embedding methods and automated text analysis: - we collected all tweets which contained a link to one of 26 legacy of alternative news outlets for March/2023 (2.5M tweets). Results Individual-level and party-level factors of German MPs’ general and migration-related political communication in parliament and on Facebook between 2013 and 2017 Hertie School, Germany Relevance & Research Question Facebook allows for direct communication with voters in the electorates. An issue that is divisive or polarizing on social media and political discourse is migration. This raises the guiding research question, of whether MPs who have positive or negative attitudes toward migration are more likely to speak in parliament on the issue or post about it on Facebook. This study compares the classical form of political speeches in parliament with social media communication on Facebook by members of parliament of the 18th German Bundestag (2013-2017). While prior studies compared political speech in parliamentary speeches and on social media focused on Twitter messages, this study uses a unique data set linking parliamentary speeches with election data, a candidate survey (GLES), and MPs’ social media communication on Facebook. The linked data allows to control for a number of candidate characteristics and test the influence of party or migration-attitudes on speaking and posting behaviour. The first part of the analysis examines factors associated with general political communication activity in parliament and on Facebook and deploys a generalized linear quasi-Poisson mode, whilst the second part identifies migration-related speeches and posting using a dictionary approach and also analyses the association with candidate characteristics in a quasi-Poisson model. Results The first part of the analysis finds that party differences and candidacy play a role in speech activity, whereas being from a ’left-centrist’ party (DIE LINKE, SPD, GRÜNE) is positively associated with the number of Facebook messages issued by MPs. The second part focuses on migration-related communication activity. Against the expectation that MPs with negative migration stances might have used Facebook more intensively to post about migration, the findings indicate that MPs who are in favour of migration were more likely to speak about migration-related issues in parliament and post about it on Facebook. |
5:00pm - 6:00pm | D4: Wissenschaft trifft Praxis. Wann ist eine Online-Stichprobe gut für welchen Bedarf. Location: Auditorium (Room 0.09/0.10/0.11) Session Chair: Otto Hellwig, Bilendi & respondi, Germany Impulse von: Dr. Carina Cornesse (German Institute for Economic Research, Germany & DGOF Vorstand) Menno Smid (Vorstandsvorsitzender (CEO) bei Infas Holding AG) Weitere Diskutanten: Beate Waibel-Flanz (Business Insights - Market research Manager bei REWE GROUP & stellv. Sprecherin des BVM Regionalrates) Dr. Barbara Felderer (Teamleiterin Survey Design & Methodology Survey Statistics bei Gesis) |
|
Wann ist eine Stichprobe “fit-for-purpose”? German Institute for Economic Research, Germany In den Medien werden immer wieder Fälle von Studienerkenntnissen diskutiert, die sich bei genauerer Betrachtung als falsch herausstellen. Nicht selten ist der Grund die Datenbasis, welche die proklamierten Schlüsse aufgrund der Selektivität der Stichprobe nicht zulässt. Dies betrifft in besonderem Maße Studien, die von nicht-zufallsbasierten Online-Stichproben auf die deutsche Gesamtbevölkerung schließen wollen. Diese Rückschlüsse beruhen in der Regel auf Annahmen, die weder explizit kommuniziert werden, noch in vielen Fällen haltbar sind. Die Stichproben stellen sich dann also als nicht geeignet für den Zweck heraus, den sie erfüllen sollen und sind somit nicht “fit-for-purpose”. Dieser Impulsvortrag beschreibt die Annahmen, die Inferenzschlüssen basierend auf Nicht-Zufallsstichproben zugrunde liegen und diskutiert Umstände, unter denen diese Annahmen halten können. Der Fokus liegt dabei auf der Frage, ob (und wenn ja wann) eine (hoch)selektive nicht-probabilistische Online-Stichprobe fitness-for-purpose für einen bestimmten Forschungszweck aufweisen kann. |
8:00pm - 11:00pm | GOR 24 Party Location: Mach Bar - Zülpicher Str. 40, 50674 Köln |
Date: Friday, 23/Feb/2024 | |
9:30am - 10:00am | Begin Check-in |
10:00am - 10:45am | Keynote 2: Keynote 2 Location: Auditorium (Room 0.09/0.10/0.11) |
|
Data collection using mobile apps: What can we do to increase participation? University of Essex, United Kingdom There are limits to what can be measured with survey questions: we can only collect information about things our respondents know, can recall, are willing to tell us – and that fit within a time-constrained questionnaire. Increases in smartphone ownership and use, along with technological changes are creating new possibilities to collect data for surveys of the general population, for example, through linkage or donation of existing digital data, collection of bio-samples or -measures, or use of sensors and trackers. Surveys are therefore developing into systems of data collection: depending on the concept of interest, different methods are used to generate data of the required level of accuracy, granularity, and periodicity. For example, Understanding Society: the UK Household Longitudinal Study supplements the annual questionnaire-based data with linked data and data derived from bio measures and bio samples. In addition, we are developing and testing protocols to collect data using mobile applications, activity and GPS trackers and air quality sensors. We have conducted a series of mobile app studies, collecting detailed information about household expenditure, daily data about relationships, stressors and wellbeing, detailed body measurements, and spatial cognition. However, in each case, only a sub-set of respondents invited to the mobile app study participated and provided data. In this talk I will present research from a series of experimental studies carried out on the Understanding Society Innovation Panel, that aim to identify the barriers faced by respondents in participating in mobile app studies, provide evidence on how best to design data collection protocols to maximise participation and reduce selectiveness of participants, and examine the quality of data collected with mobile apps. |
10:45am - 11:15am | GOR Award Ceremony |
11:15am - 11:45am | Break |
11:45am | Track A.1: Survey Research: Advancements in Online and Mobile Web Surveys sponsored by GESIS – Leibniz-Institut für Sozialwissenschaften |
11:45am | Track A.2: Survey Research: Advancements in Online and Mobile Web Surveys sponsored by GESIS – Leibniz-Institut für Sozialwissenschaften |
11:45am | Track B: Data Science: From Big Data to Smart Data |
11:45am | Track C: Politics, Public Opinion, and Communication |
11:45am | Track D: Digital Methods in Applied Research |
11:45am - 12:45pm | A5.1: Recruiting Survey Participants Location: Seminar 1 (Room 1.01) Session Chair: Olga Maslovskaya, University of Southampton, United Kingdom |
|
