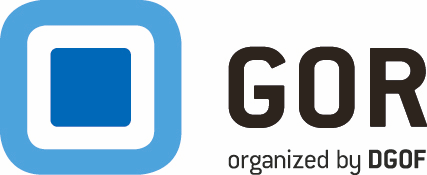
General Online Research Conference 2024 (GOR 24)
Rheinische Fachhochschule Cologne - Campus Vogelsanger Straße
21 - 23 February 2024
Conference Agenda
Overview and details of the sessions of this conference. Please select a date or location to show only sessions at that day or location. Please select a single session for detailed view (with abstracts and downloads if available).
|
Session Overview | |
Location: Auditorium (Room 0.09/0.10/0.11) Rheinische Fachhochschule Köln Campus Vogelsanger Straße Vogelsanger Str. 295 50825 Cologne Germany |
Date: Wednesday, 21/Feb/2024 | |
5:00pm - 6:30pm | DGOF Members General Meeting Location: Auditorium (Room 0.09/0.10/0.11) |
Date: Thursday, 22/Feb/2024 | |
9:00am - 10:15am | GOR 24 Opening & Keynote I Location: Auditorium (Room 0.09/0.10/0.11) |
|
Digital monopolies: How Big Tech stole the internet - and how we can reclaim it AMP Digital Ventures, Germany Data measurements prove crystal clear: The huge amount of domains on the internet is meaningless, it is only a handful of tech companies which are attracting the majority of digital traffic. The rest of the internet resembles a huge graveyard. Digital monopolies are currently bringing ever larger parts of our lives under their control. The platforms are increasingly dominating the formation of political opinion and at the same time are deleting our free market economy. We should ask ourselves: is this still legal? Why should we put up with it any longer? Media scientist Martin Andree shows how far the hostile takeover of our society by the tech giants has already progressed - and how we can reclaim the internet. Moreover, the keynote will address the specific role of science and market research, which should take a clear position towards these problems. The current destabilization of Western democracies shows how much people depend on competent scientific media research to provide guidelines and orientation for society in order to save our democracy, especially in times of disinformation and fake news. It’s time to take over responsibility and make a change for the better – as long as it is still possible.
|
10:45am - 11:45am | D1: Best Practice Cases Location: Auditorium (Room 0.09/0.10/0.11) Session Chair: Yannick Rieder, Janssen EMEA, Germany |
|
Brave new world! How artificial intelligence is changing employee research at DHL. 1r)evolution GmbH, Germany; 2DHL Group Relevance & Research Question We try to estimate the possible financial, personnel and time savings by using AI and we want to show how AI can improve the outcome of a research system. Abstract In an annual online survey, DHL Group collects structured and open feedback from around 550,000 employees. This Employee Opinion Survey (EOS) is conducted worldwide in 55 languages and for 60,000 organizational units. Due to its size, the operational implementation of the survey requires the use of large financial and human resources. It is not surprising that various stakeholders expressed a desire to optimize the survey: HR departments wanted more automation and a more effective follow-up process. Team heads wished more specific recommendations for action and top management wanted an optimized use of resources. EOS project team then asked itself how AI could help to make the project simpler, more insightful, and more effective. All process steps were evaluated, and five AI pilots/projects were rolled out. 1. AI to automate survey setup. The challenge is that the reporting structure cannot be derived directly from the formal line organization. And therefore, it’s a major manual effort for the HR departments to assign 550,000 employees to their teams. To optimize the process, we used machine learning models to automatically assign employees to the reporting structure. 2. AI to improve online questionnaire. In online surveys, the answers to open questions often remain short, as there is no interviewer to explore. To fill this gap, we used a GPT model that reacts individually to the respondent's open answer and asks additional in-depth questions. 3. AI to speed up the open comment processing. We used AI to translate, anonymize and categorize the 142,000 open comments in a fully automated process. 4. AI to make results dashboard more user-friendly and effective. We implemented a chatbot in our reporting tool that uses the current OpenAI GPT model. The chatbot starts with an individual management summary and answers specific questions of the users. 5. AI to predict which follow-up measures are particularly effective. We evaluated the initiatives documented in the action planning tool from previous years with next year’s EOS results using an AI-supported decision tree analysis. Using these five AI projects as examples, we show the opportunities, limits and risks of using AI, especially for large research systems. |
