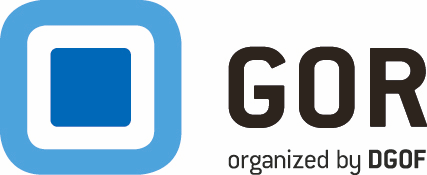
General Online Research Conference 2024 (GOR 24)
Rheinische Fachhochschule Cologne - Campus Vogelsanger Straße
21 - 23 February 2024
Conference Agenda
Overview and details of the sessions of this conference. Please select a date or location to show only sessions at that day or location. Please select a single session for detailed view (with abstracts and downloads if available).
|
Session Overview |
Date: Friday, 23/Feb/2024 | |
9:30am - 10:00am | Begin Check-in |
10:00am - 10:45am | Keynote 2: Keynote 2 Location: Auditorium (Room 0.09/0.10/0.11) |
|
Data collection using mobile apps: What can we do to increase participation? University of Essex, United Kingdom There are limits to what can be measured with survey questions: we can only collect information about things our respondents know, can recall, are willing to tell us – and that fit within a time-constrained questionnaire. Increases in smartphone ownership and use, along with technological changes are creating new possibilities to collect data for surveys of the general population, for example, through linkage or donation of existing digital data, collection of bio-samples or -measures, or use of sensors and trackers. Surveys are therefore developing into systems of data collection: depending on the concept of interest, different methods are used to generate data of the required level of accuracy, granularity, and periodicity. For example, Understanding Society: the UK Household Longitudinal Study supplements the annual questionnaire-based data with linked data and data derived from bio measures and bio samples. In addition, we are developing and testing protocols to collect data using mobile applications, activity and GPS trackers and air quality sensors. We have conducted a series of mobile app studies, collecting detailed information about household expenditure, daily data about relationships, stressors and wellbeing, detailed body measurements, and spatial cognition. However, in each case, only a sub-set of respondents invited to the mobile app study participated and provided data. In this talk I will present research from a series of experimental studies carried out on the Understanding Society Innovation Panel, that aim to identify the barriers faced by respondents in participating in mobile app studies, provide evidence on how best to design data collection protocols to maximise participation and reduce selectiveness of participants, and examine the quality of data collected with mobile apps. |
10:45am - 11:15am | GOR Award Ceremony |
11:15am - 11:45am | Break |
11:45am | Track A.1: Survey Research: Advancements in Online and Mobile Web Surveys sponsored by GESIS – Leibniz-Institut für Sozialwissenschaften |
11:45am | Track A.2: Survey Research: Advancements in Online and Mobile Web Surveys sponsored by GESIS – Leibniz-Institut für Sozialwissenschaften |
11:45am | Track B: Data Science: From Big Data to Smart Data |
11:45am | Track C: Politics, Public Opinion, and Communication |
11:45am | Track D: Digital Methods in Applied Research |
11:45am - 12:45pm | A5.1: Recruiting Survey Participants Location: Seminar 1 (Room 1.01) Session Chair: Olga Maslovskaya, University of Southampton, United Kingdom |
|
Recruiting online panel through face-to-face and push-to-web surveys. HUN-REN Centre for Social Sciences, Hungary Relevance & Research Question: This presentation focuses on the difficulties and solutions related to recruiting web panels through probability-based face-to-face and push-to-web surveys. It also compares the panel composition when using two different survey modes for recruitment. Methods & Data: As part of the ESS SUSTAIN-2 project, a webpanel was recruited in 2021/22 through a face-to-face survey of ESS R10 in 12 countries. Unfortunately, the recruitment rate was low and the sample size achieved in Hungary was inadequate for further analysis. To increase the size of the webpanel (CRONOS-2), the Hungarian team initiated a probability-based mixed-mode self-completion survey (push-to-web design). Respondents were sent a post inviting them to go online or complete a questionnaire, which was identical to the interviewer-assisted ESS R10 survey. Results: We will present our findings on how the type of survey affects recruitment to a web panel through probability sampling. We will begin by introducing the design of the two surveys, then discuss the challenges encountered in setting up the panel, and finally compare the composition of the panel recruited through the two surveys (interviewer-assisted ESS R10 and push-to-web survey with self-completion). Our research provides valuable insight into how the type of survey and social and political environment affect recruitment to a web panel. Added Value: This analysis focuses on the mode effect on the recruitment of participants for a scientific research panel. Our findings highlight the effect of the social and political environment, which could be used as a source of inspiration for other local studies. Initiating Chain-Referral for Virtual Respondent-Driven Sampling – A Pilot Study with Experiments 1German Institute for Economic Research; 2University of Bremen; 3German Center for Integration and Migration Relevance & Research Question RDS is a network sampling technique for surveying complex populations in the absence of sampling frames. The idea is simple: identify some people (“seeds”) who belong or have access to the target population, encourage them to start a survey invitation chain-referral process in their community, ensure that every respondent can be traced back along the referral chain. But who will recruit? And whom? And which strategies help initiate the referral process? Methods & Data We conducted a pilot study in 2023 where we invited 5,000 panel study members to a multi-topic online survey. During the survey, we asked respondents whether they would be willing