Recruiting online panel through face-to-face and push-to-web surveys. HUN-REN Centre for Social Sciences, Hungary Relevance & Research Question: This presentation focuses on the difficulties and solutions related to recruiting web panels through probability-based face-to-face and push-to-web surveys. It also compares the panel composition when using two different survey modes for recruitment. Methods & Data: As part of the ESS SUSTAIN-2 project, a webpanel was recruited in 2021/22 through a face-to-face survey of ESS R10 in 12 countries. Unfortunately, the recruitment rate was low and the sample size achieved in Hungary was inadequate for further analysis. To increase the size of the webpanel (CRONOS-2), the Hungarian team initiated a probability-based mixed-mode self-completion survey (push-to-web design). Respondents were sent a post inviting them to go online or complete a questionnaire, which was identical to the interviewer-assisted ESS R10 survey. Results: We will present our findings on how the type of survey affects recruitment to a web panel through probability sampling. We will begin by introducing the design of the two surveys, then discuss the challenges encountered in setting up the panel, and finally compare the composition of the panel recruited through the two surveys (interviewer-assisted ESS R10 and push-to-web survey with self-completion). Our research provides valuable insight into how the type of survey and social and political environment affect recruitment to a web panel. Added Value: This analysis focuses on the mode effect on the recruitment of participants for a scientific research panel. Our findings highlight the effect of the social and political environment, which could be used as a source of inspiration for other local studies. Initiating Chain-Referral for Virtual Respondent-Driven Sampling – A Pilot Study with Experiments 1German Institute for Economic Research; 2University of Bremen; 3German Center for Integration and Migration Relevance & Research Question RDS is a network sampling technique for surveying complex populations in the absence of sampling frames. The idea is simple: identify some people (“seeds”) who belong or have access to the target population, encourage them to start a survey invitation chain-referral process in their community, ensure that every respondent can be traced back along the referral chain. But who will recruit? And whom? And which strategies help initiate the referral process? Methods & Data We conducted a pilot study in 2023 where we invited 5,000 panel study members to a multi-topic online survey. During the survey, we asked respondents whether they would be willing to recruit up to three of their network members. If they agreed, we asked them about their relationship with those network members as well as these people’s ages, gender, and education and provided unique survey invitation links to be shared virtually. As part of the study, we experimentally varied the RDS consent wording, information layout, and survey link sharing options. We also applied a dual incentive scheme, rewarding seeds as well as recruits. Results Overall, 624 initial respondents (27%) were willing to invite network members. They recruited 782 people (i.e., on average 1.25 people per seed). Recruits were mostly invited via email (46%) or WhatsApp (43%) and belonged to the seeds’ family (53%) and friends (38%). Only 20% of recruits are in contact with the seed less than once a week, suggesting recruitment mostly among close ties. We find an adequate gender balance (52% female) and representation of people with migration background (22%) in our data, but a high share of people with college or university degrees (52%) and high median age (52 years). The impact of the experimental design on recruitment success is negligible. Added Value While in theory, RDS is a promising procedure, it often fails in practice. Among other challenges, this is commonly due to the fact that seeds will not or only insufficiently start the chain-referral process. Our project shows in which target groups initiating RDS may work and to what extent UX enhancements may increase RDS success. |
11:45am - 12:45pm | A5.2: Detecting Undesirable Response Behavior Location: Seminar 3 (Room 1.03/1.04) Session Chair: Jan-Lucas Schanze, GESIS - Leibniz-Institut für Sozialwissenschaften, Germany |
|
Who is going back and why? Using survey navigation paradata to differentiate between potential satisficers and optimizers in web surveys 1GESIS – Leibniz-Institut für Sozialwissenschaften in Mannheim, Germany; 2Utrecht University, Netherlands Relevance & Research Question: Survey navigation paradata presents a unique opportunity to delve into the web survey completion behavior of respondents, particularly actions like revisiting questions and potentially altering answers. Such behavior could be indicative of motivated misreporting, especially when respondents revisit filter or looping questions to modify answers and circumvent subsequent inquiries — a manifestation of satisficing behavior. Conversely, altering answers upon revisiting may also signify optimizing behavior, where respondents strive for utmost accuracy. This study focuses on the revisiting behavior of web survey respondents, aiming to quantify its frequency, identify associated respondent characteristics, and ascertain who shortens their questionnaire through revisiting. Methods & Data: Using paradata from the probability-based online-administered Generations and Gender Programme (GGP) survey in Estonia (N=8916), we analyze the frequency of revisiting questions, characteristics of these questions, and the ensuing actions. We investigate the connection between revisiting behavior and respondent characteristics using a zero-inflated Poisson regression model and check which respondents’ characteristics were connected with a higher proportion of shortening the questionnaire as a result of revisiting questions. Results: We find a discernible pattern of revisiting questions during the survey, notably prevalent in immediate filter questions, where almost half of respondents go back after a filter question (that can change the routing of the questionnaire). Added Value: This study contributes a nuanced understanding of respondents' behavior during web survey self-completion. Utilizing paradata enhances insights into respondents' survey completion patterns and various behavioral types, providing valuable insights for survey design and data quality management. Socially Desirable Responding in Panel Studies – Does Repeated Interviewing Affect Answers to Sensitive Behavioral Questions? GESIS - Leibniz Institute for the Social Sciences Relevance and Research Question: Social desirability (SD-) bias (i.e., the tendency to report socially desirable opinions and behaviors instead of revealing true ones) is a widely known threat to response quality and the validity of self-reports. Previous studies investigating socially desirable responding in a longitudinal context provide mixed evidence on whether SD-bias increases or decreases with repeated interviewing and how these changes affect response quality in later waves. However, most studies were non-experimental and only suggestive of the underlying mechanisms of observed changes in SD-bias over time. Methods and Data: This study investigates socially desirable responding in panel studies using a longitudinal survey experiment comprising six panel waves. The experiment manipulated the frequency of receiving identical sensitive questions (target questions) and assigned respondents to one of three groups: One group received the target questions in each wave (fully conditioned), the second group received the target questions in the last three waves (medium conditioned), and the control group received the target questions only in the last wave of the study (unconditioned). The experiment was conducted within a German non-probability (n = 1,946) and a probability-based panel study (n = 4,660), resulting in 2x3 experimental groups in total. The analysis focusses on between-group and within-group comparisons of different sensitive behavioral measures. It further includes measures on the questions’ degree of sensitivity as a moderating variable. These measures result from an additional survey (n = 237) in which respondents were asked to rate the sensitivity of multiple attitudinal and behavioral questions. To further examine the underlying mechanisms of change, I use a measure on respondents’ trust towards the survey (sponsor) and the scores of an established SD-scale.