12:00pm - 1:15pm | D2: Innovation in Practice: LLMs and more ... Location: Auditorium (Room 0.09/0.10/0.11) Session Chair: Stefan Oglesby, data IQ AG, Switzerland |
|
Beyond Reports: Maximizing Customer Segmentation Impact with AI-Driven Persona Conversations Factworks, Germany Relevance & Research Question Segmentation is a challenging, core strategic research task, enabling businesses to understand the many needs of diverse customer groups. Yet, its effectiveness lies in its adoption within an organization. Despite AI's pervasive influence, its potential benefit in segmentation studies remains underutilized. This prompts us to explore: To what extent can AI help us socialize segmentation research, enabling stakeholder interaction with data and driving organizational adoption to influence business outcomes? Our research introduces an innovative approach leveraging AI-driven persona chatbots, tapping into Language Model-based systems like ChatGPT. Our aim is to create an interactive chatbot that can be shared across organizational departments, facilitating in-depth familiarization with customer segments. To do that, we train a GPT model on a comprehensive dataset combining quantitative and qualitative research findings from a study on segmenting online travel booking site users. This will enable us to evaluate its potential as a tool to humanize research findings and share accurate information about identified segments. Our research plans to assess the chatbot's capacity to uphold factual accuracy based on its training data while also exploring its ability to generate creative yet aligned responses consistent with the characteristics of the segmented customer groups it represents. Initial assessments showcase promising signs of the chatbot's capacity to navigate between factual accuracy and creative engagement, aligning well with the segmented customer profiles it represents. However, its effectiveness heavily depends on well-engineered prompt design. Added Value In embracing this innovative approach, our goal is to create a tool that aids organizations in unlocking the full potential of segmentation. By encouraging greater immersion and fostering deeper empathy with consumer segments, our persona chatbot aims to make research findings more accessible to wider, less research-savvy audiences and enables them to get to know segments in a more playful and engaging way. How good are conversational agents for online qualitative research? 1Université Paris Nanterre, France; 2Bilendi Relevance & Research Question Conversational agents such as chatGPT open up new ways for online qualitative research. On the analysis side, they may be used to extract key ideas and to provide participants’ quotes illustrating those. On the field management side, they may be used for moderation, to help dig deeper into what participants think. However, beyond the obvious advantages in terms of feasibility, numbers, speed and costs, the question how AI supplemented research design fares compared to purely human driven research appears as a pressing and hard to address question. Our goal in this research is more precisely to assess the quality of AI supplemented qualitative research for analysis and moderation, by comparison with human standards. Methods & Data We shall compare results obtained with and without a chatGPT powered AI assistant on a recently launched qualitative research platform www.bilendi.de/static/bilendi-discuss enabling the use of such an assistant. Regarding analysis, we qualitatively compare the results of a pure human analysis with those of the ChatGPT powered analysis. Regarding moderation, we quantitatively compare the response rate of participants to human moderators vs the chatGPT powered moderator. The data will consist in two data sets, a first study run in Finland in September 2023 gathering 22 participants, and a second study run in France and Germany and the UK over November-December 2023 gathering 30 participants per country. A pilot was run for this second demo in France in November 2023 with 225 participants. In the Finnish study (analysis only), ideas provided by the ChatGPT powered assistant were found to be 70% consistent with those of the human analysis, 20% consistent but ‘not usable as such’ and 10% inconsistent. In the pilot study for the second demo (moderation only), response rate to human moderators was 85% and response rate to the ChatGPT powered assistant was 74.63%. Added Value Recent research by Chopra and Haaland (‘Conducting Qualitative Interviews with AI’, Cesifo Working Paper, 2023) provides encouraging evidence in terms of participants engagement and generated insights. The present research develops on those results by coming with systematic comparisons between human and machine performance. Smartphone app-based mobility research intervista AG, Switzerland Thanks to GPS tracking with a smartphone app, a person's mobility behavior can be tracked in great detail. The information obtained on stages, routes, transport use and mobility purposes offers real added value in many areas of research. In this presentation, Beat Fischer explains the methodology, provides insights into the data science behind it and shows case studies with data from the Swiss Footprints Panel. |