to recruit up to three of their network members. If they agreed, we asked them about their relationship with those network members as well as these people’s ages, gender, and education and provided unique survey invitation links to be shared virtually. As part of the study, we experimentally varied the RDS consent wording, information layout, and survey link sharing options. We also applied a dual incentive scheme, rewarding seeds as well as recruits. Results Overall, 624 initial respondents (27%) were willing to invite network members. They recruited 782 people (i.e., on average 1.25 people per seed). Recruits were mostly invited via email (46%) or WhatsApp (43%) and belonged to the seeds’ family (53%) and friends (38%). Only 20% of recruits are in contact with the seed less than once a week, suggesting recruitment mostly among close ties. We find an adequate gender balance (52% female) and representation of people with migration background (22%) in our data, but a high share of people with college or university degrees (52%) and high median age (52 years). The impact of the experimental design on recruitment success is negligible. Added Value While in theory, RDS is a promising procedure, it often fails in practice. Among other challenges, this is commonly due to the fact that seeds will not or only insufficiently start the chain-referral process. Our project shows in which target groups initiating RDS may work and to what extent UX enhancements may increase RDS success. |
11:45am - 12:45pm | A5.2: Detecting Undesirable Response Behavior Location: Seminar 3 (Room 1.03/1.04) Session Chair: Jan-Lucas Schanze, GESIS - Leibniz-Institut für Sozialwissenschaften, Germany |
|
Who is going back and why? Using survey navigation paradata to differentiate between potential satisficers and optimizers in web surveys 1GESIS – Leibniz-Institut für Sozialwissenschaften in Mannheim, Germany; 2Utrecht University, Netherlands Relevance & Research Question: Survey navigation paradata presents a unique opportunity to delve into the web survey completion behavior of respondents, particularly actions like revisiting questions and potentially altering answers. Such behavior could be indicative of motivated misreporting, especially when respondents revisit filter or looping questions to modify answers and circumvent subsequent inquiries — a manifestation of satisficing behavior. Conversely, altering answers upon revisiting may also signify optimizing behavior, where respondents strive for utmost accuracy. This study focuses on the revisiting behavior of web survey respondents, aiming to quantify its frequency, identify associated respondent characteristics, and ascertain who shortens their questionnaire through revisiting. Methods & Data: Using paradata from the probability-based online-administered Generations and Gender Programme (GGP) survey in Estonia (N=8916), we analyze the frequency of revisiting questions, characteristics of these questions, and the ensuing actions. We investigate the connection between revisiting behavior and respondent characteristics using a zero-inflated Poisson regression model and check which respondents’ characteristics were connected with a higher proportion of shortening the questionnaire as a result of revisiting questions. Results: We find a discernible pattern of revisiting questions during the survey, notably prevalent in immediate filter questions, where almost half of respondents go back after a filter question (that can change the routing of the questionnaire). Added Value: This study contributes a nuanced understanding of respondents' behavior during web survey self-completion. Utilizing paradata enhances insights into respondents' survey completion patterns and various behavioral types, providing valuable insights for survey design and data quality management. Socially Desirable Responding in Panel Studies – Does Repeated Interviewing Affect Answers to Sensitive Behavioral Questions? GESIS - Leibniz Institute for the Social Sciences Relevance and Research Question: Social desirability (SD-) bias (i.e., the tendency to report socially desirable opinions and behaviors instead of revealing true ones) is a widely known threat to response quality and the validity of self-reports. Previous studies investigating socially desirable responding in a longitudinal context provide mixed evidence on whether SD-bias increases or decreases with repeated interviewing and how these changes affect response quality in later waves. However, most studies were non-experimental and only suggestive of the underlying mechanisms of observed changes in SD-bias over time. Methods and Data: This study investigates socially desirable responding in panel studies using a longitudinal survey experiment comprising six panel waves. The experiment manipulated the frequency of receiving identical sensitive questions (target questions) and assigned respondents to one of three groups: One group received the target questions in each wave (fully conditioned), the second group received the target questions in the last three waves (medium conditioned), and the control group received the target questions only in the last wave of the study (unconditioned). The experiment was conducted within a German non-probability (n = 1,946) and a probability-based panel study (n = 4,660), resulting in 2x3 experimental groups in total. The analysis focusses on between-group and within-group comparisons of different sensitive behavioral measures. It further includes measures on the questions’ degree of sensitivity as a moderating variable. These measures result from an additional survey (n = 237) in which respondents were asked to rate the sensitivity of multiple attitudinal and behavioral questions. To further examine the underlying mechanisms of change, I use a measure on respondents’ trust towards the survey (sponsor) and the scores of an established SD-scale.