Results: Results will be presented at the conference in February. Added Value: Altogether, this study provides experimental evidence on the impact of repeated interviewing on changes in social desirability bias. It further contributes to the understanding of what causes these changes by examining different levels of exposure to identical sensitive questions and including measures on respondents’ trust towards the survey (sponsor) and their scores on a SD-scale. Distinguishing satisficing and optimising web survey respondents using paradata GESIS – Leibniz-Institut für Sozialwissenschaften in Mannheim, Germany Relevance & Research Question This study seeks to investigate the interplay between different paradata types and data quality indicators derived from survey data, aiming to identify distinct patterns characterizing respondents' satisficing and optimizing behaviors. Methods & Data Employing a laboratory two-wave experiment with a crossover design involving 93 students, participants were randomly assigned to either satisficing or optimizing conditions in the first wave, with groups reversed in the second. Participants were asked to complete a web survey in either satidficing or in optimising manner. Manipulation checks were used to ensure participants' compliance with a condition. The survey encompassed open-ended, factual, and matrix questions, coupled with reliable scales gauging trust, values, and other sociological and psychological measures. Paradata, such as completion time, mouse movements, browser focus, reaction to warnings, scrolling, and resizing, were collected using the One Click Survey (1ka.si) online software. Added Value |
11:45am - 12:45pm | B5: To Trace or to Donate, That’s the Question Location: Seminar 2 (Room 1.02) Session Chair: Alexander Wenz, University of Mannheim, Germany |
|
Exploring the Viability of Data Donations for WhatsApp Chat Logs 1GESIS - Leibniz Institute for the Social Sciences; 2Ulm University Relevance & Research Question Data donations are a new tool for collecting research data. They can ensure informed consent, highly granular, retrospective, and potentially less biased behavioral traces, and are independent from APIs or webscraping pipelines. We thus seek to explore the viability of data donations for a type of highly personal data: WhatsApp chat logs. Specifically, we are exploring a wide range of demographic, psychological, and relational charactersitics and how they relate to peoples donation willingness, censoring, and actual data donation behavior. We used an opt-in survey assessing demographics, personality, relationship characteristics of a self-selected social relationship, and concerns for privacy. Participants were also asked whether they are willing to donate a WhatsApp chat from a 1:1 chat from the respective relationship. If they agreed, participants were forwarded to an online platform where they could securely upload, review, self-censor, and donate the chat log. Donated chats were anonimized automatically by first extracting variables of interest (e.g. number of words per message, emoji, smilies, sent domains, response time) and then deleting the raw message content. In a second step, participants selected which parts of the anonymized data should be included in the donations. The study was reviewed and accepted by the ethics committee of Ulm University. So far, 244 people participated in the survey and 140 chat log files with over 1 million messages in total were donated. Preliminary Results Preliminary results (based on 198 ppts.) show that participants were mostly university students. Self-indicated willingness to donate a chat was surprisingly high (73%), with a sizable gap to actual donations (39.4%). Interestingly participants rarely excluded any data manually after the automatic anonimization step. Furthermore, we did not find any meaningful differences in data donation willingness and behavior with respect to demographics, personality, privacy concerns, or relationship characteristics. Added Value The Mix Makes the Difference: Using Mobile Sensing Data to Foster the Understanding of Non-Compliance in Experience Sampling Studies 1Charlotte Fresenius Hochschule, University of Psychology, Germany; 2LMU Munich, Department of Psychology Relevance & Research Question For decades, social sciences have focused on broad one-time assessments and neglected the role of momentary experiences and behaviors. Now, novel digital tools facilitate the ambulatory collection of data on a moment-to-moment basis via experience sampling methods. But the compliance to answer short questionnaires in daily life varies considerably between and within participants. Compliance and consequently mechanisms leading to missing data in experience sampling studies, however, still remain in the dark today. In our study we therefore explored person-, context- and behavior-related patterns associated with participants’ compliance in experience sampling studies. Methods & Data We used a data set part (N = 592) of the Smartphone Sensing Panel Study recruited according to quotas representing the German population. We extracted over 400 different person-, context-, and behavior-related variables by combining assessments from traditional surveys (e.g., personality traits), experience sampling (e.g., mood), and passively collected mobile sensing data (e.g., smartphone usage, GPS). Based on more than 25,000 observations, we predicted participants' compliance to answer experience sampling questionnaires. For this purpose, we used a machine learning based modeling approach and benchmarked different classification algorithms using 10-fold cross-validation. In addition, we applied methods from interpretable machine learning to better understand the importance of single variables and constellations of variable groups. Results We found that compliance to experience sampling questionnaires could be successfully predicted above chance and that among the compared algorithms the linear elastic net model performed best (MAUC = 0.723). Our follow-up analysis showed that study-related past behaviors such as the average response rate to previous experience sampling questionnaires were the most informative, followed by location information such “at home” or “at work”. Added Value Our study shows that compliance in experience sampling studies is related to participants' behavioral and situational context. Accordingly, we illustrate systematic patterns associated with missing data. Our study is an empirical starting point for discussing the design of experience sampling studies in social sciences and for pointing out future directions in research addressing experience sampling methodology and missing data. |
11:45am - 12:45pm | C5: Politics, Media, Trust Location: Seminar 4 (Room 1.11) Session Chair: Felix Gaisbauer, Weizenbaum-Institut e.V., Germany |
|