2:30pm - 3:30pm | P 1.1: Postersession Location: Auditorium (Room 0.09/0.10/0.11) |
|
Fear in the Digital Age – How Nomophobia together with FoMO and extensive smartphone use lowers social and psychological wellbeing Rheinische Fachhochschule gGmbH (RFH), Germany Relevance & Research Question While FoMO (Fear of Missing Out) is already well known as an important factor that leads to extensive smartphone use (ESU) and lowers wellbeing (WB), research starts to look at the new phenomenon Nomophobia (the fear of being separated from one’s smartphone and not being connected and reachable, e.g. Yildirim & Correia, 2015). However, it still remains unclear, how Nomophobia lowers wellbeing – social as well as psychological wellbeing – over and above the already known factors FoMO and ESU. Methods & Data This study (ad-hoc-sample: N=132) combines all factors in one design and investigates to what extend Nomophobia (measured by: NMP-Q-D Coenen & Görlich, 2022) is an additional factor that causes negative effects on wellbeing (measured by: FAHW, Wydra, 2020) over and above FoMO (measured by FoMO, Spitzer, 2015) as well as ESU (measured by: SAS-SV, Randler et al., 2016). Several regression analyses calculated the effect sizes for the main effects as well as the interaction effects for the different factors – controlled for age and gender. Results Interestingly, different effects can be found regarding psychological wellbeing compared to social wellbeing. Whereas ESU (beta=-.31, p<.01) but not nomophobia lowered the psychological wellbeing quite a lot, instead Nomophobia (beta=-.18, p<.10) but not ESU lowered the social wellbeing significantly. FoMO was similarly a negative factor for psychological (beta=-.22, p<.05) as well as social wellbeing (beta=-.21, p<.05). Interaction effects between all of these factors were tested but could not be found. All in all, quite a part of the variance can be explained only by these three factors: 16% of the variance of psychological wellbeing and 12% of the variance of social wellbeing. Added Value This study extends the knowledge about the factors that causes negative effects on the wellbeing of people in the digital age. Smartphones are so prominent and important nowadays that the fear of losing them can cause additional harm. The results show, that they serve as an important connection tool for social relationships, losing them creates stress and their exorbitant use lowers the wellbeing of people. Is less really more? The Impact of Survey Frequency on Participation and Response Behaviour in an Online Panel Survey German Centre for Higher Education Research and Science Studies (DZHW), Germany Relevance & Research Question Online surveys offer the possibility of interviewing participants of a panel more frequently at reasonable costs. A higher contact frequency might thereby lead to a lower rate of unsuccessful contact attempts through increased bonding with the respondents and address maintenance. If life history data is collected, a higher survey frequency also offers the advantage of shorter reporting periods and decreased time lag for the retrospective collection of these data (Haunberger 2010). This should reduce recall errors and the cognitive burden for respondents. Nevertheless, more frequent interviews also increase the response burden or survey fatigue and could thus lead to a reduced willingness to participate (Haunberger 2010; Schnauber and Daschmann 2016; Stocké and Langfeldt 2003; Nederhof 1986). Until now there is insufficient empirical evidence for survey makers to decide on an optimal design when implementing online panel surveys (see most recently Zabel 1998 for very short wave intervals). Furthermore, existing evidence on survey frequency is limited to CATI and face to face interviews, constraining the validity of possible conclusions about online surveys. We are therefore analysing how the response rate changes when the survey frequency in an online survey is increased. Methods & Data To examine the effect of the survey frequency we implemented an experiment in a panel of secondary school graduates that surveys respondents every two years. To vary the survey frequency, an additional wave was conducted one year after the second wave for a random sample of participants. Both, control and treatment group, were interviewed again two years after the second wave. We compare response rates between these two groups in the latest wave. Results We find a minimally higher response rate with a biennial survey – but without statistical significance. Thus, with a higher expected data quality, no (significant) losses in terms of response seem to be expected if the survey frequency is increased from biennial to annual. Added Value Our results serve as a guideline for survey makers on how to implement online panel surveys aiming for the sweet spot between optimized contact strategies, response burden, and high quality online panel data. |