Results: Results will be presented at the conference in February. Added Value: Altogether, this study provides experimental evidence on the impact of repeated interviewing on changes in social desirability bias. It further contributes to the understanding of what causes these changes by examining different levels of exposure to identical sensitive questions and including measures on respondents’ trust towards the survey (sponsor) and their scores on a SD-scale. Distinguishing satisficing and optimising web survey respondents using paradata GESIS – Leibniz-Institut für Sozialwissenschaften in Mannheim, Germany Relevance & Research Question This study seeks to investigate the interplay between different paradata types and data quality indicators derived from survey data, aiming to identify distinct patterns characterizing respondents' satisficing and optimizing behaviors. Methods & Data Employing a laboratory two-wave experiment with a crossover design involving 93 students, participants were randomly assigned to either satisficing or optimizing conditions in the first wave, with groups reversed in the second. Participants were asked to complete a web survey in either satidficing or in optimising manner. Manipulation checks were used to ensure participants' compliance with a condition. The survey encompassed open-ended, factual, and matrix questions, coupled with reliable scales gauging trust, values, and other sociological and psychological measures. Paradata, such as completion time, mouse movements, browser focus, reaction to warnings, scrolling, and resizing, were collected using the One Click Survey (1ka.si) online software. Added Value |
11:45am - 12:45pm | B5: To Trace or to Donate, That’s the Question Location: Seminar 2 (Room 1.02) Session Chair: Alexander Wenz, University of Mannheim, Germany |
|
Exploring the Viability of Data Donations for WhatsApp Chat Logs 1GESIS - Leibniz Institute for the Social Sciences; 2Ulm University Relevance & Research Question Data donations are a new tool for collecting research data. They can ensure informed consent, highly granular, retrospective, and potentially less biased behavioral traces, and are independent from APIs or webscraping pipelines. We thus seek to explore the viability of data donations for a type of highly personal data: WhatsApp chat logs. Specifically, we are exploring a wide range of demographic, psychological, and relational charactersitics and how they relate to peoples donation willingness, censoring, and actual data donation behavior. We used an opt-in survey assessing demographics, personality, relationship characteristics of a self-selected social relationship, and concerns for privacy. Participants were also asked whether they are willing to donate a WhatsApp chat from a 1:1 chat from the respective relationship. If they agreed, participants were forwarded to an online platform where they could securely upload, review, self-censor, and donate the chat log. Donated chats were anonimized automatically by first extracting variables of interest (e.g. number of words per message, emoji, smilies, sent domains, response time) and then deleting the raw message content. In a second step, participants selected which parts of the anonymized data should be included in the donations. The study was reviewed and accepted by the ethics committee of Ulm University. So far, 244 people participated in the survey and 140 chat log files with over 1 million messages in total were donated. Preliminary Results Preliminary results (based on 198 ppts.) show that participants were mostly university students. Self-indicated willingness to donate a chat was surprisingly high (73%), with a sizable gap to actual donations (39.4%). Interestingly participants rarely excluded any data manually after the automatic anonimization step. Furthermore, we did not find any meaningful differences in data donation willingness and behavior with respect to demographics, personality, privacy concerns, or relationship characteristics. Added Value The Mix Makes the Difference: Using Mobile Sensing Data to Foster the Understanding of Non-Compliance in Experience Sampling Studies 1Charlotte Fresenius Hochschule, University of Psychology, Germany; 2LMU Munich, Department of Psychology Relevance & Research Question For decades, social sciences have focused on broad one-time assessments and neglected the role of momentary experiences and behaviors. Now, novel digital tools facilitate the ambulatory collection of data on a moment-to-moment basis via experience sampling methods. But the compliance to answer short questionnaires in daily life varies considerably between and within participants. Compliance and consequently mechanisms leading to missing data in experience sampling studies, however, still remain in the dark today. In our study we therefore explored person-, context- and behavior-related patterns associated with participants’ compliance in experience sampling studies. Methods & Data We used a data set part (N = 592) of the Smartphone Sensing Panel Study recruited according to quotas representing the German population. We extracted over 400 different person-, context-, and behavior-related variables by combining assessments from traditional surveys (e.g., personality traits), experience sampling (e.g., mood), and passively collected mobile sensing data (e.g., smartphone usage, GPS). Based on more than 25,000 observations, we predicted participants' compliance to answer experience sampling questionnaires. For this purpose, we used a machine learning based modeling approach and benchmarked different classification algorithms using 10-fold cross-validation. In addition, we applied methods from interpretable machine learning to better understand the importance of single variables and constellations of variable groups. Results We found that compliance to experience sampling questionnaires could be successfully predicted above chance and that among the compared algorithms the linear elastic net model performed best (MAUC = 0.723). Our follow-up analysis showed that study-related past behaviors such as the average response rate to previous experience sampling questionnaires were the most informative, followed by location information such “at home” or “at work”. Added Value Our study shows that compliance in experience sampling studies is related to participants' behavioral and situational context. Accordingly, we illustrate systematic patterns associated with missing data. Our study is an empirical starting point for discussing the design of experience sampling studies in social sciences and for pointing out future directions in research addressing experience sampling methodology and missing data. |
11:45am - 12:45pm | C5: Politics, Media, Trust Location: Seminar 4 (Room 1.11) Session Chair: Felix Gaisbauer, Weizenbaum-Institut e.V., Germany |
|