What makes media contents credible? A survey experiment on the relative importance of visual layout, objective quality and confirmation bias for public opinion formation Konstanz University, Germany Relevance & Research Question The emergence of social media has transformed the way people consume and share information. As such platforms widely lack mechanisms to ensure content quality, their increasing popularity has raised concerns about the spread of fake news and conspiracy beliefs – with potentially harmful effects on public opinion and social cohesion. Our research aims to understand the underlying mechanisms of media perception and sharing behaviour when people are confronted with factual vs conspiracy-based media contents. Under which circumstances do people believe in a media content? Do traditional indicators of quality matter? Are pre-existing views more important than quality (confirmation bias)? How is perceived credibility linked to sharing behaviour? Methods & Data To empirically assess these questions, we administered a survey experiment to a general population sample in Germany via Bilendi in August 2023. As respondents with a general susceptibility to conspiracy beliefs are of major substantive interest, we made use of responses from a previous survey to oversample “conspiracy thinkers”. Respondents were asked to evaluate the credibility of different media contents related to three vividly debated topics: vaccines against Covid-19, the climate crisis, and the Ukraine war. We analyze these evaluations regarding the objective quality of the content (measured by author identity and data source), its visual layout (newspaper vs tweet), and previous respondent beliefs on the respective topic to measure confirmation bias. Results Our findings suggest that the inclination to confirm pre-existing beliefs is the most important predictor for believing a media content, irrespective of its objective quality. This general tendency applies to both, the mainstream society and “conspiracy thinkers”. However, according to self-reports, the latter group is much more likely to share media contents they believe in. Added Value Methodologically, we use an interesting survey experiment that allows us to vary opinion (in)consistency and objective quality of media contents simultaneously, meaning that we can estimate the relative effect of these features on the credibility of media contents. We provide insights into the underlying mechanisms of the often debated spread of conspiracy beliefs through online platforms, with their practical implications for public opinion formation. Sharing is caring! Youth Political Participation in the Digital Age GESIS, Germany Relevance & Research Question Navigating Political Turbulence: A Study of Trust and online / offline Engagement in Unstable Political Contexts The Max Stern Yezreel Valley College, Israel Relevance & Research Question: Within the backdrop of Israel's turbulent 2022 elections, the fifth round of elections within three years, This study delves into the complex interplay between political trust, efficacy, and engagement. It seeks to unravel how individuals' trust in politicians and the political system, coupled with their sense of political efficacy, influences their online and offline engagement in the political process. The research question focuses on identifying the specific predictors of political engagement in a context characterized by political unpredictability and frequent elections. Methods & Data: The study analyzes a representative survey of 530 Israeli respondents during the 2022 Israeli election period. The research evaluates the influence of various variables. These include trust in politicians, the political system, and political efficacy in online and offline political engagement. The analysis focuses on the differentiation between online engagement, such as social media activity, and offline engagement, like attending rallies or voting. Results: Statistical analysis reveals a robust correlation between political efficacy and both forms of political engagement (r = .62 for online, r = .57 for offline, p < .01). Trust in the political system emerges as a significant predictor of offline engagement (β = .36, p < .01), while trust in politicians is more strongly associated with online engagement (β = .41, p < .01). Notably, a mediation analysis indicates that political efficacy serves as a mediator in the relationship between trust in politicians and online engagement (indirect effect = 0.15, 95% CI [0.07, 0.24], p < .01). In contrast, such mediating effects between system trust and offline engagement are not observed. Added Value: By examining the nuanced factors influencing political engagement during political uncertainty, this study offers new insights into the differentiated impact of trust in politicians and the political system. It underscores the distinct psychological pathways that drive online and offline political engagement, enhancing our understanding of citizen behavior in democracies facing political instability. These findings have critical implications for political strategists, policymakers, and scholars seeking to foster civic engagement in similar contexts. |
11:45am - 12:45pm | D5: KI Forum: Impuls-Session - Chancen und Regulierungen Location: Auditorium (Room 0.09/0.10/0.11) Session Moderators: Oliver Tabino, Q Agentur für Forschung Yannick Rieder, Janssen-Cilag GmbH Georg Wittenburg, Inspirient This session is in German. |
|
EU AI Act: Innovationsmotor oder Innovationsbremse? KI Bundesverband, Germany Der Artificial Intelligence Act (AI Act) der EU ist das erste Regelwerk, das sich mit der Regulierung von Künstlicher Intelligenz (KI) befasst. Mit dem AI Act will die EU einen weltweiten Goldstandard und eine Blaupause für die Regulierung von KI schaffen. Doch kann der AI Act tatsächlich zum Innovationsmotor für vertrauenswürdige KI werden oder wird er zum wirtschaftlichen Hemmschuh? Das Potential von Foundation Models und Generativer KI – Ein Blick in die Zukunft IAIS, Germany Foundation Models stehen im Zentrum des gegenwärtigen Hypes um (Generative) Künstliche Intelligenz. Sie besitzen das Potential, die Art und Weise, wie wir arbeiten, branchen- und aufgabenübergreifend zu revolutionieren.. Wir präsentieren ein aktuelles Projekt, in dem LLMs für personalisiertes Marketing genutzt werden und wagen einen Blick in die Zukunft von KI. Ein besonderer Fokus liegt auf der Rolle von Open Source in der Demokratisierung der KI-Technologie, dem Potenzial autonomer Agenten, die menschliche Arbeit unterstützen und ergänzen, sowie den Möglichkeiten, die Small Language Models für spezialisierte Anwendungen bieten. |
12:45pm - 2:00pm | Lunch Break Location: Cafeteria (Room 0.15) |
2:00pm - 3:00pm | A6.1: Questionnaire Design Choices Location: Seminar 1 (Room 1.01) Session Chair: Julian B. Axenfeld, German Institute for Economic Research (DIW Berlin), Germany |
|
Grid design in mixed device surveys: an experiment comparing four grid designs in a general Dutch population survey. Statistics Netherlands, Netherlands, The Relevance & Research Question Within the current stylesheet, half of the sample units were randomly assigned to the standard grid design as currently used (a table format for large screens and a stem-fixed vertical scrollable format for small screens) and the other half to a general stem-fixed grid design (stem-fixed design for both the large and the small screen). Within the experimental stylesheet, one third of the sample was randomly assigned to either the general stem-fix grid design, a carrousel grid design (in which only one item is displayed at the time and after answering one item, the next item automatically ‘flies in‘) or an accordion grid design (all items are presented vertically on one page, and answer options are automatically closed and unfolded after an item is answered). Various indicators are used to assess response quality, e.g. break-off, item non response, straightlining, mid-point reporting. Respondent satisfaction is assessed with a set of evaluation questions at the end of the questionnaire. Results Data are currently being analyzed.