2:30pm - 3:30pm | P 1.2: Postersession Location: Auditorium (Room 0.09/0.10/0.11) |
|
Digitalisation: Catalyzing the Transition to a Circular Economy in Ukraine Centre for Advanced Internet Studies, Germany Relevance & Research Question Digitalization can contribute to the shift towards a sustainable circular economy (CE). Digitalization not only refines business processes but also emphasizes waste curtailment, prolonging product life, and slashing transaction costs. However, fully leveraging this integration presents challenges, with clear gaps hindering the fluid adoption of digital-backed circular business models. Despite its significance, there's a dearth of comprehensive literature on digitalization's potential and challenges. This research aims to explore the main benefits and obstacles of applying digitalization in CE business models in Ukraine, focusing on identifying opportunities and challenges and finding ways to overcome these hurdles. Methods & Data The study involved interviews with business representatives, researchers, NGOs, and students (a total of 36 participants) during the thematic training course according to the Erasmus+ program in an online format in Ukraine. One of the activities during the training course was finding answers to four questions relevant to the research aim in small groups for 40 minutes for 7-8 participants. Results I identified challenges related to the integration of circular principles into existing business models, data ownership, data sharing, data integration, collaboration, and competence requirements. The post-war rebuilding and modernizing of industries towards sustainability, visualization, and innovation in product design, enhancement in resource efficiency, and optimization of logistics process collaboration with stakeholders and implementation of digital technologies were noted as main opportunities in adopting business models based on CE in the Ukrainian perspective. Added Value This research uncovered less recognized or previously unexplored prospects linked to digitalization in the context of transitioning to a CE. One of the new opportunities is virtualization in business models can influence on reduce costs, conserve resources, and provide reliable data. The research underscores the significant role of digitalization in enabling the transition towards a circular economy in Ukraine's business sector. While there are considerable opportunities for innovation and modernization, the challenges of integration, collaboration, data management, and skill gaps cannot be overlooked. Addressing these challenges through targeted educational programs, strategic partnerships, and supportive policies will be pivotal in harnessing the full potential of digitalization in advancing circular economy models. Device use in a face-to-face recruited neighborhood survey. University of Groningen, Netherlands, The Relevance & Research Question Due to the overall presence of smartphones and the ease of use of these devices, understanding the impact of device choice on survey data quality is becoming increasingly important. This study delves into the intricacies of a community survey conducted through both a paper flyer in the mail box and face-to-face recruitment by students. The primary objective is to explore the correlation between demographic characteristics and the selection of devices for survey completion. Additionally, the study investigates variations in data quality, measured through completion time and response patterns such as straightlining, acquiescence bias, and midpoint responding. Methods & Data The target population consisted of all 5475 residents of a neighborhood in Groningen, living in 4035 households. In December 2023, a total of 3500 flyers were distributed to every address that was recognized as a home address with a separate mail box. Subsequently, students visited homes, encouraging residents to participate in the survey. Students referred to the flyer delivered in the mail box, but presented residents with a new flyer in case the flyer got lost. Participants were given the option to engage via a QR code (i.e., completion on a smartphone) or a concise URL (i.e., completion on a pc), with a sweet incentive of a cake as compensation for their contribution. Results Within two weeks, 605 residents completed the questionnaire, resulting in a response rate of 17%. Notably, the QR code emerged as the preferred method for survey completion, with 85% opting for it, while the URL accounted for 15%. Interestingly, both students and individuals aged over 65 demonstrated a higher likelihood of using the URL. However, no significant associations were uncovered between completion time and the type of device chosen for survey participation. Added Value This study boasts a unique inclusion of all addresses within a single neighborhood in the recruitment sample, transforming it into a comprehensive population survey. In addition, in this study door-to-door recruitment and use of flyers that respondents use to decide their type of device distinguishes this study from earlier work. |