What makes media contents credible? A survey experiment on the relative importance of visual layout, objective quality and confirmation bias for public opinion formation Konstanz University, Germany Relevance & Research Question The emergence of social media has transformed the way people consume and share information. As such platforms widely lack mechanisms to ensure content quality, their increasing popularity has raised concerns about the spread of fake news and conspiracy beliefs – with potentially harmful effects on public opinion and social cohesion. Our research aims to understand the underlying mechanisms of media perception and sharing behaviour when people are confronted with factual vs conspiracy-based media contents. Under which circumstances do people believe in a media content? Do traditional indicators of quality matter? Are pre-existing views more important than quality (confirmation bias)? How is perceived credibility linked to sharing behaviour? Methods & Data To empirically assess these questions, we administered a survey experiment to a general population sample in Germany via Bilendi in August 2023. As respondents with a general susceptibility to conspiracy beliefs are of major substantive interest, we made use of responses from a previous survey to oversample “conspiracy thinkers”. Respondents were asked to evaluate the credibility of different media contents related to three vividly debated topics: vaccines against Covid-19, the climate crisis, and the Ukraine war. We analyze these evaluations regarding the objective quality of the content (measured by author identity and data source), its visual layout (newspaper vs tweet), and previous respondent beliefs on the respective topic to measure confirmation bias. Results Our findings suggest that the inclination to confirm pre-existing beliefs is the most important predictor for believing a media content, irrespective of its objective quality. This general tendency applies to both, the mainstream society and “conspiracy thinkers”. However, according to self-reports, the latter group is much more likely to share media contents they believe in. Added Value Methodologically, we use an interesting survey experiment that allows us to vary opinion (in)consistency and objective quality of media contents simultaneously, meaning that we can estimate the relative effect of these features on the credibility of media contents. We provide insights into the underlying mechanisms of the often debated spread of conspiracy beliefs through online platforms, with their practical implications for public opinion formation. Sharing is caring! Youth Political Participation in the Digital Age GESIS, Germany Relevance & Research Question Navigating Political Turbulence: A Study of Trust and online / offline Engagement in Unstable Political Contexts The Max Stern Yezreel Valley College, Israel Relevance & Research Question: Within the backdrop of Israel's turbulent 2022 elections, the fifth round of elections within three years, This study delves into the complex interplay between political trust, efficacy, and engagement. It seeks to unravel how individuals' trust in politicians and the political system, coupled with their sense of political efficacy, influences their online and offline engagement in the political process. The research question focuses on identifying the specific predictors of political engagement in a context characterized by political unpredictability and frequent elections. Methods & Data: The study analyzes a representative survey of 530 Israeli respondents during the 2022 Israeli election period. The research evaluates the influence of various variables. These include trust in politicians, the political system, and political efficacy in online and offline political engagement. The analysis focuses on the differentiation between online engagement, such as social media activity, and offline engagement, like attending rallies or voting. Results: Statistical analysis reveals a robust correlation between political efficacy and both forms of political engagement (r = .62 for online, r = .57 for offline, p < .01). Trust in the political system emerges as a significant predictor of offline engagement (β = .36, p < .01), while trust in politicians is more strongly associated with online engagement (β = .41, p < .01). Notably, a mediation analysis indicates that political efficacy serves as a mediator in the relationship between trust in politicians and online engagement (indirect effect = 0.15, 95% CI [0.07, 0.24], p < .01). In contrast, such mediating effects between system trust and offline engagement are not observed. Added Value: By examining the nuanced factors influencing political engagement during political uncertainty, this study offers new insights into the differentiated impact of trust in politicians and the political system. It underscores the distinct psychological pathways that drive online and offline political engagement, enhancing our understanding of citizen behavior in democracies facing political instability. These findings have critical implications for political strategists, policymakers, and scholars seeking to foster civic engagement in similar contexts. |
11:45am - 12:45pm | D5: KI Forum: Impuls-Session - Chancen und Regulierungen Location: Auditorium (Room 0.09/0.10/0.11) Session Moderators: Oliver Tabino, Q Agentur für Forschung Yannick Rieder, Janssen-Cilag GmbH Georg Wittenburg, Inspirient This session is in German. |
|
EU AI Act: Innovationsmotor oder Innovationsbremse? KI Bundesverband, Germany Der Artificial Intelligence Act (AI Act) der EU ist das erste Regelwerk, das sich mit der Regulierung von Künstlicher Intelligenz (KI) befasst. Mit dem AI Act will die EU einen weltweiten Goldstandard und eine Blaupause für die Regulierung von KI schaffen. Doch kann der AI Act tatsächlich zum Innovationsmotor für vertrauenswürdige KI werden oder wird er zum wirtschaftlichen Hemmschuh? Das Potential von Foundation Models und Generativer KI – Ein Blick in die Zukunft IAIS, Germany Foundation Models stehen im Zentrum des gegenwärtigen Hypes um (Generative) Künstliche Intelligenz. Sie besitzen das Potential, die Art und Weise, wie wir arbeiten, branchen- und aufgabenübergreifend zu revolutionieren.. Wir präsentieren ein aktuelles Projekt, in dem LLMs für personalisiertes Marketing genutzt werden und wagen einen Blick in die Zukunft von KI. Ein besonderer Fokus liegt auf der Rolle von Open Source in der Demokratisierung der KI-Technologie, dem Potenzial autonomer Agenten, die menschliche Arbeit unterstützen und ergänzen, sowie den Möglichkeiten, die Small Language Models für spezialisierte Anwendungen bieten. |
12:45pm - 2:00pm | Lunch Break Location: Cafeteria (Room 0.15) |
2:00pm - 3:00pm | A6.1: Questionnaire Design Choices Location: Seminar 1 (Room 1.01) Session Chair: Julian B. Axenfeld, German Institute for Economic Research (DIW Berlin), Germany |
|
Grid design in mixed device surveys: an experiment comparing four grid designs in a general Dutch population survey. Statistics Netherlands, Netherlands, The Relevance & Research Question Within the current stylesheet, half of the sample units were randomly assigned to the standard grid design as currently used (a table format for large screens and a stem-fixed vertical scrollable format for small screens) and the other half to a general stem-fixed grid design (stem-fixed design for both the large and the small screen). Within the experimental stylesheet, one third of the sample was randomly assigned to either the general stem-fix grid design, a carrousel grid design (in which only one item is displayed at the time and after answering one item, the next item automatically ‘flies in‘) or an accordion grid design (all items are presented vertically on one page, and answer options are automatically closed and unfolded after an item is answered). Various indicators are used to assess response quality, e.g. break-off, item non response, straightlining, mid-point reporting. Respondent satisfaction is assessed with a set of evaluation questions at the end of the questionnaire. Results Data are currently being analyzed.