Towards a mobile web questionnaire for the Vacation Survey: UX design challenges Statistics Netherlands, Netherlands, The Towards a mobile web questionnaire for the Vacation Survey: UX design challenges Vivian Meertens & Maaike Kompier Key words: Mobile Web Questionnaire Design, Smartphone First Design, Vacation Survey, Statistics Netherlands, UX testing, Qualitative Approach, Mixed Device Surveys Relevance & Research Question: —your text here— Despite the fact that online surveys are not always fit for small screens and mobile device navigation, the number of respondents that start online surveys on mobile devices instead of PC or laptop device, is still growing. Statistics Netherlands (CBS) has responded to this trend by developing and designing mixed device surveys. This study focuses on the redesign of the Vacation Survey, applying a smartphone first approach. The Vacation Survey is a web only panel survey, that could only be completed on a PC or laptop. The layered design with a master detail approach was formatted in such a way that a large screen was needed to be able to complete the questionnaire. Despite a warning in the invitation letter that a PC or laptop should be used to complete the questionnaire, 14.5% of first-time logins in 2023 were via smartphones, resulting in a redesign with a smartphone first approach. The study examines the applicability and understandability of the Vacation Survey’s layered design, specifically its master-detail approach, from a user experience (UX) design perspective. Results: —your text here— Added Value: —your text here Optimising recall-based travel diaries: Lessons from the design of the Wales National Travel Survey National Centre for Social Research, United Kingdom Relevance & Research Question: Recall-based travel diaries require respondents to report their travel behaviour over a period ranging from one to seven days. During this period, they are asked to indicate the start and end times and locations, modes of transport, distances, and the number of people on each trip. Depending on the mode, additional questions are asked to gather information on ticket types and costs or fuel types. Due to the specificity of the requested information and its non-centrality for most respondents, travel diaries pose a substantial burden, increasing the risk of satisficing behaviours and trip underreporting. Methods & Data: In this presentation, we describe key decisions made during the design of the Wales National Travel Survey. This push-to-web project includes a questionnaire and a 2-day travel diary programmed into the survey. Results: Critical aspects of these decisions include the focus of the recall (trip, activity, or location based) and the sequence of follow-up questions (interleaved vs. roster approach). Recent literature suggests that location-based diaries align better with respondents’ cognitive processes than trip-based diaries and help reduce underreporting. Therefore, a location-based travel diary was proposed with an auto-complete field to match inputs with known addresses or postcodes. Interactive maps were also proposed for user testing. While they can be particularly useful when respondents have difficulty describing locations or when places lack formal addresses, previous research warns that advanced diary features can increase drop-off rates. Regarding the follow-up sequence, due to mixed findings in the literature and limited information on the performance of these approaches in web-based travel diaries, experimentation is planned to understand how each approach performs in terms of the accuracy of the filter questions and the follow-up questions. Additionally, this presentation discusses the challenges and options for gathering distance data in recall-based travel diaries, along with learnings from the early phases of diary testing based on the application of a Questionnaire Appraisal System and cognitive/usability interviews. Added Value: These findings offer valuable insights into the design of complex web-based surveys with multiple loops and non-standard features, extending beyond travel diaries. |
2:00pm - 3:00pm | A6.2: Data Quality Assessments 2 Location: Seminar 3 (Room 1.03/1.04) Session Chair: Fabienne Kraemer, GESIS Leibniz-Institut für Sozialwissenschaften, Germany |
|
Can we identify and prevent cheating in online surveys? Evidence from a web tracking experiment. 1University of Oxford, United Kingdom; 2The London School of Economics, United Kingdom; 3Universitat Pompeu Fabra, Spain; 4Institut Barcelona Estudis Internacionals (IBEI), Spain Relevance & Research Question: Survey measures of political knowledge, widely used in political science research, face challenges in online administration due to potential cheating. Previous research reveals a significant proportion of participants resort to online searches when answering political knowledge questions, casting doubt on measurement quality. Existing studies testing potential interventions to curb cheating have relied on indirect measures of cheating, such as catch questions. This study introduces a novel approach, employing direct observations of participants' Internet browsing via web trackers, combined with an experimental design testing two strategies to prevent cheating (instructions and time limit). The paper explores three research questions: what proportion of participants looks up information when posed political knowledge questions (RQ.1)? What is the impact of the interventions on the likelihood of individuals looking up information (RQ.2)? How do estimates from direct observations differ from indirect proxies (e.g., self-reports, paradata) (RQ.3)? A web survey experiment (N = 1,200) in Spain was deployed within an opt-in access online panel. Cross quotas for age and gender, and quotas for educational level, and region were used to ensure a sample matching on these variables to the Internet adult population. Participants answered six knowledge questions on political facts and current events. Cheating was identified by analysing URLs from web tracking data, and alternative indirect measures were applied, including catch questions, self-reports, and paradata. Two noteworthy patterns emerge. Firstly, cheating prevalence from web tracking data is below 5%, markedly smaller than levels estimated by indirect measures (2 to 7 times larger). Secondly, based on web tracking data the anti-cheating interventions have no effect. Nonetheless, using indirect measures of cheating we find that both interventions significantly reduce the likelihood of cheating. This study pioneers the integration of web tracking data and experimental design to examine cheating in online political knowledge assessments. Despite requiring further validation, the substantial differences between web tracking data and indirect approaches suggest two competing conclusion: either cheating in online surveys is substantially lower than first thought, or web tracking data may not be suitable for identifying cheating in online surveys. The Quality of Survey Items and the Integration of the Survey Quality Predictor 3.0 into the Questionnaire Development Process GESIS - Leibniz Institute for the Social Sciences, Germany Relevance & Research Question Probability-based online and mixed-method panels from a data quality perspective 1HUN-REN Centre for Social Sciences, Hungary; 2Panelstory Opinion Polls, Hungary Relevance & Research Question: Probability-based online and mixed-method panels are widely used in scientific research, but not as much for market research or political opinion polling. This presentation will explore the case of "Panelstory", the first Hungarian probability-based mixed-method panel, which was established in 2022 with the purpose of utilizing scientific methods to address market research and political opinion polling issues. |