2:30pm - 3:30pm | P 1.3: Postersession Location: Auditorium (Room 0.09/0.10/0.11) |
|
Long Term Attrition and Sample Composition Over Time: 11 Years of the German Internet Panel University of Mannheim, Germany Relevance & Research Question For longitudinal research and panel practitioners, it is important to understand how much attrition to expect over time and which groups of respondents are especially at risk. These insights aid in guiding researchers in determining how many respondents to recruit, when to refresh the sample and which respondents should be especially targeted with strategies for improving recruitment rates or reducing attrition. SampcompR: A new R-Package for Sample Comparisons and Bias Analyses GESIS - Leibnitz Institute for Social Sciences, Germany Relevance & Research Question The steady trend in declining response rates and the rise of non-probability surveys makes it increasingly important to conduct nonresponse and selection bias analyses for social science surveys or conduct robustness checks to evaluate if the results are robust across population subgroups. Although this is important for any research project, it can be very time-consuming. The new R-Package SampcompR was created to provide easy-to-apply functions for those analyses and make it easier for any researcher to compare their survey against benchmark data for bias estimation on a univariate, bivariate, and multivariate level. Methods & Data To illustrate the functions of the package, we compare three web surveys conducted in Africa in March 2023 using Meta advertisements as a recruitment method (Ghana n = 527, Kenya n = 2,843, and South Africa n =313) to benchmarks from the cross-national Demographics and Health Survey (DHS). The benchmarks will be socio-demographics and health-related variables such as HIV knowledge. In univariate comparison, bias is measured as the relative bias for every variable and, on an aggregated level, the average absolute relative bias (AARB). In bivariate estimation, we compare Pearson’s r values against each other, and in multivariate comparison, different regression models are compared against each other. Results Our poster will show examples of output from the package, including visualizations and tables for each comparison level. While the focus will be on figures, tables can also be useful for documentation and more detailed inspection. As to the specific content of our example, we will see that the social media surveys show a high amount of bias on a univariate level. In contrast, the bias is less pronounced on a bivariate or multivariate level. We will also report country differences in sample accuracy. Added Value Our R-Package will provide an easy-to-use toolkit to perform bias analyses and survey comparisons and, therefore, will be a valuable tool in the social research workflow. Using the same or similar procedures and visualizations for the various comparisons will increase comparability and standardization. The visualization is based on the commonly used R-package ggplot2, making it easily customizable.
|
2:30pm - 3:30pm | P 1.4: Postersession Location: Auditorium (Room 0.09/0.10/0.11) |
|
Ask a Llama - Creating variance in synthetic survey data GESIS-Leibniz-Institut für Sozialwissenschaften in Mannheim, Germany Relevance & Research Question: Recently there has been a growth of research on whether Large Language Models (LLM) can be a source for high quality synthetic survey data. However, research has shown that synthetic survey data produced by LLMs underestimates the variational and correlational patterns that exist in human data. Additionally, the process of creating synthetic survey data with LLMs inherently has a lot of researcher’s degrees of freedom which can impact the distribution of the synthetic survey data. In this study we assess the problem of underestimated (co-)variance by systematically varying three factors and observe their impact on synthetic survey data: (1) The number and type of covariates a LLM sees before answering a question, (2) the model used to create the synthetic survey data and (3) the way we extract responses from the model. Methods & Data: We use five socio-demographic background questions and seven substantive questions from the 2018 German General Social Survey as covariates to have the LLM predict one substantive outcome, the satisfaction of the respondent with the government. To predict responses to the target question we use LLama2 in its chat and non-chat variant, as well as two versions finetuned on German text data to control for