Towards a mobile web questionnaire for the Vacation Survey: UX design challenges Statistics Netherlands, Netherlands, The Towards a mobile web questionnaire for the Vacation Survey: UX design challenges Vivian Meertens & Maaike Kompier Key words: Mobile Web Questionnaire Design, Smartphone First Design, Vacation Survey, Statistics Netherlands, UX testing, Qualitative Approach, Mixed Device Surveys Relevance & Research Question: —your text here— Despite the fact that online surveys are not always fit for small screens and mobile device navigation, the number of respondents that start online surveys on mobile devices instead of PC or laptop device, is still growing. Statistics Netherlands (CBS) has responded to this trend by developing and designing mixed device surveys. This study focuses on the redesign of the Vacation Survey, applying a smartphone first approach. The Vacation Survey is a web only panel survey, that could only be completed on a PC or laptop. The layered design with a master detail approach was formatted in such a way that a large screen was needed to be able to complete the questionnaire. Despite a warning in the invitation letter that a PC or laptop should be used to complete the questionnaire, 14.5% of first-time logins in 2023 were via smartphones, resulting in a redesign with a smartphone first approach. The study examines the applicability and understandability of the Vacation Survey’s layered design, specifically its master-detail approach, from a user experience (UX) design perspective. Results: —your text here— Added Value: —your text here Optimising recall-based travel diaries: Lessons from the design of the Wales National Travel Survey National Centre for Social Research, United Kingdom Relevance & Research Question: Recall-based travel diaries require respondents to report their travel behaviour over a period ranging from one to seven days. During this period, they are asked to indicate the start and end times and locations, modes of transport, distances, and the number of people on each trip. Depending on the mode, additional questions are asked to gather information on ticket types and costs or fuel types. Due to the specificity of the requested information and its non-centrality for most respondents, travel diaries pose a substantial burden, increasing the risk of satisficing behaviours and trip underreporting. Methods & Data: In this presentation, we describe key decisions made during the design of the Wales National Travel Survey. This push-to-web project includes a questionnaire and a 2-day travel diary programmed into the survey. Results: Critical aspects of these decisions include the focus of the recall (trip, activity, or location based) and the sequence of follow-up questions (interleaved vs. roster approach). Recent literature suggests that location-based diaries align better with respondents’ cognitive processes than trip-based diaries and help reduce underreporting. Therefore, a location-based travel diary was proposed with an auto-complete field to match inputs with known addresses or postcodes. Interactive maps were also proposed for user testing. While they can be particularly useful when respondents have difficulty describing locations or when places lack formal addresses, previous research warns that advanced diary features can increase drop-off rates. Regarding the follow-up sequence, due to mixed findings in the literature and limited information on the performance of these approaches in web-based travel diaries, experimentation is planned to understand how each approach performs in terms of the accuracy of the filter questions and the follow-up questions. Additionally, this presentation discusses the challenges and options for gathering distance data in recall-based travel diaries, along with learnings from the early phases of diary testing based on the application of a Questionnaire Appraisal System and cognitive/usability interviews. Added Value: These findings offer valuable insights into the design of complex web-based surveys with multiple loops and non-standard features, extending beyond travel diaries. |
2:00pm - 3:00pm | A6.2: Data Quality Assessments 2 Location: Seminar 3 (Room 1.03/1.04) Session Chair: Fabienne Kraemer, GESIS Leibniz-Institut für Sozialwissenschaften, Germany |
|
Can we identify and prevent cheating in online surveys? Evidence from a web tracking experiment. 1University of Oxford, United Kingdom; 2The London School of Economics, United Kingdom; 3Universitat Pompeu Fabra, Spain; 4Institut Barcelona Estudis Internacionals (IBEI), Spain Relevance & Research Question: Survey measures of political knowledge, widely used in political science research, face challenges in online administration due to potential cheating. Previous research reveals a significant proportion of participants resort to online searches when answering political knowledge questions, casting doubt on measurement quality. Existing studies testing potential interventions to curb cheating have relied on indirect measures of cheating, such as catch questions. This study introduces a novel approach, employing direct observations of participants' Internet browsing via web trackers, combined with an experimental design testing two strategies to prevent cheating (instructions and time limit). The paper explores three research questions: what proportion of participants looks up information when posed political knowledge questions (RQ.1)? What is the impact of the interventions on the likelihood of individuals looking up information (RQ.2)? How do estimates from direct observations differ from indirect proxies (e.g., self-reports, paradata) (RQ.3)? A web survey experiment (N = 1,200) in Spain was deployed within an opt-in access online panel. Cross quotas for age and gender, and quotas for educational level, and region were used to ensure a sample matching on these variables to the Internet adult population. Participants answered six knowledge questions on political facts and current events. Cheating was identified by analysing URLs from web tracking data, and alternative indirect measures were applied, including catch questions, self-reports, and paradata. Two noteworthy patterns emerge. Firstly, cheating prevalence from web tracking data is below 5%, markedly smaller than levels estimated by indirect measures (2 to 7 times larger). Secondly, based on web tracking data the anti-cheating interventions have no effect. Nonetheless, using indirect measures of cheating we find that both interventions significantly reduce the likelihood of cheating. This study pioneers the integration of web tracking data and experimental design to examine cheating in online political knowledge assessments. Despite requiring further validation, the substantial differences between web tracking data and indirect approaches suggest two competing conclusion: either cheating in online surveys is substantially lower than first thought, or web tracking data may not be suitable for identifying cheating in online surveys. The Quality of Survey Items and the Integration of the Survey Quality Predictor 3.0 into the Questionnaire Development Process GESIS - Leibniz Institute for the Social Sciences, Germany Relevance & Research Question Probability-based online and mixed-method panels from a data quality perspective 1HUN-REN Centre for Social Sciences, Hungary; 2Panelstory Opinion Polls, Hungary Relevance & Research Question: Probability-based online and mixed-method panels are widely used in scientific research, but not as much for market research or political opinion polling. This presentation will explore the case of "Panelstory", the first Hungarian probability-based mixed-method panel, which was established in 2022 with the purpose of utilizing scientific methods to address market research and political opinion polling issues. |