2:00pm - 3:00pm | B6.1: Automatic analysis of answers to open-ended questions in surveys Location: Seminar 2 (Room 1.02) Session Chair: Barbara Felderer, GESIS, Germany |
|
Using the Large Language Model BERT to categorize open-ended responses to the "most important political problem" in the German Longitudinal Election Study (GLES) GESIS, Germany Relevance & Research Question Open-ended survey questions are crucial e.g., for capturing unpredictable trends, but the resulting unstructured text data poses challenges. Quantitative usability requires categorization, a labor-intensive process in terms of costs and time, especially with large datasets. In the case of the German Longitudinal Election Study (GLES) spanning from 2018 to 2022, with nearly 400,000 uncoded mentions, it prompted us to explore new ways of coding. Our objective was to test various machine learning approaches to determine the most efficient and cost-effective method for creating a long-term solution for coding responses, ensuring high quality simultaneously. Which approach is best suited for the long-term coding of open-ended mentions regarding the "most important political problem" in the GLES? Methods & Data Pre-2018, GLES data was manually coded. Shifting to a (partially) automated process involved revising the codebook. Subsequently, the extensive dataset comprising nearly 400,000 open responses to the question regarding the "most important political problem" in the GLES surveys conducted between 2018 and 2022 was employed. The coding process was facilitated using the Large Language Model BERT (Bidirectional Encoder Representations from Transformers). During the entire process, we tested a whole host of important aspects (hyperparameter finetuning, downsizing of the “other” category, simulations of different amounts of training data, quality control of different survey modes, using training data from 2017) before arriving at the final implementation. The "new" codebook already demonstrates high quality and consistency, evident from its Fleiss Kappa value of 0.90 for the matching of individual codes. Utilizing this refined codebook as a foundation, 43,000 mentions were manually coded, serving as the training dataset for BERT. The final implementation of coding for the extensive dataset of almost 400,000 mentions using BERT yields excellent results, with a 0/1 loss of 0.069, a Micro F1 score of 0.946 and a Macro F1 score of 0.878. The outcomes highlight the efficacy of the (partially) automated coding approach, emphasizing accuracy with the refined codebook and BERT's robust performance. This strategic shift towards advanced language models signifies an innovative departure from traditional manual methods, emphasizing efficiency in the coding process. The Genesis of Systematic Analysis Methods Using AI: An Explorative Case Study TU Dresden, Germany Relevance & Research Question The analysis of open-ended questions in large-scale surveys can provide detailed insights into respondents' views that often can't be assessed with closed-ended questions. However, due to the large number of respondents, it takes a lot of resources to review the answers within open-ended questions and thus provide them as research results. This contribution aims to show the potential benefits and limitations of using AI-based tools (e.g. ChatGPT), for analyzing open-ended questions in large-scaled surveys. It therefore also aims to highlight the challenge of conducting systematic analysis methods with AI. Methods & Data Results Added Value Insights from the Hypersphere - Embedding Analytics in Market Research SPLENDID Research, Germany Relevance & Research Question: In the intersection of qualitative and quantitative research, analyzing open-ended questions remains a significant challenge for data analysts. The incorporation of AI language models introduces the complex embedding space: a realm where semantics intertwine with mathematical principles. This paper explores how Embedding Analytics, a subset of explainable AI, can be utilized to decode and analyze open-ended questions effectively. Methods & Data: Our approach utilized the ada_V2 encoder to transform market research responses into spatial representations on the surface of a 1,536-dimensional hypersphere. This process enabled us to analyze semantic similarities using traditional statistics as well as advanced machine learning techniques. We employed K-Means Clustering for text grouping and respondent segmentation, and Gaussian Mixture Models for overarching topic analysis across numerous responses. Dimensional reduction through t-SNE facilitated the transformation of these complex data sets into more comprehensible 2D or 3D visual representations. Results: Utilizing OpenAI’s ada_V2 encoder, we successfully generated text embeddings that can be plausibly clustered based on semantic content, transcending barriers of language and text length. These clusters, formed via K-Means and Gaussian Mixture Models, effectively yield insightful and automated analyses from qualitative data. The two-dimensional “cognitive constellations” created through t-SNE offer clear and accessible visualizations of intricate knowledge domains, such as brand perception or public opinion. Added Value: This methodology allows for a precise numerical analysis of verbatim responses without the need for labor-intensive manual coding. It facilitates automated segmentation, simplification of complex data, and even enables qualitative data to drive prediction tasks. The rich, nuanced datasets derived from semantic complexity are suitable for robust analysis using a wide range of statistical methods, thereby enhancing the efficacy and depth of market research analysis. |
2:00pm - 3:00pm | B6.2: AI Tools for Survey Research 2 Location: Seminar 4 (Room 1.11) Session Chair: Florian Keusch, University of Mannheim, Germany |
|