differences between LLMs. Results: First results show that the (co-)variance in synthetic survey data changes depending on (1) the type and quantity of covariates the model sees, (2) the model used to generate the responses and (3) whether we simulate from the model implied probability distribution or only look at the most likely response option. Especially (3), simulating from the model implied probability distribution, improves the estimation of standard deviations. Covariances estimates, however, remain underestimated. Added Value: We add value in three ways: (1) We provide information on which factors impact variance in synthetic survey data. (2) By creating German synthetic survey data, we can compare findings with results from research that has mostly focused on survey data from the US. (3) We show that using open-source LLMs enables researchers to obtain more information from the models than relying on closed-source APIs. To Share or Not to Share? Analyzing Survey Responses on Smartphone Sensor Data Sharing through Text Mining. Statistics Netherlands, Netherlands, The Relevance & Research Question In 2019, Statistics Netherlands (CBS) conducted the consent survey, inviting respondents to share various types of smartphone data, including location, personal photos and videos, and purchase receipts. The survey particularly focused on understanding the reasons behind the reluctance to share this data. This study explores the following research question: What classifications of motivations and sentiments can be identified for unwillingness to share data with CBS, using a data-driven text mining approach? Methods & Data This research applies multiple text mining techniques to detect underlying sentiments and motivations for not sharing sensor measurements with CBS. The manually classified responses from the survey serve as valuable training and test data for our text mining algorithms. Our findings provide a comprehensive comparison and validation of manual and automated classification methods, offering insights into the effectiveness of text mining. Added Value The study underscores the potential of text mining as an additional tool for analyzing open-text responses in survey research. By using this technique, we detect sentiments and motivations, enhancing the understanding of respondents’ perspectives on data sharing. This approach not only contributes to applying text mining in understanding attitudes towards data privacy and consent, but expands the methodology of survey research for analyzing open-ended questions and text data in general. |
2:30pm - 3:30pm | P 1.5: Postersession Location: Auditorium (Room 0.09/0.10/0.11) |
|
The AI Reviewer: Exploring the Potential of Large Language Models in Scientific Research Evaluation Bielefeld University, Germany Relevance & Research Question The advent of large language models (LLMs) has introduced the potential to automate routine tasks across various professions, including the academic field. This case study explores the feasibility of employing LLMs to reduce the workload of researchers by performing simple scientific review tasks. Specifically, it addresses the question: Can LLMs complete simple reviewer tasks to the same degree as real researchers? Methods & Data We utilized original text data from abstracts submitted to the GOR 2024 conference, along with multiple reviewer assessments (i.e., numeric scores) for each abstract. In addition, we used ChatGPT 4 to generate several AI reviewer scores for each abstract. The ChatGPT model was specifically instructed to mimic the GOR conference review criteria applied by the scientific reviewers, focusing on the quality of research, relevance to the scientific field, and alignment with the conference’s focus. This approach allows us to compare multiple AI assessments with multiple peer-review assessments for each abstract. Results Our results indicate that ChatGPT can quickly and comprehensively evaluate conference abstracts, with ratings slightly higher, i.e. on average more positive, than those of academic reviewers, while retaining a similar variance. Added Value This case study contributes to the ongoing discourse on the integration of AI in academic workflows by demonstrating that LLMs, like ChatGPT, can potentially reduce the burden on researchers and organizers when handling a large set of scientific contributions. Can socially desirable responding be reduced with unipolar response scales? University of Iceland, Iceland Relevance & Research Question It is well-known that the presentation and length of response scales can affect responses to questionnaire items. However, less is known about how different response scales affect responses and what the possible underlying mechanisms are. The purpose of this study was to compare bipolar and unipolar scales using a measure of personality (HEXACO-60) with regard to changes is response distributions, social desirability and acquiescence.