2:00pm - 3:00pm | B6.1: Automatic analysis of answers to open-ended questions in surveys Location: Seminar 2 (Room 1.02) Session Chair: Barbara Felderer, GESIS, Germany |
|
Using the Large Language Model BERT to categorize open-ended responses to the "most important political problem" in the German Longitudinal Election Study (GLES) GESIS, Germany Relevance & Research Question Open-ended survey questions are crucial e.g., for capturing unpredictable trends, but the resulting unstructured text data poses challenges. Quantitative usability requires categorization, a labor-intensive process in terms of costs and time, especially with large datasets. In the case of the German Longitudinal Election Study (GLES) spanning from 2018 to 2022, with nearly 400,000 uncoded mentions, it prompted us to explore new ways of coding. Our objective was to test various machine learning approaches to determine the most efficient and cost-effective method for creating a long-term solution for coding responses, ensuring high quality simultaneously. Which approach is best suited for the long-term coding of open-ended mentions regarding the "most important political problem" in the GLES? Methods & Data Pre-2018, GLES data was manually coded. Shifting to a (partially) automated process involved revising the codebook. Subsequently, the extensive dataset comprising nearly 400,000 open responses to the question regarding the "most important political problem" in the GLES surveys conducted between 2018 and 2022 was employed. The coding process was facilitated using the Large Language Model BERT (Bidirectional Encoder Representations from Transformers). During the entire process, we tested a whole host of important aspects (hyperparameter finetuning, downsizing of the “other” category, simulations of different amounts of training data, quality control of different survey modes, using training data from 2017) before arriving at the final implementation. The "new" codebook already demonstrates high quality and consistency, evident from its Fleiss Kappa value of 0.90 for the matching of individual codes. Utilizing this refined codebook as a foundation, 43,000 mentions were manually coded, serving as the training dataset for BERT. The final implementation of coding for the extensive dataset of almost 400,000 mentions using BERT yields excellent results, with a 0/1 loss of 0.069, a Micro F1 score of 0.946 and a Macro F1 score of 0.878. The outcomes highlight the efficacy of the (partially) automated coding approach, emphasizing accuracy with the refined codebook and BERT's robust performance. This strategic shift towards advanced language models signifies an innovative departure from traditional manual methods, emphasizing efficiency in the coding process. The Genesis of Systematic Analysis Methods Using AI: An Explorative Case Study TU Dresden, Germany Relevance & Research Question The analysis of open-ended questions in large-scale surveys can provide detailed insights into respondents' views that often can't be assessed with closed-ended questions. However, due to the large number of respondents, it takes a lot of resources to review the answers within open-ended questions and thus provide them as research results. This contribution aims to show the potential benefits and limitations of using AI-based tools (e.g. ChatGPT), for analyzing open-ended questions in large-scaled surveys. It therefore also aims to highlight the challenge of conducting systematic analysis methods with AI. Methods & Data Results Added Value Insights from the Hypersphere - Embedding Analytics in Market Research SPLENDID Research, Germany Relevance & Research Question: In the intersection of qualitative and quantitative research, analyzing open-ended questions remains a significant challenge for data analysts. The incorporation of AI language models introduces the complex embedding space: a realm where semantics intertwine with mathematical principles. This paper explores how Embedding Analytics, a subset of explainable AI, can be utilized to decode and analyze open-ended questions effectively. Methods & Data: Our approach utilized the ada_V2 encoder to transform market research responses into spatial representations on the surface of a 1,536-dimensional hypersphere. This process enabled us to analyze semantic similarities using traditional statistics as well as advanced machine learning techniques. We employed K-Means Clustering for text grouping and respondent segmentation, and Gaussian Mixture Models for overarching topic analysis across numerous responses. Dimensional reduction through t-SNE facilitated the transformation of these complex data sets into more comprehensible 2D or 3D visual representations. Results: Utilizing OpenAI’s ada_V2 encoder, we successfully generated text embeddings that can be plausibly clustered based on semantic content, transcending barriers of language and text length. These clusters, formed via K-Means and Gaussian Mixture Models, effectively yield insightful and automated analyses from qualitative data. The two-dimensional “cognitive constellations” created through t-SNE offer clear and accessible visualizations of intricate knowledge domains, such as brand perception or public opinion. Added Value: This methodology allows for a precise numerical analysis of verbatim responses without the need for labor-intensive manual coding. It facilitates automated segmentation, simplification of complex data, and even enables qualitative data to drive prediction tasks. The rich, nuanced datasets derived from semantic complexity are suitable for robust analysis using a wide range of statistical methods, thereby enhancing the efficacy and depth of market research analysis. |
2:00pm - 3:00pm | B6.2: AI Tools for Survey Research 2 Location: Seminar 4 (Room 1.11) Session Chair: Florian Keusch, University of Mannheim, Germany |
|