Vox Populi, Vox AI? Estimating German Public Opinion Through Language Models 1LMU Munich, Germany; 2University of Mannheim, Germany Relevance & Research Question: Integrating LLMs into cognitive pretesting procedures: A case study using ChatGPT GESIS - Leibniz Institute for the Social Sciences, Germany Relevance & Research Question Using Large Language Models for Evaluating and Improving Survey Questions 1University of Mannheim, Germany; 2LMU Munich, Germany Relevance & Research Question: The recent advances and availability of large language models (LLMs), such as OpenAI’s GPT, have created new opportunities for research in the social and behavioral sciences. Questionnaire development and evaluation is a potential area where researchers can benefit from LLMs: Trained on large amounts of text data, LLMs might serve as an easy-to-implement and inexpensive method for both assessing and improving the design of survey questions, by detecting problems in question wordings and suggesting alternative versions. In this paper, we examine to what extent GPT-4 can be leveraged for questionnaire design and evaluation by addressing the following research questions: (1) How accurately can GPT-4 detect problematic linguistic features in survey questions compared to existing computer-based evaluation methods? (2) To what extent can GPT-4 improve the design of survey questions? Methods & Data: We prompt GPT-4 with a set of survey questions and ask to identify features in the question stem or the response options that can potentially cause comprehension problems, such as vague terms or a complex syntax. For each survey question, we also ask the LLM to suggest an improved version. To compare the LLM-based results with an existing computer-based survey evaluation method, we use the Question Understanding Aid (QUAID; Graesser et al. 2006) that rates survey questions on different categories of comprehension problems. Based on an expert review among researchers with a PhD in survey methodology, we assess the accuracy of the GPT-4- and QUAID-based evaluation methods in identifying problematic features in the survey questions. We also ask the expert reviewers to evaluate the quality of the new question versions developed by GPT-4 compared to their original versions. Results: We compare both evaluation methods with regard to the number of problematic question features identified, building upon the five categories used in QUAID: (1) unfamiliar technical terms, (2) vague or imprecise relative terms, (3) vague or ambiguous noun phrases, (4) complex syntax, and (5) working memory overload. Added Value: The results from this paper provide novel evidence on the usefulness of LLMs for facilitating survey data collection. |
2:00pm - 3:00pm | D6: KI Forum: KI Café Location: Auditorium (Room 0.09/0.10/0.11) Session Moderators: Oliver Tabino, Q Agentur für Forschung Yannick Rieder, Janssen-Cilag GmbH Georg Wittenburg, Inspirient This session is in German. Moderierter Austausch zu folgenden Themen: • Messbare Qualität von KI-Tools ist Grundlage für Vertrauen und Voraussetzung für den betrieblichen Einsatz, aber welche Qualitätskriterien haben sich bewährt? Wie können sie erfasst und verglichen werden? • Wie implementiert man KI-Anwendungen in Prozesse? Wobei ist die Nutzung bereits etabliert? Was gibt es dabei zu beachten? • KI und Ethik: Was geht und was nicht? |
3:00pm - 3:15pm | Break |
3:15pm - 4:15pm | A7.1: Survey Methods Interventions 2 Location: Seminar 1 (Room 1.01) Session Chair: Joss Roßmann, GESIS - Leibniz Institute for the Social Sciences, Germany |
|
Pushing older target persons to the web: Do we still need a paper questionnaire? GESIS - Leibniz-Institut für Sozialwissenschaften, Germany Relevance & Research Question Methods & Data Results Added Value Clarification features in web surveys: Usage and impact of “on-demand” instructions GESIS - Leibniz Institute for the Social Sciences, Germany Relevance & Research Question |
3:15pm - 4:15pm | A7.2: Social Media Recruited Surveys Location: Seminar 3 (Room 1.03/1.04) Session Chair: Tobias Rettig, University of Mannheim, Germany |
|
Assessing the impact of advertisement design on response quality in surveys using social media recruitment 1Max Planck Institut for Demographic Research, Germany; 2Bielefeld University, Germany Relevance & research question: Researchers are increasingly using social media platforms for survey recruitment. Typically, advertisements are distributed through these platforms to motivate users to participate in an online survey. To date, there is little empirical evidence on how the content and design characteristics of advertisements can affect response quality in surveys based on social media recruitment. This project is the first comprehensive study of the effects of ad design on response quality in surveys recruited via social media. Methods and data: We use data from the SoMeRec survey, which was conducted via Facebook ads in Germany and the United States in June 2023 and focused primarily on climate change and migration. The survey ad campaign featured 15 images with different thematic associations to climate change and migration, including strong and loose associations and neutral images. A commercial access panel company was contracted to include identical survey questions serving as benchmark comparison. The Facebook sample consisted of 7,139 respondents in Germany and 13,022 in the US, while the access panel consisted of 1,555 surveys in Germany and 1,576 surveys in the US. In our analyses, we compare common data quality indicators, including completion time, straightlining, item non-response, and follow-up availability, across different ad features. Results: First analysis show that survey completion time is higher for thematic ad designs compared to neutral ads and the reference sample. There are differences in the overall item non-response rate, with higher item non-response for the immigration-themed ad designs. There are no significant differences in straightlining between samples and ad designs. Finally, respondents recruited through neutral ads were more likely to be available for follow up surveys than those recruited through themed ads. Added value: Our study advances the literature by studying the general population in Germany and the US, by testing various indicators of survey data quality, and by including a benchmark survey of respondents not recruited through social media. The results clearly indicate an effect of ad design on survey data quality and highlight the importance of sample and recruitment design for estimates based on social media recruitment and online surveys. Do expensive social media ad groups pay off in the recruitment of a non-probabilistic panel? An inspection on coverage and cost structure GESIS Leibniz Institute for the Social Sciences, Germany Relevance & Research Question: Social media advertisement is becoming an increasingly popular method of recruiting participants for studies in the social sciences. Recently, more and more participants of surveys are recruited via social media. This method of recruitment has been particularly prominent for recruiting special populations for surveys, such as migrants or LGBT persons, but recently meta has significantly reduced these selection criteria. However, meta still allows the selection of common socio-demographic characteristics, such as age and gender, when placing an ad. Meta estimates these socio-demographic characteristics based on the user's data. With this information, we took an non-probabilistic quota-sampling-like approach by specifying to meta the desired peoples' proportions for socio-demographic characteristics which should click on the ad and be directed to the recruitment survey of our nonprobabilistic panel. However, the volatile and hard to control nature of social media recruitment opens it up to scrutiny and demands evaluation. In this study we assess coverage issues and cost effectiveness of utilizing Meta advertisement in recruiting