Methods & Data Four versions of the HEXACO-60 personality questionnaire were administered online via MTurk to 1,000 participants, randomly assigned to one of four groups, each containing one of the four versions. The first group received the HEXACO with its original response options (a five-point bipolar response scale), the second group received the HEXACO with a five-point unipolar agreement response scale, and the third group also received a unipolar agreement response scale but with three response options (the original response scale without the disagree response options). The fourth group was asked to rate the social desirability scale value (SDSV) of each of the 60 HEXACO items on a seven-point response scale (from very undesirable to very desirable). An index of item desirability was created from the SDSV and a measure of acquiescence was created by selecting items with incompatible content from the HEXACO to produce item pairs where agreement to both items would indicate acquiescence.
Results The three versions of the HEXACO-60 were analyzed with regard to distributions, social desirability of item content and acquiescence. The results show differences in the distribution of responses between the three response scales. Compared to the bipolar scale, the unipolar scales increased agreement with items rated as undesirable, which would indicate less socially desirable responding on unipolar scales. However, the use of unipolar scales increased overall agreement to items, which could indicate either increased acquiescence or different interpretations of the question in relation to the response options. The results and possible interpretations will be discussed. Added Value The study provides added understanding of the effects of changing response scales from bipolar to unipolar and aids in understanding of the mechanisms underlying responses.
|
3:45pm - 4:45pm | D3: Virtual Respondents and Audiences - Is This the Future of Survey Research? (organised by marktforschung.de) Location: Auditorium (Room 0.09/0.10/0.11) Session Chair: Holger Geissler, marktforschung.de, Germany Panelists: Dirk Held, Co-Founder & Managing Director of DECODE Marketing and Co-Founder of Aimpower Louise Leitsch, Director Research of Appinio Frank Buckler, Founder & CEO of Success Drivers & Supra Tools Florian Kögl, Founder & CEO of ReDem |
5:00pm - 6:00pm | D4: Wissenschaft trifft Praxis. Wann ist eine Online-Stichprobe gut für welchen Bedarf. Location: Auditorium (Room 0.09/0.10/0.11) Session Chair: Otto Hellwig, Bilendi & respondi, Germany Impulse von: Dr. Carina Cornesse (German Institute for Economic Research, Germany & DGOF Vorstand) Menno Smid (Vorstandsvorsitzender (CEO) bei Infas Holding AG) Weitere Diskutanten: Beate Waibel-Flanz (Business Insights - Market research Manager bei REWE GROUP & stellv. Sprecherin des BVM Regionalrates) Dr. Barbara Felderer (Teamleiterin Survey Design & Methodology Survey Statistics bei Gesis) |
|
Wann ist eine Stichprobe “fit-for-purpose”? German Institute for Economic Research, Germany In den Medien werden immer wieder Fälle von Studienerkenntnissen diskutiert, die sich bei genauerer Betrachtung als falsch herausstellen. Nicht selten ist der Grund die Datenbasis, welche die proklamierten Schlüsse aufgrund der Selektivität der Stichprobe nicht zulässt. Dies betrifft in besonderem Maße Studien, die von nicht-zufallsbasierten Online-Stichproben auf die deutsche Gesamtbevölkerung schließen wollen. Diese Rückschlüsse beruhen in der Regel auf Annahmen, die weder explizit kommuniziert werden, noch in vielen Fällen haltbar sind. Die Stichproben stellen sich dann also als nicht geeignet für den Zweck heraus, den sie erfüllen sollen und sind somit nicht “fit-for-purpose”. Dieser Impulsvortrag beschreibt die Annahmen, die Inferenzschlüssen basierend auf Nicht-Zufallsstichproben zugrunde liegen und diskutiert Umstände, unter denen diese Annahmen halten können. Der Fokus liegt dabei auf der Frage, ob (und wenn ja wann) eine (hoch)selektive nicht-probabilistische Online-Stichprobe fitness-for-purpose für einen bestimmten Forschungszweck aufweisen kann. |
Date: Friday, 23/Feb/2024 | |
10:00am - 10:45am | Keynote 2: Keynote 2 Location: Auditorium (Room 0.09/0.10/0.11) |