Vox Populi, Vox AI? Estimating German Public Opinion Through Language Models 1LMU Munich, Germany; 2University of Mannheim, Germany Relevance & Research Question: Integrating LLMs into cognitive pretesting procedures: A case study using ChatGPT GESIS - Leibniz Institute for the Social Sciences, Germany Relevance & Research Question Using Large Language Models for Evaluating and Improving Survey Questions 1University of Mannheim, Germany; 2LMU Munich, Germany Relevance & Research Question: The recent advances and availability of large language models (LLMs), such as OpenAI’s GPT, have created new opportunities for research in the social and behavioral sciences. Questionnaire development and evaluation is a potential area where researchers can benefit from LLMs: Trained on large amounts of text data, LLMs might serve as an easy-to-implement and inexpensive method for both assessing and improving the design of survey questions, by detecting problems in question wordings and suggesting alternative versions. In this paper, we examine to what extent GPT-4 can be leveraged for questionnaire design and evaluation by addressing the following research questions: (1) How accurately can GPT-4 detect problematic linguistic features in survey questions compared to existing computer-based evaluation methods? (2) To what extent can GPT-4 improve the design of survey questions? Methods & Data: We prompt GPT-4 with a set of survey questions and ask to identify features in the question stem or the response options that can potentially cause comprehension problems, such as vague terms or a complex syntax. For each survey question, we also ask the LLM to suggest an improved version. To compare the LLM-based results with an existing computer-based survey evaluation method, we use the Question Understanding Aid (QUAID; Graesser et al. 2006) that rates survey questions on different categories of comprehension problems. Based on an expert review among researchers with a PhD in survey methodology, we assess the accuracy of the GPT-4- and QUAID-based evaluation methods in identifying problematic features in the survey questions. We also ask the expert reviewers to evaluate the quality of the new question versions developed by GPT-4 compared to their original versions. Results: We compare both evaluation methods with regard to the number of problematic question features identified, building upon the five categories used in QUAID: (1) unfamiliar technical terms, (2) vague or imprecise relative terms, (3) vague or ambiguous noun phrases, (4) complex syntax, and (5) working memory overload. Added Value: The results from this paper provide novel evidence on the usefulness of LLMs for facilitating survey data collection. |
2:00pm - 3:00pm | D6: KI Forum: KI Café Location: Auditorium (Room 0.09/0.10/0.11) Session Moderators: Oliver Tabino, Q Agentur für Forschung Yannick Rieder, Janssen-Cilag GmbH Georg Wittenburg, Inspirient This session is in German. Moderierter Austausch zu folgenden Themen: • Messbare Qualität von KI-Tools ist Grundlage für Vertrauen und Voraussetzung für den betrieblichen Einsatz, aber welche Qualitätskriterien haben sich bewährt? Wie können sie erfasst und verglichen werden? • Wie implementiert man KI-Anwendungen in Prozesse? Wobei ist die Nutzung bereits etabliert? Was gibt es dabei zu beachten? • KI und Ethik: Was geht und was nicht? |
3:00pm - 3:15pm | Break |
3:15pm - 4:15pm | A7.1: Survey Methods Interventions 2 Location: Seminar 1 (Room 1.01) Session Chair: Joss Roßmann, GESIS - Leibniz Institute for the Social Sciences, Germany |
|
Pushing older target persons to the web: Do we still need a paper questionnaire? GESIS - Leibniz-Institut für Sozialwissenschaften, Germany Relevance & Research Question Methods & Data Results Added Value Clarification features in web surveys: Usage and impact of “on-demand” instructions GESIS - Leibniz Institute for the Social Sciences, Germany Relevance & Research Question |
3:15pm - 4:15pm | A7.2: Social Media Recruited Surveys Location: Seminar 3 (Room 1.03/1.04) Session Chair: Tobias Rettig, University of Mannheim, Germany |
|
Assessing the impact of advertisement design on response quality in surveys using social media recruitment 1Max Planck Institut for Demographic Research, Germany; 2Bielefeld University, Germany Relevance & research question: Researchers are increasingly using social media platforms for survey recruitment. Typically, advertisements are distributed through these platforms to motivate users to participate in an online survey. To date, there is little empirical evidence on how the content and design characteristics of advertisements can affect response quality in surveys based on social media recruitment. This project is the first comprehensive study of the effects of ad design on response quality in surveys recruited via social media. Methods and data: We use data from the SoMeRec survey, which was conducted via Facebook ads in Germany and the United States in June 2023 and focused primarily on climate change and migration. The survey ad campaign featured 15 images with different thematic associations to climate change and migration, including strong and loose associations and neutral images. A commercial access panel company was contracted to include identical survey questions serving as benchmark comparison. The Facebook sample consisted of 7,139 respondents in Germany and 13,022 in the US, while the access panel consisted of 1,555 surveys in Germany and 1,576 surveys in the US. In our analyses, we compare common data quality indicators, including completion time, straightlining, item non-response, and follow-up availability, across different ad features. Results: First analysis show that survey completion time is higher for thematic ad designs compared to neutral ads and the reference sample. There are differences in the overall item non-response rate, with higher item non-response for the immigration-themed ad designs. There are no significant differences in straightlining between samples and ad designs. Finally, respondents recruited through neutral ads were more likely to be available for follow up surveys than those recruited through themed ads. Added value: Our study advances the literature by studying the general population in Germany and the US, by testing various indicators of survey data quality, and by including a benchmark survey of respondents not recruited through social media. The results clearly indicate an effect of ad design on survey data quality and highlight the importance of sample and recruitment design for estimates based on social media recruitment and online surveys. Do expensive social media ad groups pay off in the recruitment of a non-probabilistic panel? An inspection on coverage and cost structure GESIS Leibniz Institute for the Social Sciences, Germany Relevance & Research Question: Social media advertisement is becoming an increasingly popular method of recruiting participants for studies in the social sciences. Recently, more and more participants of surveys are recruited via social media. This method of recruitment has been particularly prominent for recruiting special populations for surveys, such as migrants or LGBT persons, but recently meta has significantly reduced these selection criteria. However, meta still allows the selection of common socio-demographic characteristics, such as age and gender, when placing an ad. Meta estimates these socio-demographic characteristics based on the user's data. With this information, we took an non-probabilistic quota-sampling-like approach by specifying to meta the desired peoples' proportions for socio-demographic characteristics which should click on the ad and be directed to the recruitment survey of our nonprobabilistic panel. However, the volatile and hard to control nature of social media recruitment opens it up to scrutiny and demands evaluation. In this study we assess coverage issues and cost effectiveness of utilizing Meta advertisement in recruiting