respondents for a non-probabilistic online panel, we consider three aspects in detail. First, we evaluate the extent to which the targeting criteria, namely age and gender achieve a balanced sample at different stages of the registration process into the panel and give recommendations for adjustments. Furthermore, we validate whether these social media targeting criteria are reliable and agree with the survey answers. Third, we assess the cost structure in the light of the response propensities at the different stages of the recruitment process and investigate whether expensive social media ad groups pay off in the long-term. Methods & Data: We are using data from the recruiment of the new GESIS Panel Plus. The recuitmenr process includes several steps and we sill consider each step individually using multivariate analysis methods. Results: First results suggest that expensive recruitment groups do not pay off in the long term. Added Value: These research will open up the black box of cost structure in relation to socio - demographic attributes when using Meta as recruitment frame for cross-sectional and longitudinal surveys. |
3:15pm - 4:15pm | B7: Mobile Apps and Sensors Location: Seminar 2 (Room 1.02) Session Chair: Ramona Schoedel, Charlotte Fresenius Hochschule, University of Psychology, Germany |
|
Mechanisms of Participation in Smartphone App Data Collection: A Research Synthesis University of Mannheim Relevance & Research Question: Smartphone app data collection has recently gained increasing attention in the social and behavioral sciences, allowing researchers to integrate surveys with sensor data, such as GPS to measure location and movement. Similar to other forms of surveys, participation rates of such studies in general population samples are generally low. Previous research has identified several study- and participant-level determinants of willingness to participate in smartphone app data collection. However, a comprehensive overview of which factors are predictors of willingness and a theoretical framework are currently lacking and some of the effects are inconsistent. To guide future app-based studies, we address the following research questions: (1) Which study- and participant-level characteristics affect the willingness to participate in smartphone app data collection? (2) Which theoretical frameworks can be used to understand participation decisions in smartphone app data collection? Methods & Data: We conduct a systemic review and a meta-analysis on existing studies with app-based data collection guided by the Preferred Reporting Items for Systematic reviews and Meta-analysis (PRISMA) framework (Moher et al. 2009). We compile a list of keywords to search for relevant literature in bibliographic databases. We focus on peer-reviewed articles published in English. We also perform double coding to ensure a reliable selection of literature for the analysis. Finally, we map the identified determinants of willingness to potential theoretical frameworks that can explain participation behavior. Results: In the systematic review, we summarize findings about study-level characteristics that are under the researchers' control, such as monetary incentives or invitation mode, and participant-level characteristics, such as privacy concerns and socio-demographics. Meanwhile, the meta-analysis focuses on selected characteristics, which have been most often covered in previous research. Added Value: This study will provide a holistic understanding of the current state of research on participation decisions in app-based studies. The findings will also help researchers to design effective invitation strategies for future studies. “The value of privacy is not as high as finding my person”: Self-disclosure practices on dating apps illustrate an existential dilemma for data protection University of Oxford, United Kingdom Relevance & Research Question: Dating apps create a unique digital sphere where people must disclose sensitive personal information about their demographics, location, values and lifestyle. Because of these intimate disclosures, dating apps constitute a strategic research site to explore how privacy concerns influence personal information disclosure. We use construal-level theory to understand how context influences a decision to disclose. Construal-level theory refers to the influence of psychological distance: the more psychologically distant an event the more mental effort required to understand it. When people have no direct experience in a context they rely on conventional stereotypes and quick generalizations. Using this theory we ask the research question: Why do people choose to disclose or not disclose personal Information on their dating app profile? Money or Motivation? Decision Criteria to participate in Smart Surveys Destatis - Federal Statistical Office Germany, Germany Relevance & Research Question The German Federal Statistical Office (Destatis) is continuing to develop its data collection instruments and is working on smart surveys in this context. By smart surveys we mean the combination of traditional question-based survey data collection and digital trace data collection by accessing device sensor data via an application (GPS, camera, microphone, accelerometer, ...). Unlike traditional surveys, smart surveys not only ask respondents for information but also require them to download an app and allow access to sensor data. Destatis conducted focus groups to learn more about the attitudes, motives and obstacles regarding the willingness to participate in smart surveys. This was done as part of the European Union's Smart Survey Implementation (SSI) project, in which Destatis is participating alongside other project partners. Methods & Data Three focus groups with a total of 16 participants were conducted at the end of October 2023. The group discussions were led by a moderator using a guideline. The discussions lasted around two hours each and were video-recorded. Results Overall, it became clear that participants are more willing to take part in a survey, to download an app and to grant access to sensor data if they see a purpose in doing so on the one hand and have trust on the other. In order to motivate people to participate, it seems particularly important against this background to provide transparent information explaining why to conduct the survey, why they should participate, why access to the sensor data is desired as well as what is being done to ensure a high level of data protection and data security. Added Value In official statistics, the development of new survey methods is seen as an important step towards modern data collection. However, modern survey methods can only make a positive contribution if they are used by respondents. The results are intended to provide information on how potential respondents can best be addressed to participate. In the further course of the SSI project, a quantitative field test for recruitment is planned. The results of the focus groups will also be used to prepare this test. |
Contact and Legal Notice · Contact Address: Privacy Statement · Conference: GOR 24 |
Conference Software: ConfTool Pro 2.8.101 © 2001–2024 by Dr. H. Weinreich, Hamburg, Germany |