|
Data collection using mobile apps: What can we do to increase participation? University of Essex, United Kingdom There are limits to what can be measured with survey questions: we can only collect information about things our respondents know, can recall, are willing to tell us – and that fit within a time-constrained questionnaire. Increases in smartphone ownership and use, along with technological changes are creating new possibilities to collect data for surveys of the general population, for example, through linkage or donation of existing digital data, collection of bio-samples or -measures, or use of sensors and trackers. Surveys are therefore developing into systems of data collection: depending on the concept of interest, different methods are used to generate data of the required level of accuracy, granularity, and periodicity. For example, Understanding Society: the UK Household Longitudinal Study supplements the annual questionnaire-based data with linked data and data derived from bio measures and bio samples. In addition, we are developing and testing protocols to collect data using mobile applications, activity and GPS trackers and air quality sensors. We have conducted a series of mobile app studies, collecting detailed information about household expenditure, daily data about relationships, stressors and wellbeing, detailed body measurements, and spatial cognition. However, in each case, only a sub-set of respondents invited to the mobile app study participated and provided data. In this talk I will present research from a series of experimental studies carried out on the Understanding Society Innovation Panel, that aim to identify the barriers faced by respondents in participating in mobile app studies, provide evidence on how best to design data collection protocols to maximise participation and reduce selectiveness of participants, and examine the quality of data collected with mobile apps. |
11:45am - 12:45pm | D5: KI Forum: Impuls-Session - Chancen und Regulierungen Location: Auditorium (Room 0.09/0.10/0.11) Session Moderators: Oliver Tabino, Q Agentur für Forschung Yannick Rieder, Janssen-Cilag GmbH Georg Wittenburg, Inspirient This session is in German. |
|
EU AI Act: Innovationsmotor oder Innovationsbremse? KI Bundesverband, Germany Der Artificial Intelligence Act (AI Act) der EU ist das erste Regelwerk, das sich mit der Regulierung von Künstlicher Intelligenz (KI) befasst. Mit dem AI Act will die EU einen weltweiten Goldstandard und eine Blaupause für die Regulierung von KI schaffen. Doch kann der AI Act tatsächlich zum Innovationsmotor für vertrauenswürdige KI werden oder wird er zum wirtschaftlichen Hemmschuh? Das Potential von Foundation Models und Generativer KI – Ein Blick in die Zukunft IAIS, Germany Foundation Models stehen im Zentrum des gegenwärtigen Hypes um (Generative) Künstliche Intelligenz. Sie besitzen das Potential, die Art und Weise, wie wir arbeiten, branchen- und aufgabenübergreifend zu revolutionieren.. Wir präsentieren ein aktuelles Projekt, in dem LLMs für personalisiertes Marketing genutzt werden und wagen einen Blick in die Zukunft von KI. Ein besonderer Fokus liegt auf der Rolle von Open Source in der Demokratisierung der KI-Technologie, dem Potenzial autonomer Agenten, die menschliche Arbeit unterstützen und ergänzen, sowie den Möglichkeiten, die Small Language Models für spezialisierte Anwendungen bieten. |
2:00pm - 3:00pm | D6: KI Forum: KI Café Location: Auditorium (Room 0.09/0.10/0.11) Session Moderators: Oliver Tabino, Q Agentur für Forschung Yannick Rieder, Janssen-Cilag GmbH Georg Wittenburg, Inspirient This session is in German. Moderierter Austausch zu folgenden Themen: • Messbare Qualität von KI-Tools ist Grundlage für Vertrauen und Voraussetzung für den betrieblichen Einsatz, aber welche Qualitätskriterien haben sich bewährt? Wie können sie erfasst und verglichen werden? • Wie implementiert man KI-Anwendungen in Prozesse? Wobei ist die Nutzung bereits etabliert? Was gibt es dabei zu beachten? • KI und Ethik: Was geht und was nicht? |
Contact and Legal Notice · Contact Address: Privacy Statement · Conference: GOR 24 |
Conference Software: ConfTool Pro 2.8.101 © 2001–2024 by Dr. H. Weinreich, Hamburg, Germany |