respondents for a non-probabilistic online panel, we consider three aspects in detail. First, we evaluate the extent to which the targeting criteria, namely age and gender achieve a balanced sample at different stages of the registration process into the panel and give recommendations for adjustments. Furthermore, we validate whether these social media targeting criteria are reliable and agree with the survey answers. Third, we assess the cost structure in the light of the response propensities at the different stages of the recruitment process and investigate whether expensive social media ad groups pay off in the long-term. Methods & Data: We are using data from the recruiment of the new GESIS Panel Plus. The recuitmenr process includes several steps and we sill consider each step individually using multivariate analysis methods. Results: First results suggest that expensive recruitment groups do not pay off in the long term. Added Value: These research will open up the black box of cost structure in relation to socio - demographic attributes when using Meta as recruitment frame for cross-sectional and longitudinal surveys. |
3:15pm - 4:15pm | B7: Mobile Apps and Sensors Location: Seminar 2 (Room 1.02) Session Chair: Ramona Schoedel, Charlotte Fresenius Hochschule, University of Psychology, Germany |
|
Mechanisms of Participation in Smartphone App Data Collection: A Research Synthesis University of Mannheim Relevance & Research Question: Smartphone app data collection has recently gained increasing attention in the social and behavioral sciences, allowing researchers to integrate surveys with sensor data, such as GPS to measure location and movement. Similar to other forms of surveys, participation rates of such studies in general population samples are generally low. Previous research has identified several study- and participant-level determinants of willingness to participate in smartphone app data collection. However, a comprehensive overview of which factors are predictors of willingness and a theoretical framework are currently lacking and some of the effects are inconsistent. To guide future app-based studies, we address the following research questions: (1) Which study- and participant-level characteristics affect the willingness to participate in smartphone app data collection? (2) Which theoretical frameworks can be used to understand participation decisions in smartphone app data collection? Methods & Data: We conduct a systemic review and a meta-analysis on existing studies with app-based data collection guided by the Preferred Reporting Items for Systematic reviews and Meta-analysis (PRISMA) framework (Moher et al. 2009). We compile a list of keywords to search for relevant literature in bibliographic databases. We focus on peer-reviewed articles published in English. We also perform double coding to ensure a reliable selection of literature for the analysis. Finally, we map the identified determinants of willingness to potential theoretical frameworks that can explain participation behavior. Results: In the systematic review, we summarize findings about study-level characteristics that are under the researchers' control, such as monetary incentives or invitation mode, and participant-level characteristics, such as privacy concerns and socio-demographics. Meanwhile, the meta-analysis focuses on selected characteristics, which have been most often covered in previous research. Added Value: This study will provide a holistic understanding of the current state of research on participation decisions in app-based studies. The findings will also help researchers to design effective invitation strategies for future studies. “The value of privacy is not as high as finding my person”: Self-disclosure practices on dating apps illustrate an existential dilemma for data protection University of Oxford, United Kingdom Relevance & Research Question: Dating apps create a unique digital sphere where people must disclose sensitive personal information about their demographics, location, values and lifestyle. Because of these intimate disclosures, dating apps constitute a strategic research site to explore how privacy concerns influence personal information disclosure. We use construal-level theory to understand how context influences a decision to disclose. Construal-level theory refers to the influence of psychological distance: the more psychologically distant an event the more mental effort required to understand it. When people have no direct experience in a context they rely on conventional stereotypes and quick generalizations. Using this theory we ask the research question: Why do people choose to disclose or not disclose personal Information on their dating app profile? Money or Motivation? Decision Criteria to participate in Smart Surveys Destatis - Federal Statistical Office Germany, Germany Relevance & Research Question The German Federal Statistical Office (Destatis) is continuing to develop its data collection instruments and is working on smart surveys in this context. By smart surveys we mean the combination of traditional question-based survey data collection and digital trace data collection by accessing device sensor data via an application (GPS, camera, microphone, accelerometer, ...). Unlike traditional surveys, smart surveys not only ask respondents for information but also require them to download an app and allow access to sensor data. Destatis conducted focus groups to learn more about the attitudes, motives and obstacles regarding the willingness to participate in smart surveys. This was done as part of the European Union's Smart Survey Implementation (SSI) project, in which Destatis is participating alongside other project partners. Methods & Data Three focus groups with a total of 16 participants were conducted at the end of October 2023. The group discussions were led by a moderator using a guideline. The discussions lasted around two hours each and were video-recorded. Results Overall, it became clear that participants are more willing to take part in a survey, to download an app and to grant access to sensor data if they see a purpose in doing so on the one hand and have trust on the other. In order to motivate people to participate, it seems particularly important against this background to provide transparent information explaining why to conduct the survey, why they should participate, why access to the sensor data is desired as well as what is being done to ensure a high level of data protection and data security. Added Value In official statistics, the development of new survey methods is seen as an important step towards modern data collection. However, modern survey methods can only make a positive contribution if they are used by respondents. The results are intended to provide information on how potential respondents can best be addressed to participate. In the further course of the SSI project, a quantitative field test for recruitment is planned. The results of the focus groups will also be used to prepare this test. |
Contact and Legal Notice · Contact Address: Privacy Statement · Conference: GOR 24 |
Conference Software: ConfTool Pro 2.8.102 © 2001–2024 by Dr. H. Weinreich, Hamburg, Germany |